Solving Zero-Sum One-Sided Partially Observable Stochastic Games
arXiv (Cornell University)(2020)
摘要
Many security and other real-world situations are dynamic in nature and can be modelled as strictly competitive (or zero-sum) dynamic games. In these domains, agents perform actions to affect the environment and receive observations -- possibly imperfect -- about the situation and the effects of the opponent's actions. Moreover, there is no limitation on the total number of actions an agent can perform -- that is, there is no fixed horizon. These settings can be modelled as partially observable stochastic games (POSGs). However, solving general POSGs is computationally intractable, so we focus on a broad subclass of POSGs called one-sided POSGs. In these games, only one agent has imperfect information while their opponent has full knowledge of the current situation. We provide a full picture for solving one-sided POSGs: we (1) give a theoretical analysis of one-sided POSGs and their value functions, (2) show that a variant of a value-iteration algorithm converges in this setting, (3) adapt the heuristic search value-iteration algorithm for solving one-sided POSGs, (4) describe how to use approximate value functions to derive strategies in the game, and (5) demonstrate that our algorithm can solve one-sided POSGs of non-trivial sizes and analyze the scalability of our algorithm in three different domains: pursuit-evasion, patrolling, and search games.
更多查看译文
关键词
games,zero-sum,one-sided
AI 理解论文
溯源树
样例
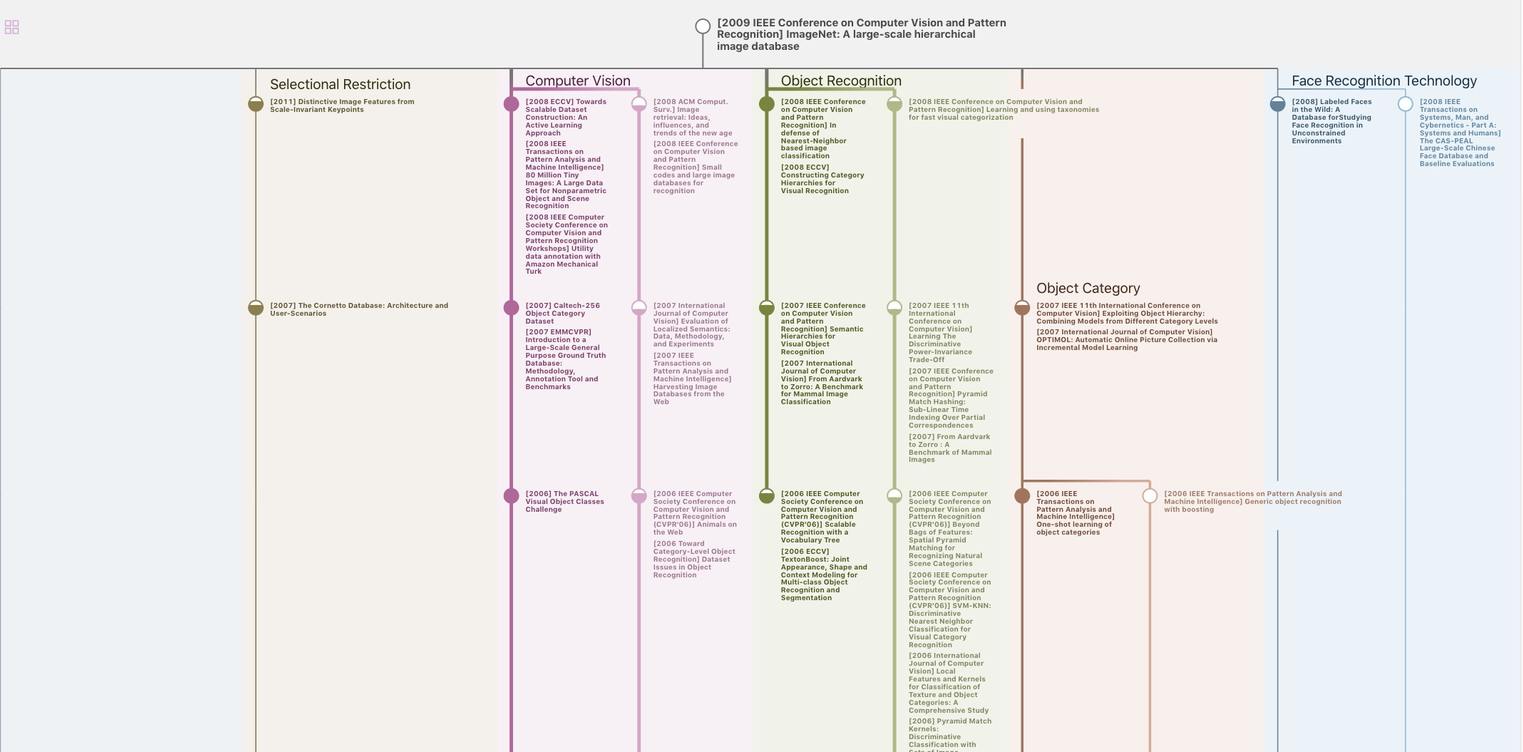
生成溯源树,研究论文发展脉络
Chat Paper
正在生成论文摘要