Open-Domain Question Answering Goes Conversational via Question Rewriting
arXiv (Cornell University)(2020)
摘要
We introduce a new dataset for Question Rewriting in Conversational Context (QReCC), which contains 14K conversations with 80K question-answer pairs. The task in QReCC is to find answers to conversational questions within a collection of 10M web pages (split into 54M passages). Answers to questions in the same conversation may be distributed across several web pages. QReCC provides annotations that allow us to train and evaluate individual subtasks of question rewriting, passage retrieval and reading comprehension required for the end-to-end conversational question answering (QA) task. We report the effectiveness of a strong baseline approach that combines the state-of-the-art model for question rewriting, and competitive models for open-domain QA. Our results set the first baseline for the QReCC dataset with F1 of 19.10, compared to the human upper bound of 75.45, indicating the difficulty of the setup and a large room for improvement.
更多查看译文
关键词
conversational,open-domain
AI 理解论文
溯源树
样例
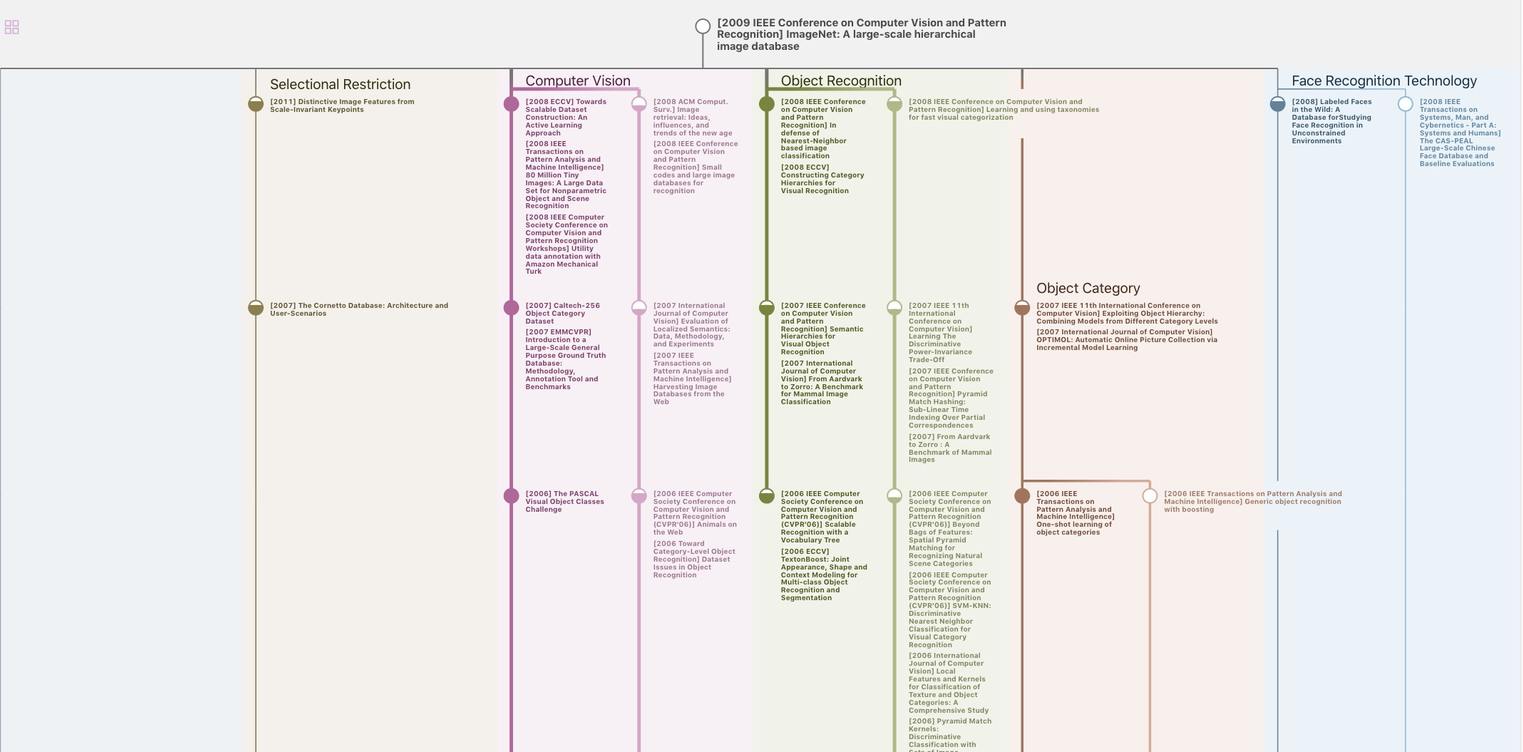
生成溯源树,研究论文发展脉络
Chat Paper
正在生成论文摘要