Let's Think Frame by Frame with VIP: A Video Infilling and Prediction Dataset for Evaluating Video Chain-of-Thought.
EMNLP 2023(2023)
摘要
Despite exciting recent results showing vision-language systems’ capacity to reason about images using natural language, their capacity for video reasoning remains underexplored. We motivate framing video reasoning as the sequential understanding of a small number of keyframes, thereby leveraging the power and robustness of vision-language while alleviating the computational complexities of processing videos. To evaluate this novel application, we introduce VIP, an inference-time challenge dataset designed to explore models’ reasoning capabilities through video chain-of-thought. Inspired by visually descriptive scene plays, we propose two formats for keyframe description: unstructured dense captions and structured scene descriptions that identify the focus, action, mood, objects, and setting (FAMOuS) of the keyframe. To evaluate video reasoning, we propose two tasks: Video Infilling and Video Prediction, which test abilities to generate multiple intermediate keyframes and predict future keyframes, respectively. We benchmark GPT-4, GPT-3, and VICUNA on VIP, demonstrate the performance gap in these complex video reasoning tasks, and encourage future work to prioritize language models for efficient and generalized video reasoning.
更多查看译文
关键词
evaluating video chain,infilling,frame,prediction
AI 理解论文
溯源树
样例
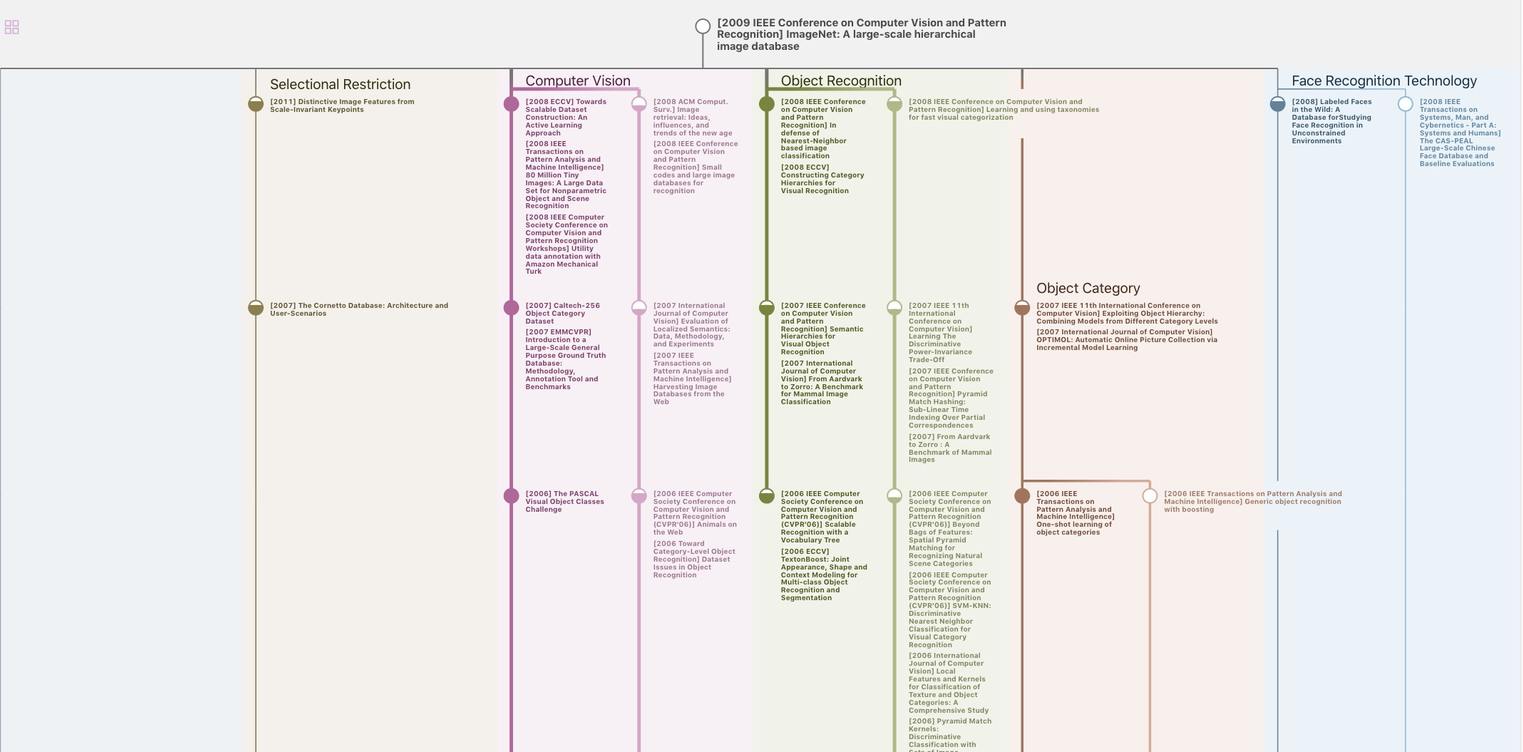
生成溯源树,研究论文发展脉络
Chat Paper
正在生成论文摘要