What is the Best Grid-Map for Self-Driving Cars Localization? An Evaluation under Diverse Types of Illumination, Traffic, and Environment
arXiv (Cornell University)(2020)
摘要
The localization of self-driving cars is needed for several tasks such as keeping maps updated, tracking objects, and planning. Localization algorithms often take advantage of maps for estimating the car pose. Since maintaining and using several maps is computationally expensive, it is important to analyze which type of map is more adequate for each application. In this work, we provide data for such analysis by comparing the accuracy of a particle filter localization when using occupancy, reflectivity, color, or semantic grid maps. To the best of our knowledge, such evaluation is missing in the literature. For building semantic and colour grid maps, point clouds from a Light Detection and Ranging (LiDAR) sensor are fused with images captured by a front-facing camera. Semantic information is extracted from images with a deep neural network. Experiments are performed in varied environments, under diverse conditions of illumination and traffic. Results show that occupancy grid maps lead to more accurate localization, followed by reflectivity grid maps. In most scenarios, the localization with semantic grid maps kept the position tracking without catastrophic losses, but with errors from 2 to 3 times bigger than the previous. Colour grid maps led to inaccurate and unstable localization even using a robust metric, the entropy correlation coefficient, for comparing online data and the map.
更多查看译文
关键词
localization,grid-map,self-driving
AI 理解论文
溯源树
样例
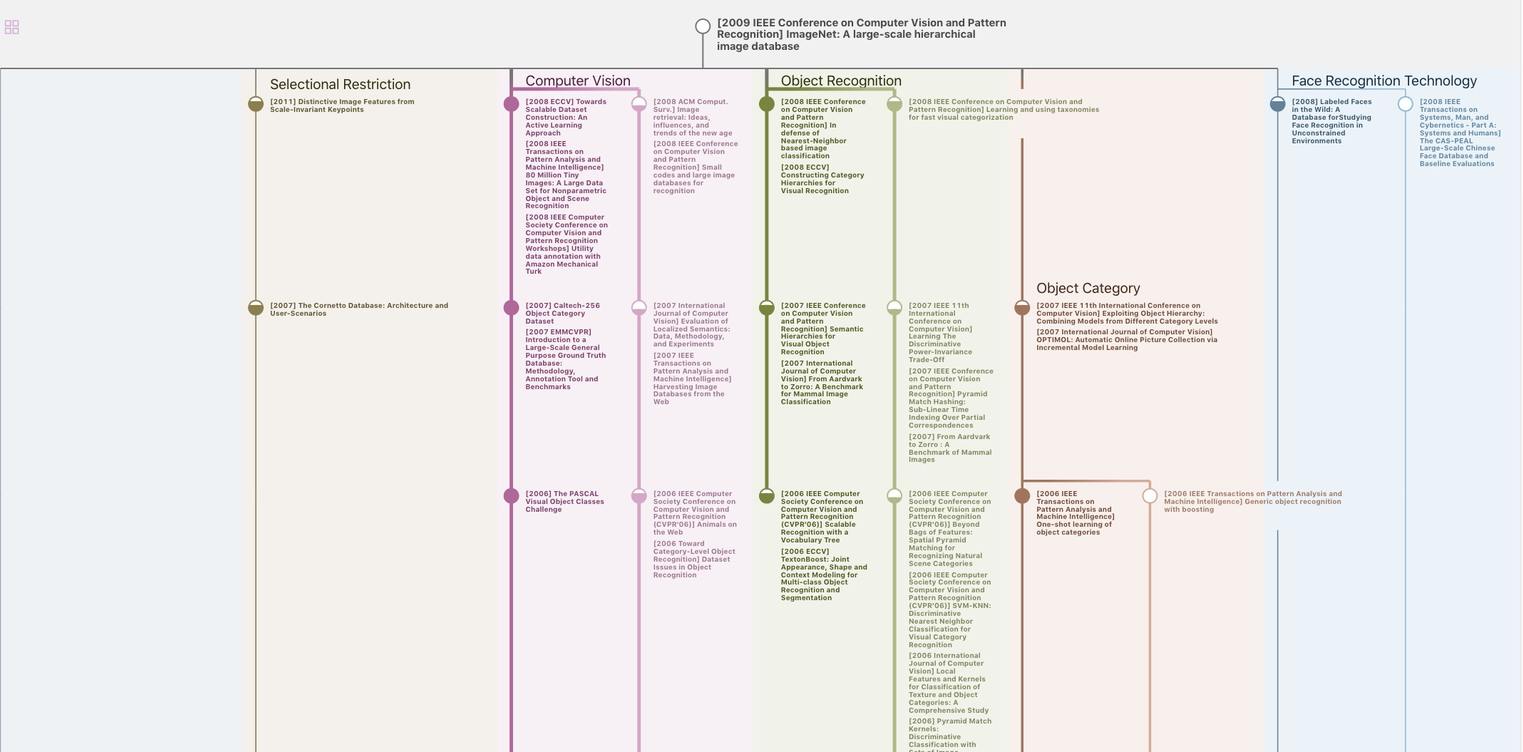
生成溯源树,研究论文发展脉络
Chat Paper
正在生成论文摘要