Exemplar VAE: Linking Generative Models, Nearest Neighbor Retrieval, and Data Augmentation
arXiv (Cornell University)(2020)
摘要
We introduce Exemplar VAEs, a family of generative models that bridge the gap between parametric and non-parametric, exemplar based generative models. Exemplar VAE is a variant of VAE with a non-parametric prior in the latent space based on a Parzen window estimator. To sample from it, one first draws a random exemplar from a training set, then stochastically transforms that exemplar into a latent code and a new observation. We propose retrieval augmented training (RAT) as a way to speed up Exemplar VAE training by using approximate nearest neighbor search in the latent space to define a lower bound on log marginal likelihood. To enhance generalization, model parameters are learned using exemplar leave-one-out and subsampling. Experiments demonstrate the effectiveness of Exemplar VAEs on density estimation and representation learning. Importantly, generative data augmentation using Exemplar VAEs on permutation invariant MNIST and Fashion MNIST reduces classification error from 1.17% to 0.69% and from 8.56% to 8.16%.
更多查看译文
关键词
generative models,data augmentation,nearest neighbor retrieval
AI 理解论文
溯源树
样例
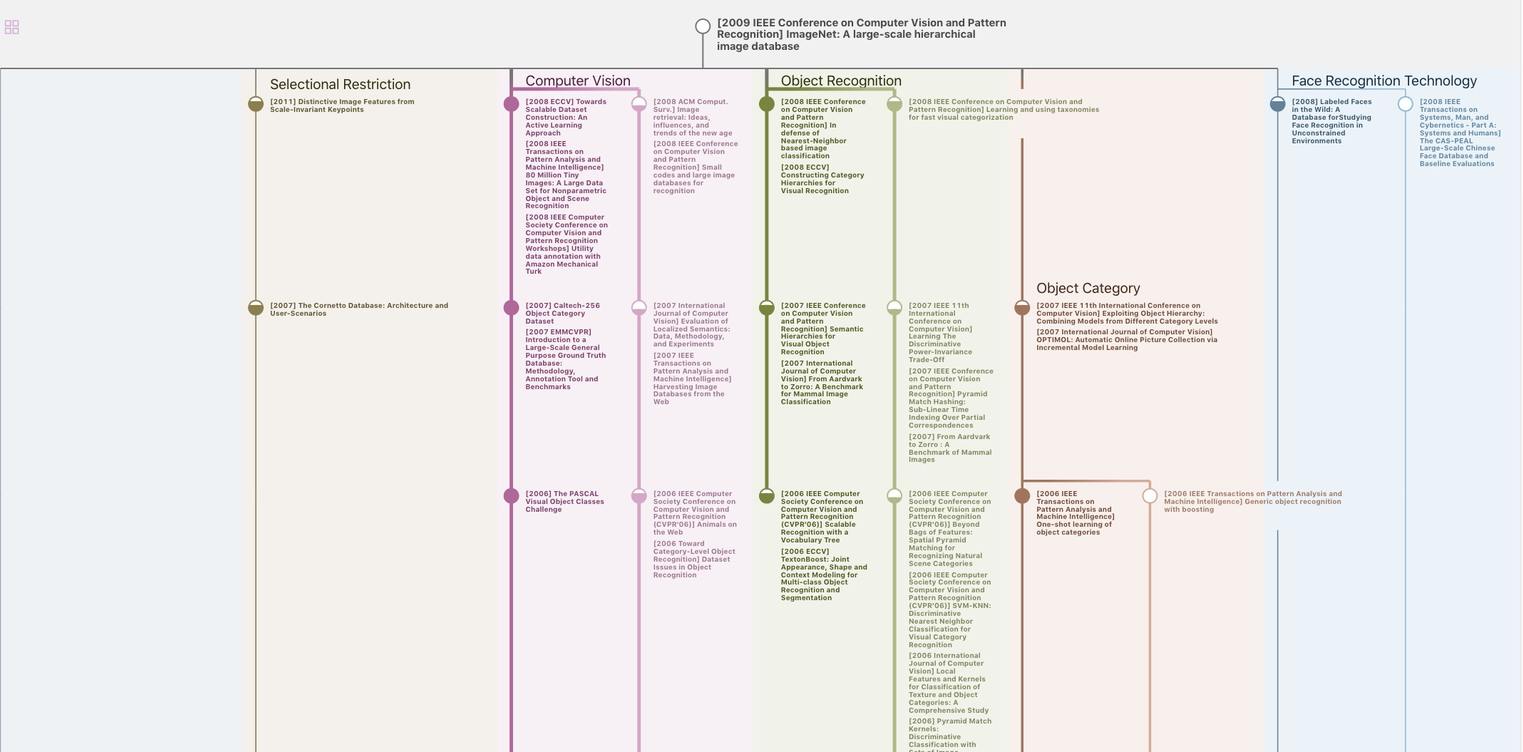
生成溯源树,研究论文发展脉络
Chat Paper
正在生成论文摘要