Noise-Robust Semi-Supervised Learning for Distantly Supervised Relation Extraction.
EMNLP 2023(2023)
摘要
Distantly supervised relation extraction (DSRE) aims to extract relational facts from texts but suffers from noisy instances.
To mitigate the influence of noisy labels, current methods typically use the Multi-Instance-Learning framework to extract relations for each bag. However, these approaches are not capable of extracting relation labels for individual sentences. Several studies have focused on sentence-level DSRE to solve the above problem. These studies primarily aim to develop methods for identifying noisy samples and filtering them out to mitigate the impact of noise. However, discarding noisy samples directly leads to the loss of useful information. To this end, we propose SSLRE, a novel Semi-Supervised-Learning Relation Extraction framework for sentence-level DSRE. We discard only the labels of the noisy samples and utilize these instances without labels as unlabeled samples. Our SSLRE framework utilizes a weighted K-NN graph to select confident samples as labeled data and the rest as unlabeled. We then design a robust semi-supervised learning framework that can efficiently handle remaining label noise present in the labeled dataset, while also making effective use of unlabeled samples. Based on our experiments on two real-world datasets, the SSLRE framework we proposed has achieved significant enhancements in sentence-level relation extraction performance compared to the existing state-of-the-art methods. Moreover, it has also attained a state-of-the-art level of performance in bag-level relation extraction with ONE aggregation strategy.
更多查看译文
AI 理解论文
溯源树
样例
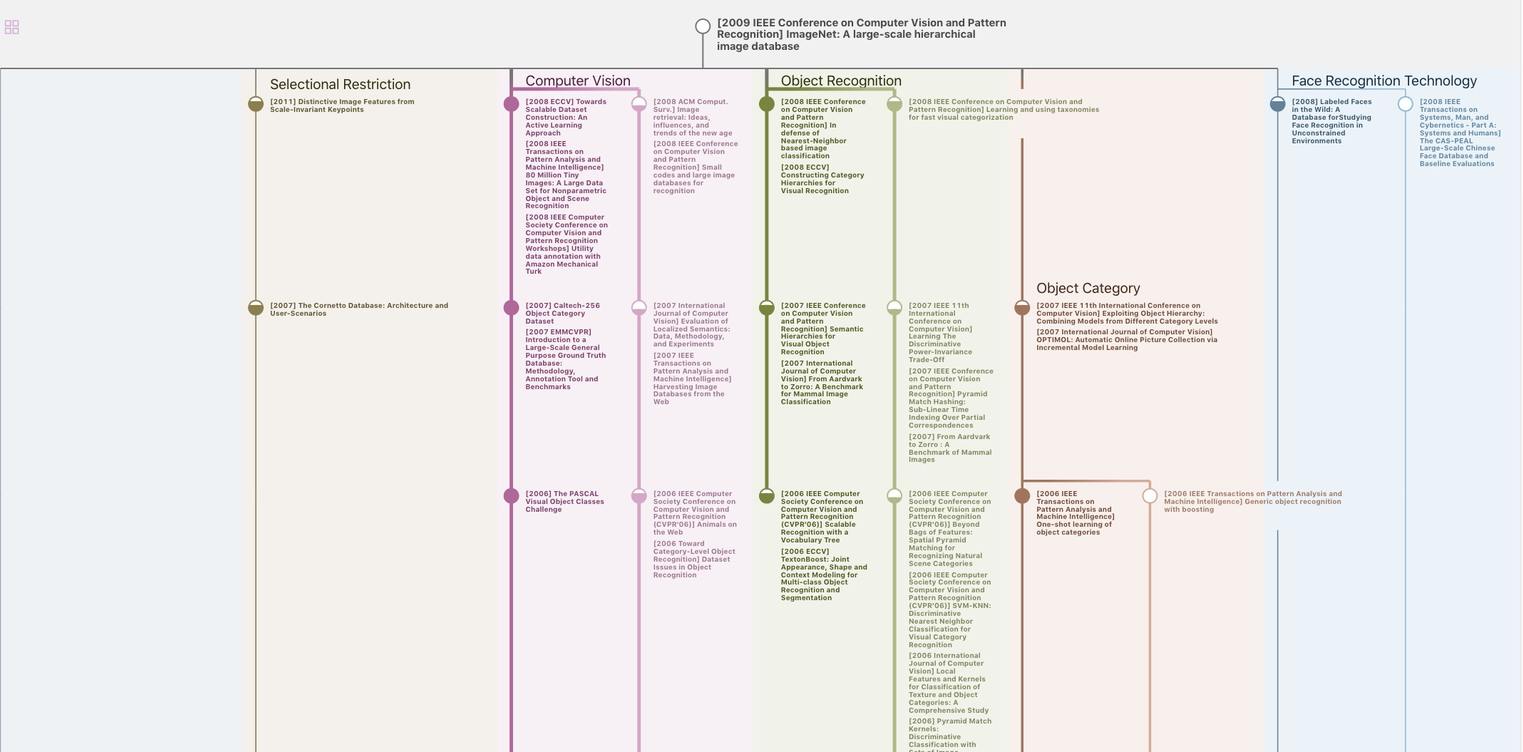
生成溯源树,研究论文发展脉络
Chat Paper
正在生成论文摘要