kNN-CM: A Non-parametric Inference-Phase Adaptation of Parametric Text Classifiers.
EMNLP 2023(2023)
摘要
Semi-parametric models exhibit the properties of both parametric and non-parametric modeling and have been shown to be effective in the next-word prediction language modeling task. However, there is a lack of studies on the text-discriminating properties of such models. We propose an inference-phase approach---\textit{k}-Nearest Neighbor Classification Model (\textit{k}NN-CM)---that enhances the capacity of a pre-trained parametric text classifier by incorporating a simple neighborhood search through the representation space of (memorized) training samples. The final class prediction of \textit{k}NN-CM is based on the convex combination of probabilities obtained from \textit{k}NN search and prediction of the classifier. Our experiments show consistent performance improvements on eight SuperGLUE tasks, three adversarial natural language inference (ANLI) datasets, 11 question-answering (QA) datasets, and two sentiment classification datasets.
更多查看译文
AI 理解论文
溯源树
样例
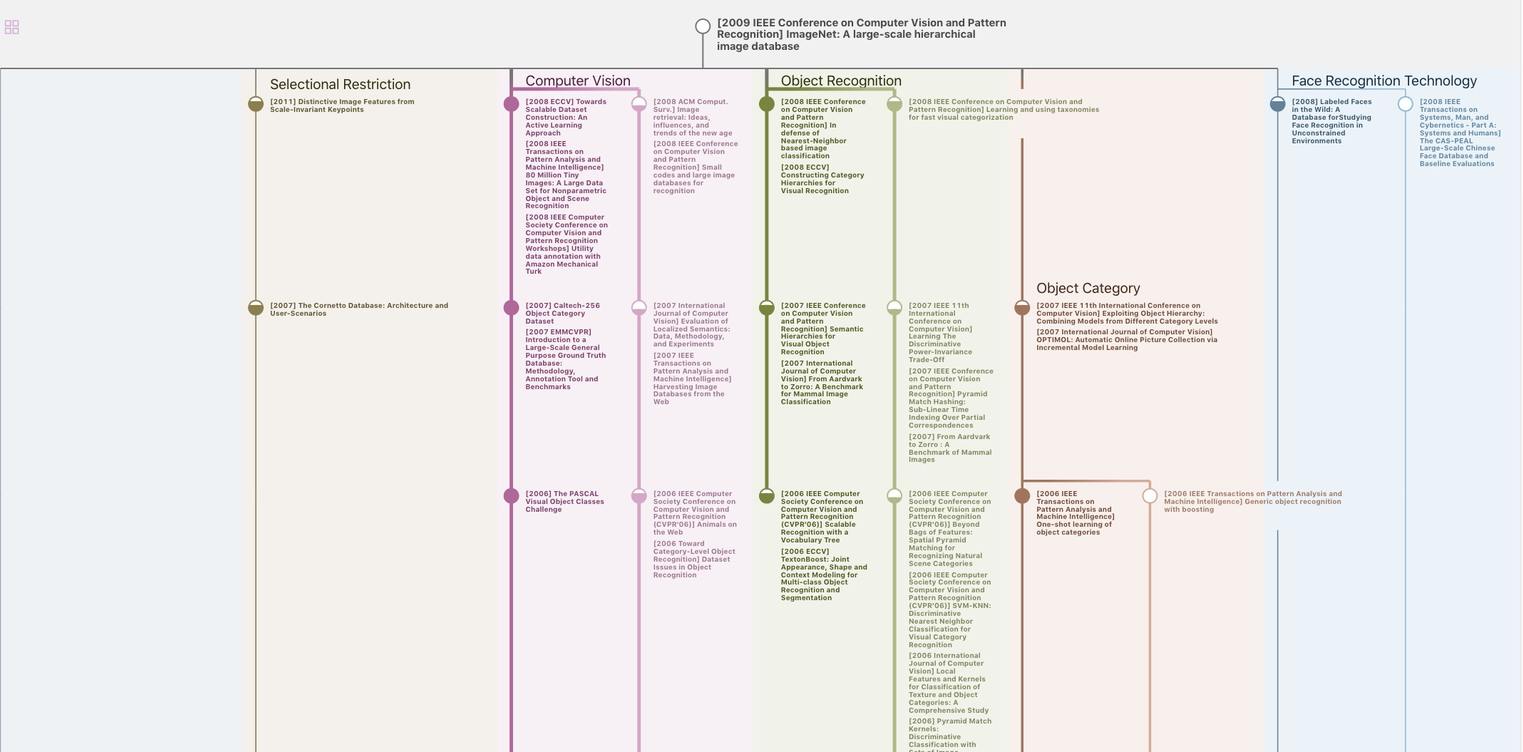
生成溯源树,研究论文发展脉络
Chat Paper
正在生成论文摘要