Exploiting Non-Linear Redundancy for Neural Model Compression
arXiv (Cornell University)(2020)
摘要
Deploying deep learning models, comprising of non-linear combination of millions, even billions, of parameters is challenging given the memory, power and compute constraints of the real world. This situation has led to research into model compression techniques most of which rely on suboptimal heuristics and do not consider the parameter redundancies due to linear dependence between neuron activations in overparametrized networks. In this paper, we propose a novel model compression approach based on exploitation of linear dependence, that compresses networks by elimination of entire neurons and redistribution of their activations over other neurons in a manner that is provably lossless while training. We combine this approach with an annealing algorithm that may be applied during training, or even on a trained model, and demonstrate, using popular datasets, that our method results in a reduction of up to 99\% in overall network size with small loss in performance. Furthermore, we provide theoretical results showing that in overparametrized, locally linear (ReLU) neural networks where redundant features exist, and with correct hyperparameter selection, our method is indeed able to capture and suppress those dependencies.
更多查看译文
关键词
neural,model,non-linear
AI 理解论文
溯源树
样例
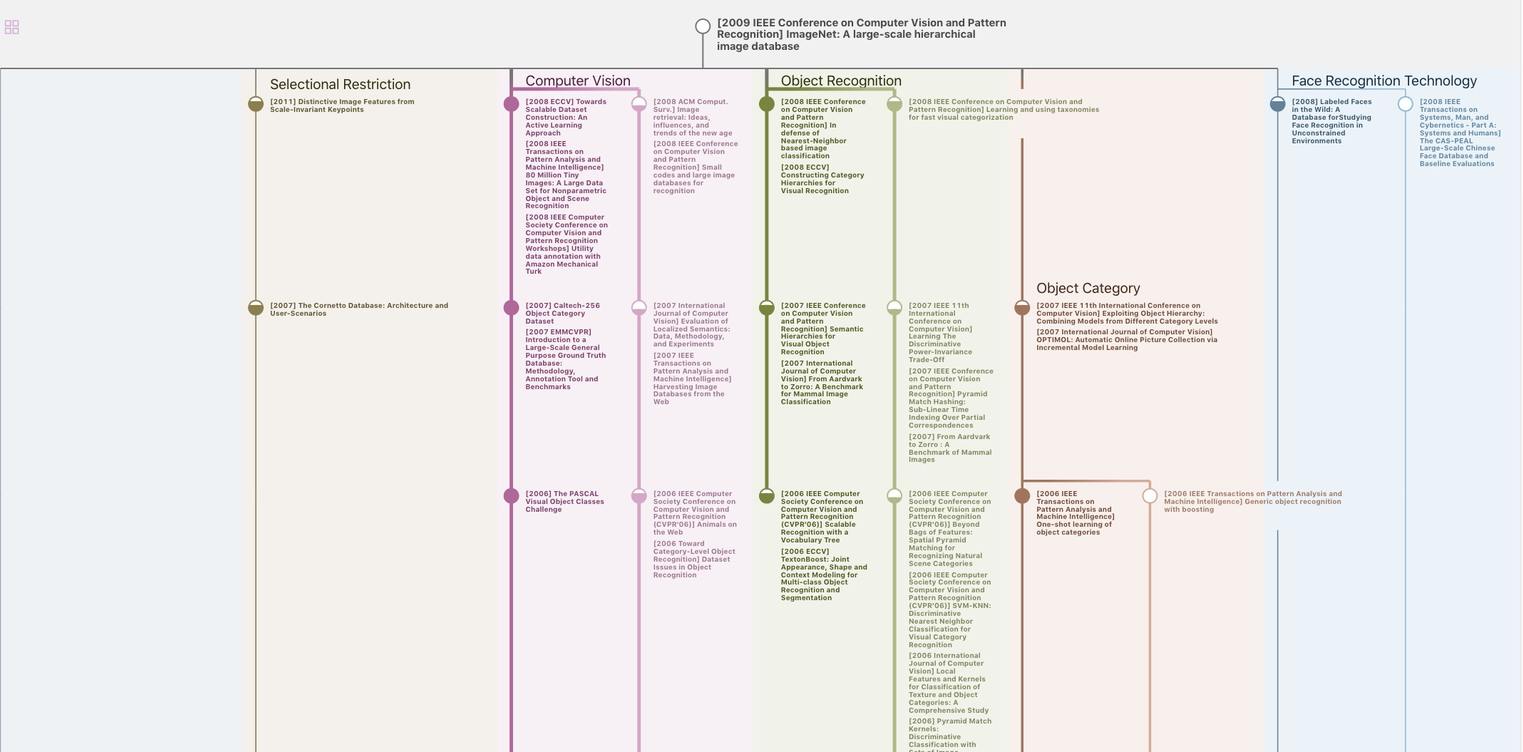
生成溯源树,研究论文发展脉络
Chat Paper
正在生成论文摘要