Detecting and analyzing collusive entities on YouTube
arXiv (Cornell University)(2020)
摘要
In this work, we provide an in-depth analysis of collusive entities on YouTube fostered by various blackmarket services. Following this, we propose models to detect three types of collusive YouTube entities - videos seeking collusive likes, channels seeking collusive subscriptions, and videos seeking collusive comments. The third type of entity is associated with temporal information. To detect videos and channels for collusive likes and subscriptions respectively, we utilize one-class classifiers trained on our curated collusive entities and a set of novel features. The SVM-based model shows significant performance with a true positive rate of 0.911 and 0.910 for detecting collusive videos and collusive channels respectively. To detect videos seeking collusive comments, we propose CollATe, a novel end-to-end neural architecture that leverages time-series information of posted comments along with static metadata of videos. CollATe is composed of three components - metadata feature extractor (which derives metadata-based features from videos), anomaly feature extractor (which utilizes the comment time-series data to detect sudden changes in the commenting activity), and comment feature extractor (which utilizes the text of the comments posted during collusion and computes a similarity score between the comments). Extensive experiments show the effectiveness of CollATe (with a true positive rate of 0.905) over the baselines.
更多查看译文
关键词
collusive entities,youtube
AI 理解论文
溯源树
样例
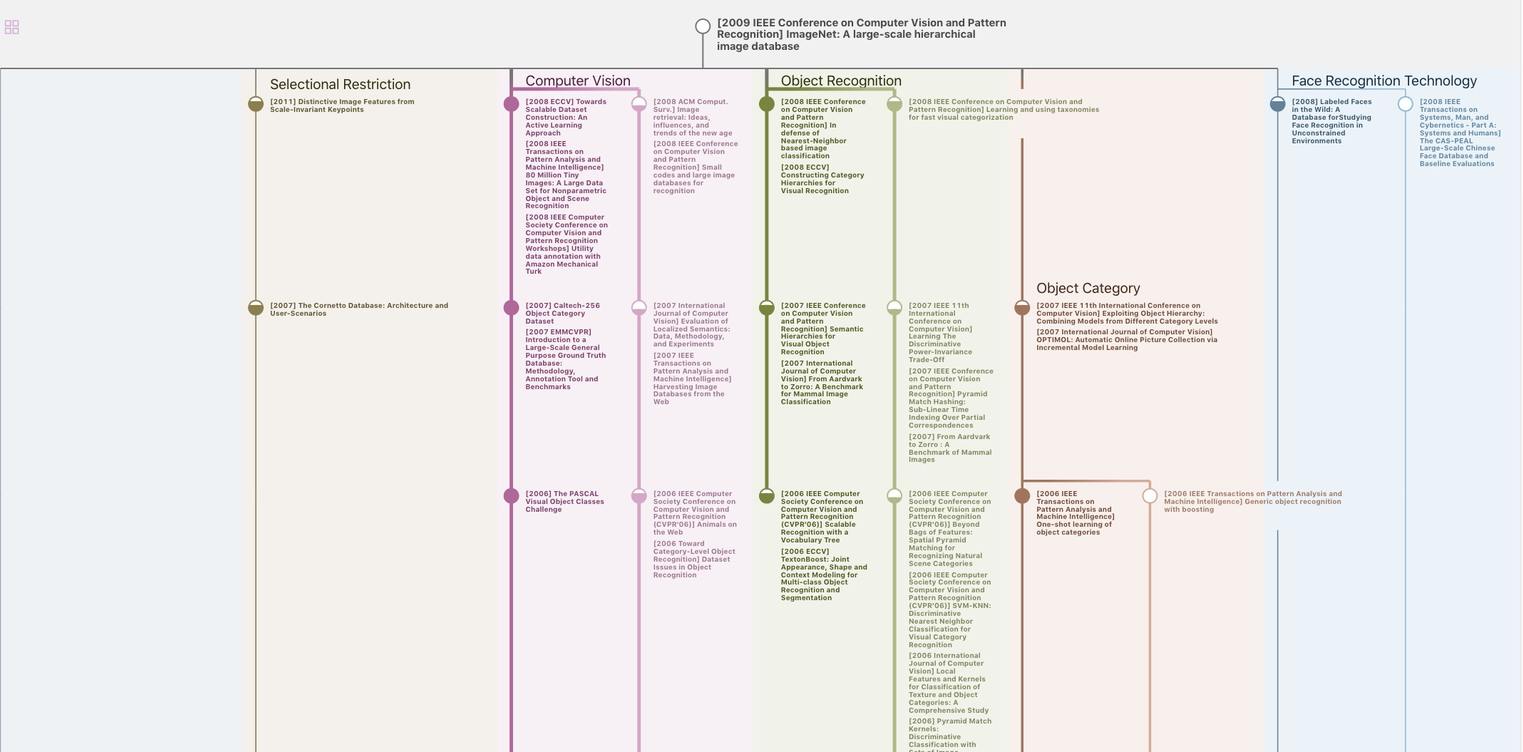
生成溯源树,研究论文发展脉络
Chat Paper
正在生成论文摘要