Zeroth-order methods for noisy H\"older-gradient functions
arXiv (Cornell University)(2020)
摘要
In this paper, we prove new complexity bounds for zeroth-order methods in non-convex optimization with inexact observations of the objective function values. We use the Gaussian smoothing approach of Nesterov and Spokoiny [2015] and extend their results, obtained for optimization methods for smooth zeroth-order non-convex problems, to the setting of minimization of functions with H\"older-continuous gradient with noisy zeroth-order oracle, obtaining noise upper-bounds as well. We consider finite-difference gradient approximation based on normally distributed random Gaussian vectors and prove that gradient descent scheme based on this approximation converges to the stationary point of the smoothed function. We also consider convergence to the stationary point of the original (not smoothed) function and obtain bounds on the number of steps of the algorithm for making the norm of its gradient small. Additionally, we provide bounds for the level of noise in the zeroth-order oracle for which it is still possible to guarantee that the above bounds hold. We also consider separately the case of $\nu = 1$ and show that in this case the dependence of the obtained bounds on the dimension can be improved.
更多查看译文
关键词
zeroth-order,older-gradient
AI 理解论文
溯源树
样例
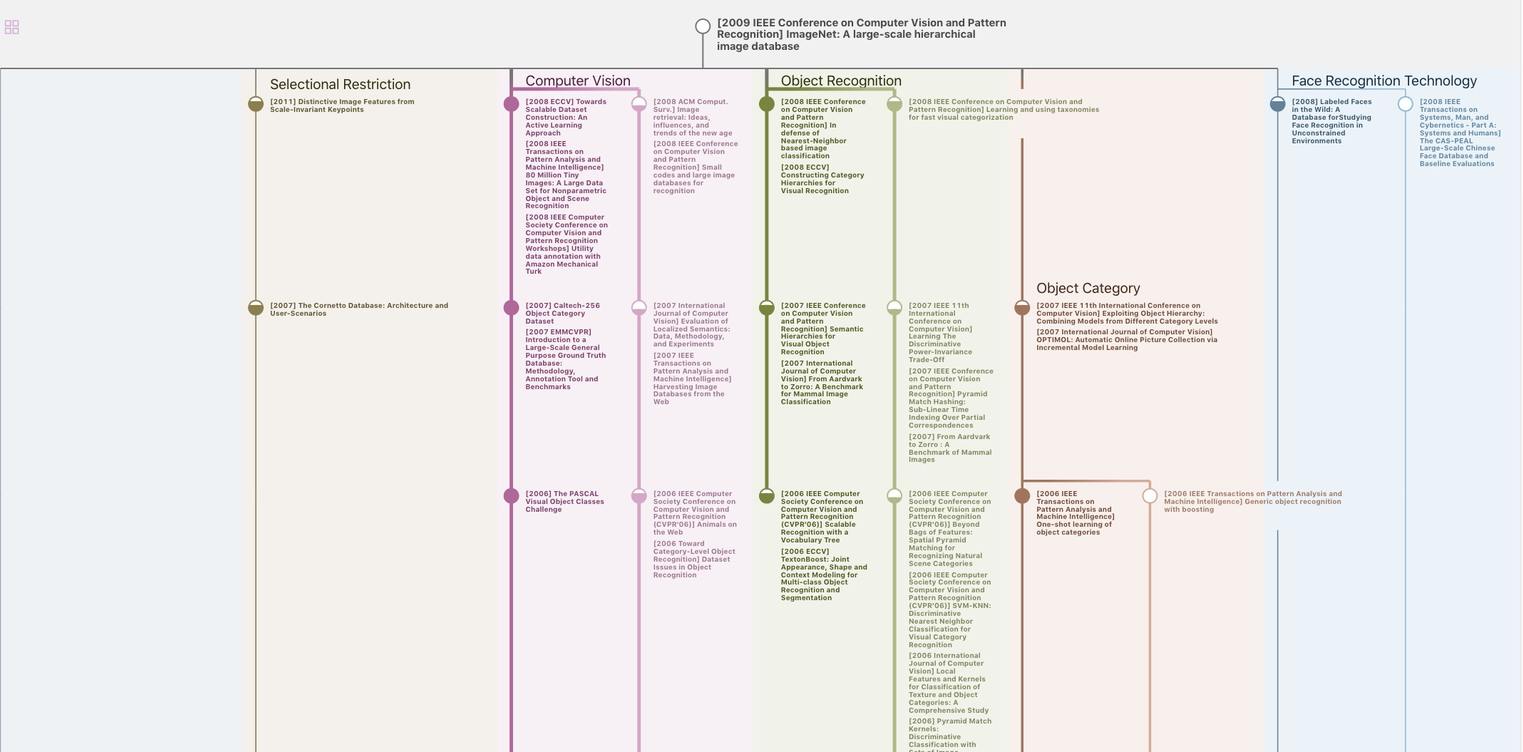
生成溯源树,研究论文发展脉络
Chat Paper
正在生成论文摘要