BAM: A Lightweight and Efficient Balanced Attention Mechanism for Single Image Super Resolution.
arXiv (Cornell University)(2021)
摘要
Attention mechanism has shown enormous potential for single image super-resolution (SISR). However, existing works only proposed some attention mechanism for a specific network. A universal attention mechanism for SISR, which could further improve the performance of networks without attention and provide a baseline for networks with attention, is still lacking. To fit this gap, we propose a lightweight and efficient Balanced Attention Mechanism (BAM), which consists of Avgpool Channel Attention Module (ACAM) and Maxpool Spatial Attention Module (MSAM) in parallel. The information extraction mechanism of ACAM and MSAM effectively filters redundant information, making the overall structure of BAM very lightweight. Owing to the parallel structure, during the gradient backpropagation process of BAM, ACAM and MSAM not only conduct self-optimization, but also mutual optimization so as to generate more balanced attention information. To verify the effectiveness and robustness of BAM, we applied it to 12 state-ofthe-art SISR networks. The results on 4 benchmark datasets demonstrate that BAM can efficiently improve the networks' performance, and for those with attention, the substitution with BAM further reduces the amount of parameters and increase the inference speed. Moreover, ablation experiments were conducted to prove the minimalism of BAM.
更多查看译文
关键词
single image super resolution,efficient balanced attention mechanism
AI 理解论文
溯源树
样例
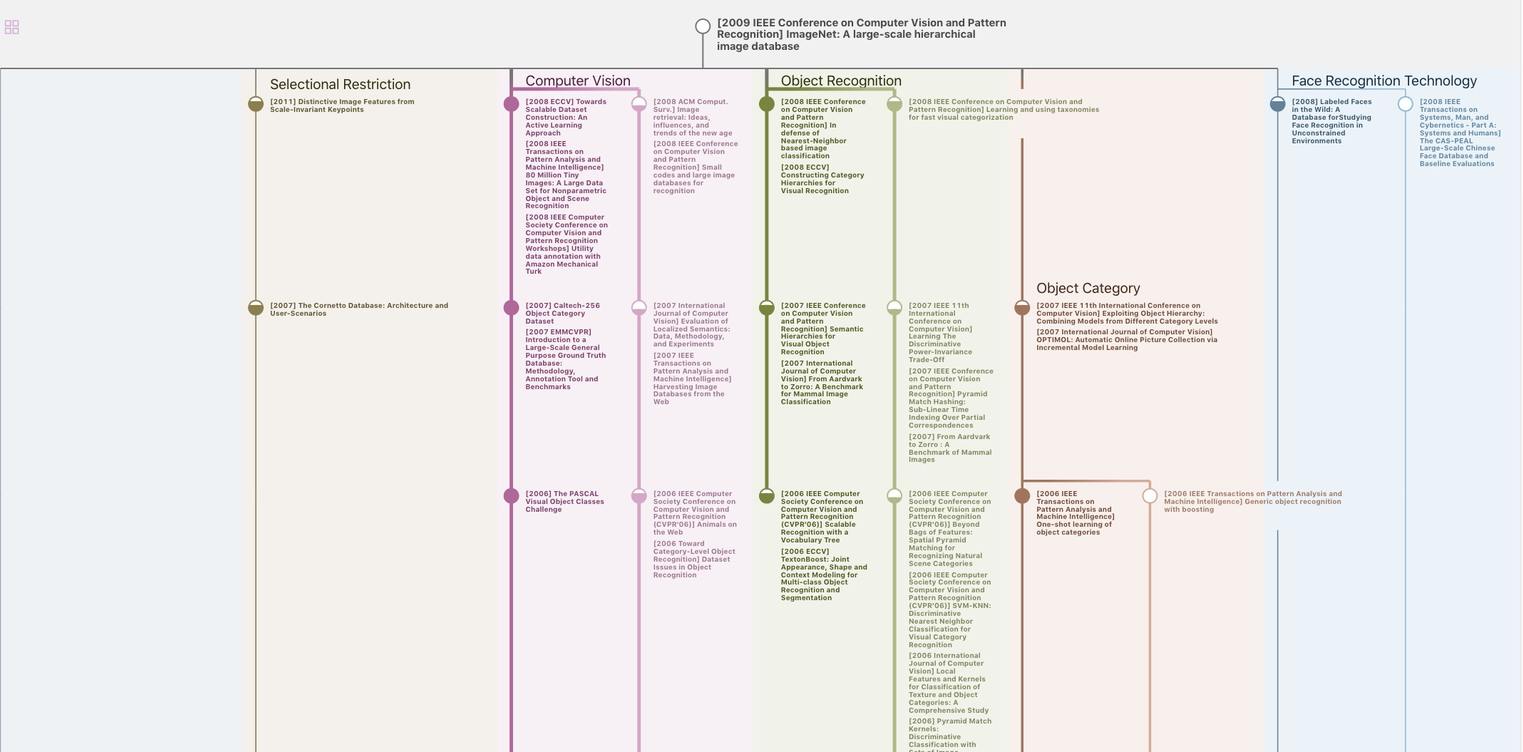
生成溯源树,研究论文发展脉络
Chat Paper
正在生成论文摘要