Voting-based Approaches For Differentially Private Federated Learning
arXiv (Cornell University)(2020)
摘要
Differentially Private Federated Learning (DPFL) is an emerging field with many applications. Gradient averaging based DPFL methods require costly communication rounds and hardly work with large-capacity models, due to the explicit dimension dependence in its added noise. In this work, inspired by knowledge transfer non-federated privacy learning from Papernot et al.(2017; 2018), we design two new DPFL schemes, by voting among the data labels returned from each local model, instead of averaging the gradients, which avoids the dimension dependence and significantly reduces the communication cost. Theoretically, by applying secure multi-party computation, we could exponentially amplify the (data-dependent) privacy guarantees when the margin of the voting scores are large. Extensive experiments show that our approaches significantly improve the privacy-utility trade-off over the state-of-the-arts in DPFL.
更多查看译文
关键词
learning,voting-based
AI 理解论文
溯源树
样例
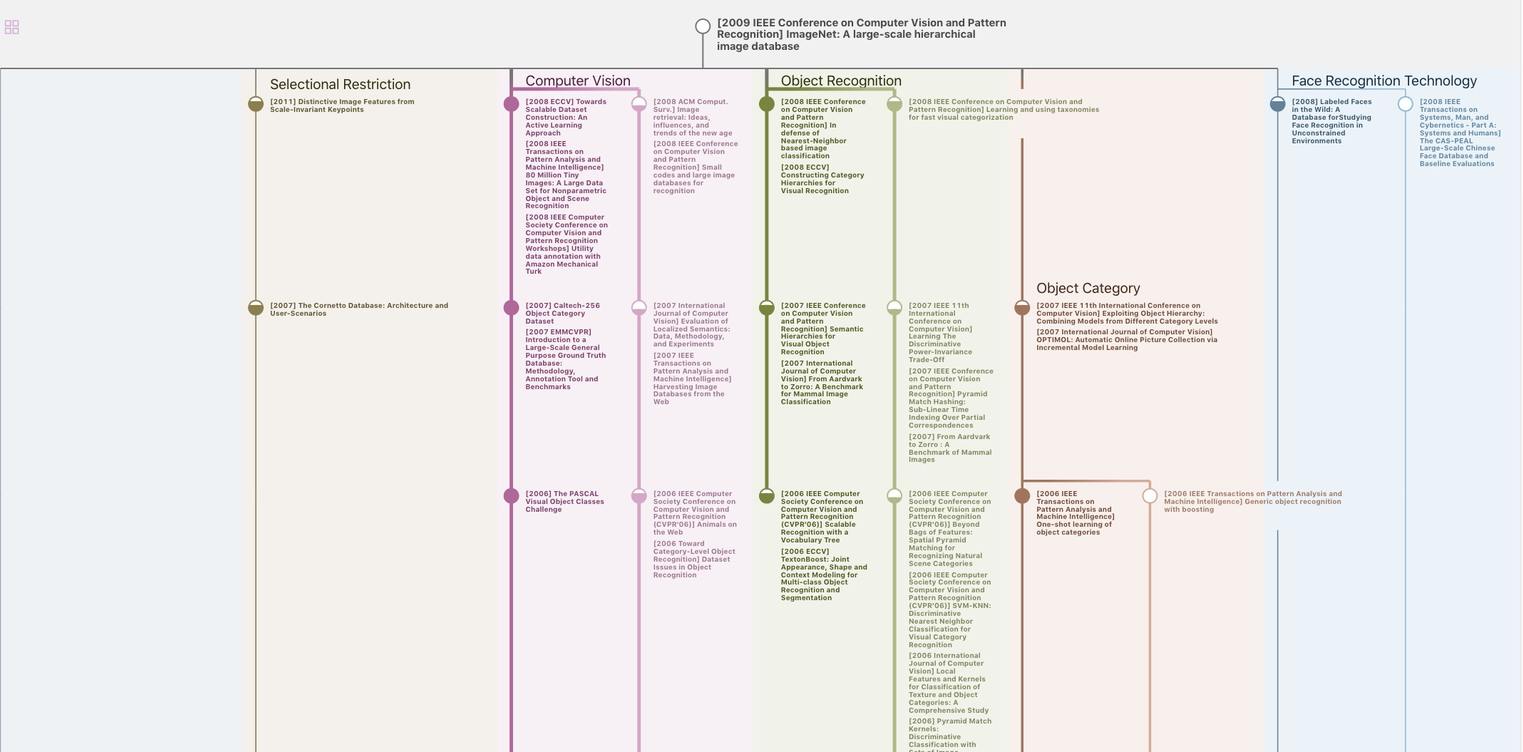
生成溯源树,研究论文发展脉络
Chat Paper
正在生成论文摘要