A Multi-View Convolutional Neural Network Method Combining Attention Mechanism for Diagnosing Autism Spectrum Disorder
PLOS ONE(2023)
摘要
Autism Spectrum Disorder (ASD) is a neurodevelopmental condition whose current psychiatric diagnostic process is subjective and behavior-based. In contrast, functional magnetic resonance imaging (fMRI) can objectively measure brain activity and is useful for identifying brain disorders. However, the ASD diagnostic models employed to date have not reached satisfactory levels of accuracy. This study proposes the use of MAACNN, a method that utilizes multi-view convolutional neural networks (CNNs) in conjunction with attention mechanisms for identifying ASD in multi-scale fMRI. The proposed algorithm effectively combines unsupervised and supervised learning. In the initial stage, we employ stacked denoising autoencoders, an unsupervised learning method for feature extraction, which provides different nodes to adapt to multi-scale data. In the subsequent stage, we perform supervised learning by employing multi-view CNNs for classification and obtain the final results. Finally, multi-scale data fusion is achieved by using the attention fusion mechanism. The ABIDE dataset is used to evaluate the model we proposed., and the experimental results show that MAACNN achieves superior performance with 75.12% accuracy and 0.79 AUC on ABIDE-I, and 72.88% accuracy and 0.76 AUC on ABIDE-II. The proposed method significantly contributes to the clinical diagnosis of ASD.
更多查看译文
关键词
Autism,Brain Network Development,Neuroimaging Data Analysis
AI 理解论文
溯源树
样例
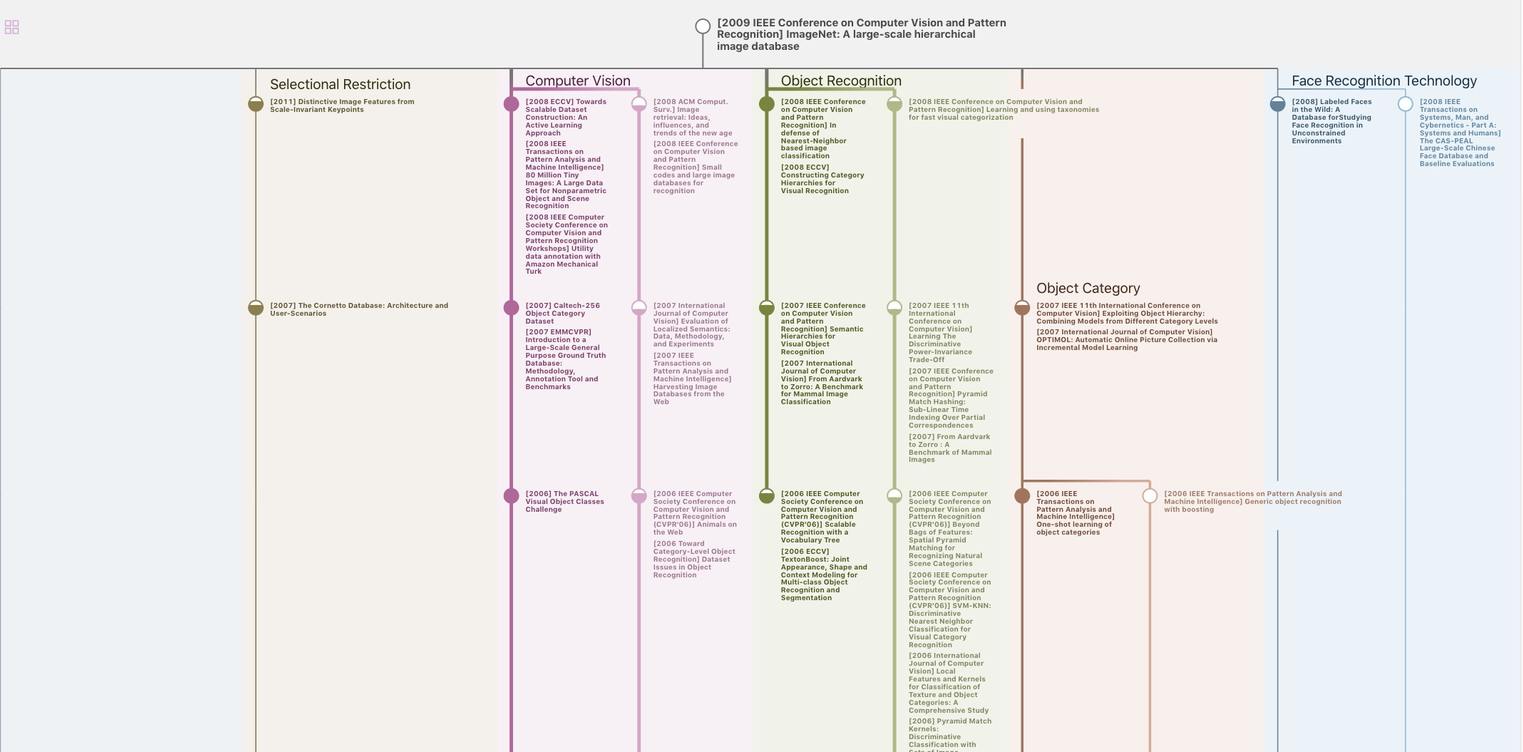
生成溯源树,研究论文发展脉络
Chat Paper
正在生成论文摘要