Modeling Interference for Individual Treatment Effect Estimation from Networked Observational Data
ACM TRANSACTIONS ON KNOWLEDGE DISCOVERY FROM DATA(2024)
摘要
Estimating individual treatment effect (ITE) from observational data has attracted great interest in recent years, which plays a crucial role in decision-making across many high-impact domains such as economics, medicine, and e-commerce. Most existing studies of ITE estimation assume that different units at play are independent and do not influence each other. However, many social science experiments have shown that there often exist different levels of interactions between units in observational data, especially in a networked environment. As a result, the treatment assignment of one unit can affect the outcome of other units connected to it in the network, which is referred to as the interference or spillover effect. In this article, we study an important problem of ITE estimation from networked observational data by modeling the interference between different units and provide a principled framework to support such study. Methodologically, we propose a novel framework, SPNet, that first captures the influence of hidden confounders with the aid of graph convolutional network and then models the interference by introducing an environment summary variable and developing a masked attention mechanism. Experimental evaluations on several semi-synthetic datasets based on real-world networks corroborate the superiority of our proposed framework over state-of-the-art individual treatment effect estimation methods.
更多查看译文
关键词
Causal inference,ITE estimation,network interference
AI 理解论文
溯源树
样例
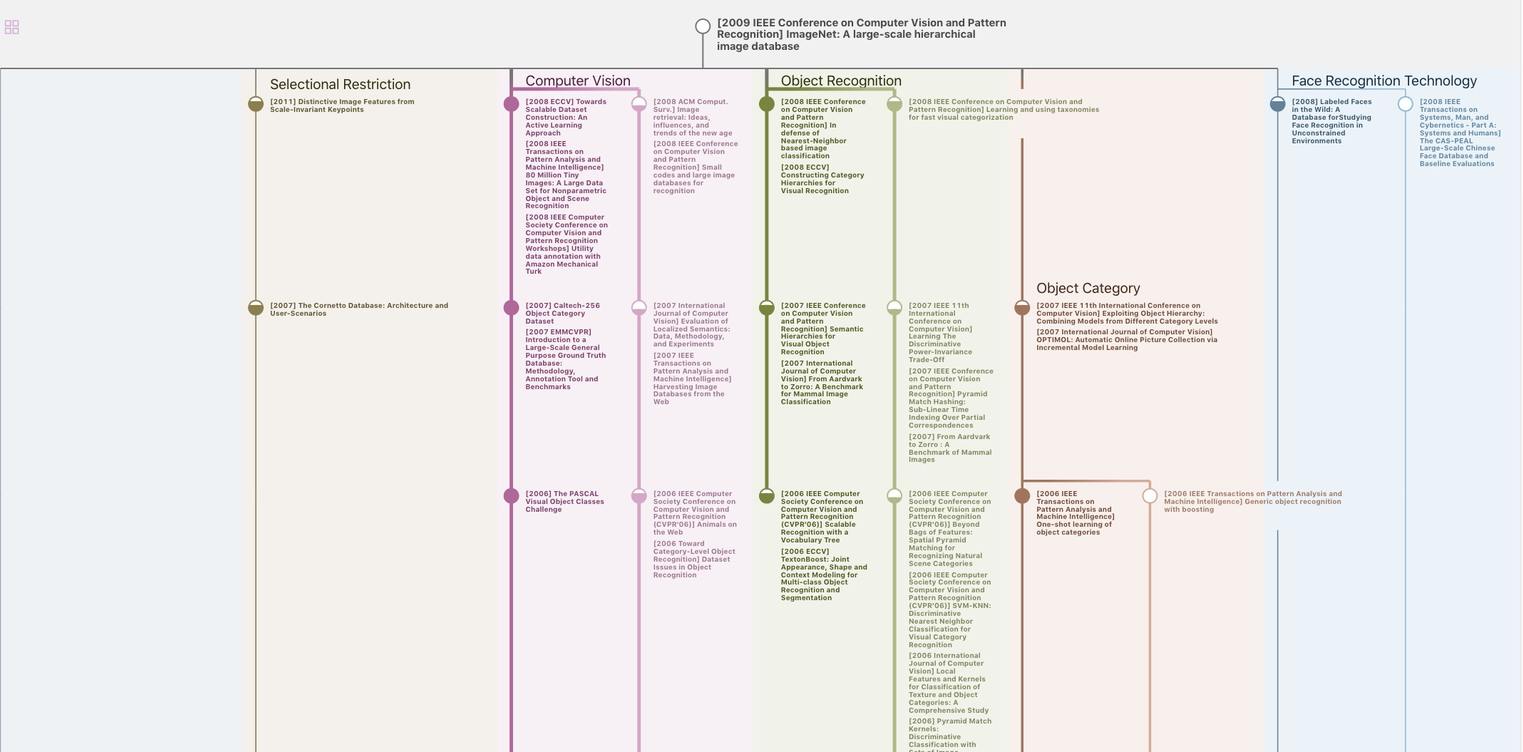
生成溯源树,研究论文发展脉络
Chat Paper
正在生成论文摘要