Bursty exposure on higher-order networks leads to nonlinear infection kernels
arXiv (Cornell University)(2021)
摘要
The co-location of individuals in specific environments is an important prerequisite for exposure to infectious diseases on a social network. Standard epidemic models fail to capture the potential complexity of this scenario by (1) neglecting the hypergraph structure of contacts which typically occur through environments like workplaces, restaurants, and households; and by (2) assuming a linear relationship between the exposure to infected contacts and the risk of infection. Here, we leverage a hypergraph model to embrace the heterogeneity of different environments and the heterogeneity of individual participation in these environments. We find that a bursty exposure to environments can induce a nonlinear relationship between the number of infected participants and infection risk. This allows us to connect complex contagions based on nonlinear infection kernels and threshold models. We then demonstrate how conventional epidemic wisdom can break down with the emergence of discontinuous transitions, super-exponential spread, and regimes of hysteresis.
更多查看译文
关键词
infection,nonlinear,kernels,exposure,higher-order
AI 理解论文
溯源树
样例
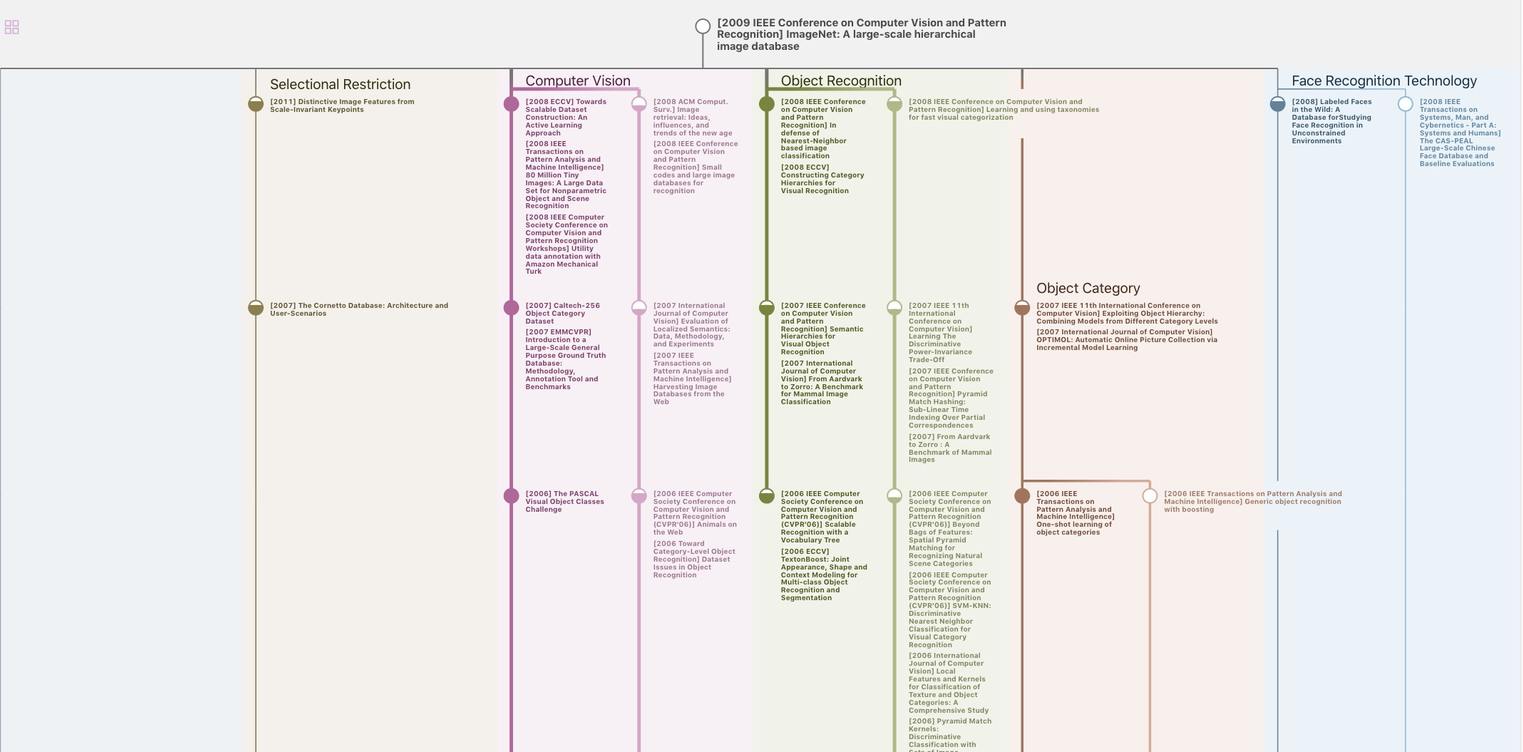
生成溯源树,研究论文发展脉络
Chat Paper
正在生成论文摘要