Symmetry & critical points for a model shallow neural network
arXiv (Cornell University)(2020)
摘要
We consider the optimization problem associated with fitting two-layer ReLU networks with $k$ hidden neurons, where labels are assumed to be generated by a (teacher) neural network. We leverage the rich symmetry exhibited by such models to identify various families of critical points and express them as power series in $k^{-\frac{1}{2}}$. These expressions are then used to derive estimates for several related quantities which imply that not all spurious minima are alike. In particular, we show that while the loss function at certain types of spurious minima decays to zero like $k^{-1}$, in other cases the loss converges to a strictly positive constant. The methods used depend on symmetry, the geometry of group actions, bifurcation, and Artin's implicit function theorem.
更多查看译文
关键词
symmetry,neural network,critical points,model
AI 理解论文
溯源树
样例
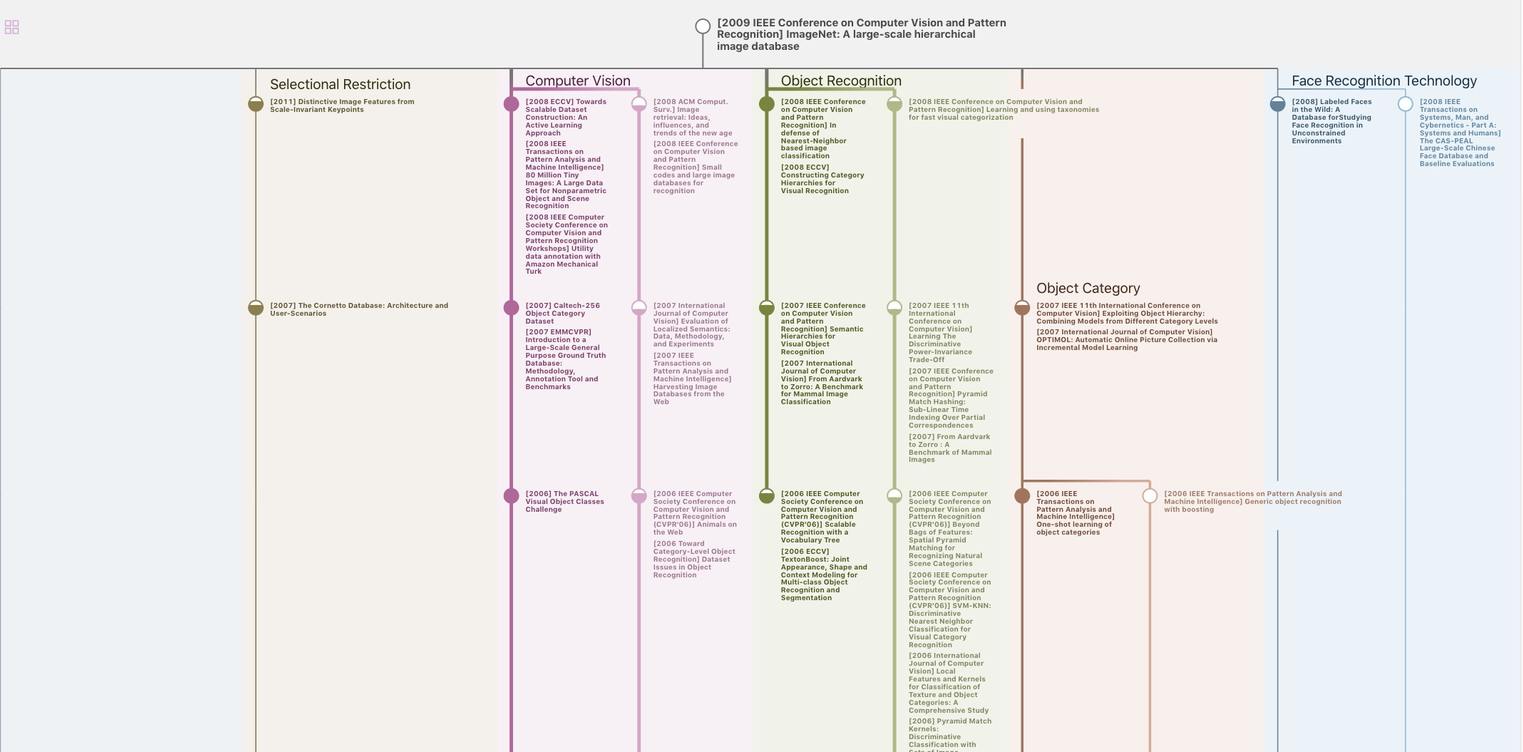
生成溯源树,研究论文发展脉络
Chat Paper
正在生成论文摘要