Deep Neural Network Representation of Density Functional Theory Hamiltonian
arXiv (Cornell University)(2021)
摘要
The marriage of density functional theory (DFT) and deep learning methods has the potential to revolutionize modern research of material science. Here we study the crucial problem of representing DFT Hamiltonian for crystalline materials of arbitrary configurations via deep neural network. A general framework is proposed to deal with the infinite dimensionality and covariance transformation of DFT Hamiltonian matrix in virtue of locality and use message passing neural network together with graph representation for deep learning. Our example study on graphene-based systems demonstrates that high accuracy ($\sim$meV) and good transferability can be obtained for DFT Hamiltonian, ensuring accurate predictions of materials properties without DFT. The Deep Hamiltonian method provides a solution to the accuracy-efficiency dilemma of DFT and opens new opportunities to explore large-scale materials and physics.
更多查看译文
关键词
deep neural network representation,density
AI 理解论文
溯源树
样例
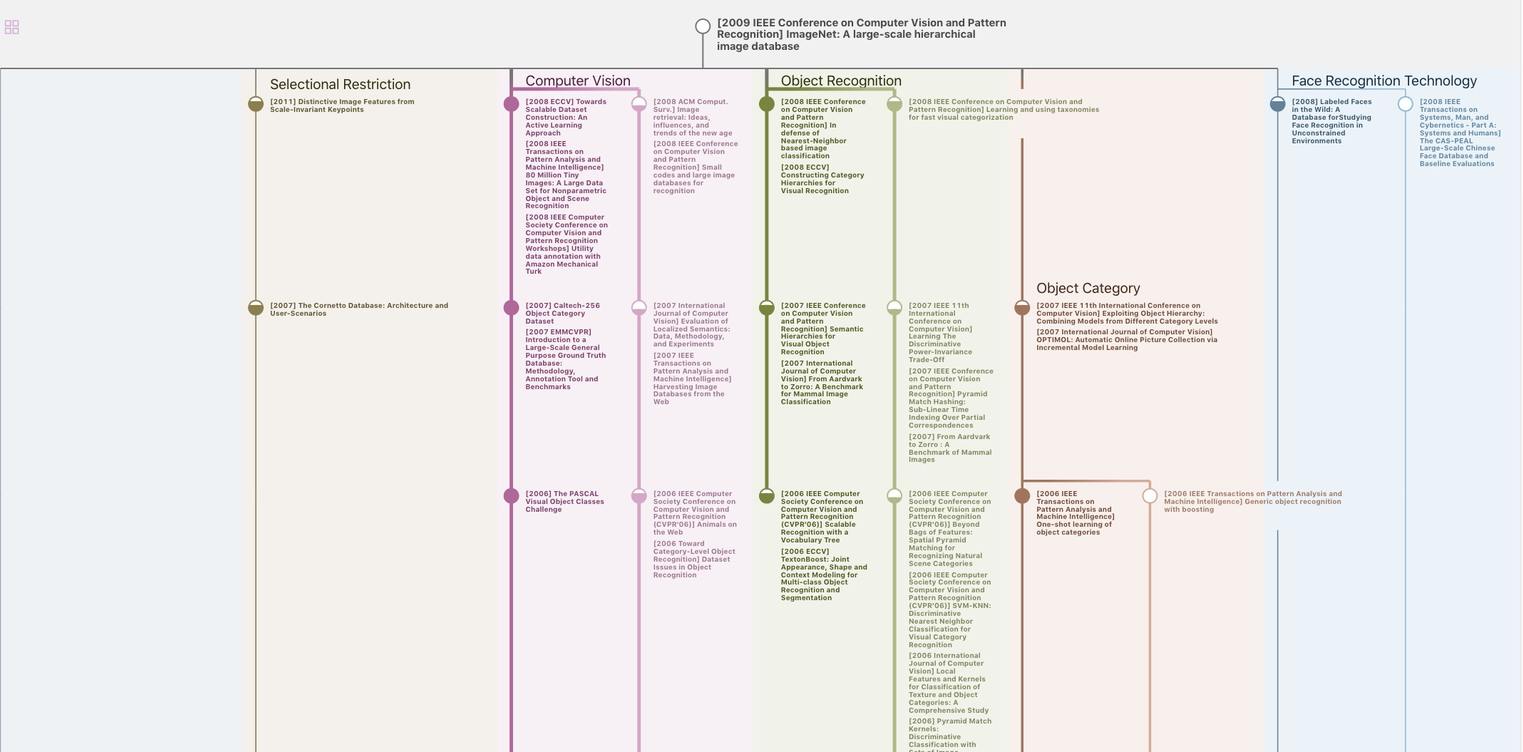
生成溯源树,研究论文发展脉络
Chat Paper
正在生成论文摘要