Nonasymptotic theory for two-layer neural networks: Beyond the bias-variance trade-off
arXiv (Cornell University)(2021)
摘要
Large neural networks have proved remarkably effective in modern deep learning practice, even in the overparametrized regime where the number of active parameters is large relative to the sample size. This contradicts the classical perspective that a machine learning model must trade off bias and variance for optimal generalization. To resolve this conflict, we present a nonasymptotic generalization theory for two-layer neural networks with ReLU activation function by incorporating scaled variation regularization. Interestingly, the regularizer is equivalent to ridge regression from the angle of gradient-based optimization, but plays a similar role to the group lasso in controlling the model complexity. By exploiting this "ridge-lasso duality," we obtain new prediction bounds for all network widths, which reproduce the double descent phenomenon. Moreover, the overparametrized minimum risk is lower than its underparametrized counterpart when the signal is strong, and is nearly minimax optimal over a suitable class of functions. By contrast, we show that overparametrized random feature models suffer from the curse of dimensionality and thus are suboptimal.
更多查看译文
关键词
nonasymptotic theory,neural networks,two-layer,bias-variance,trade-off
AI 理解论文
溯源树
样例
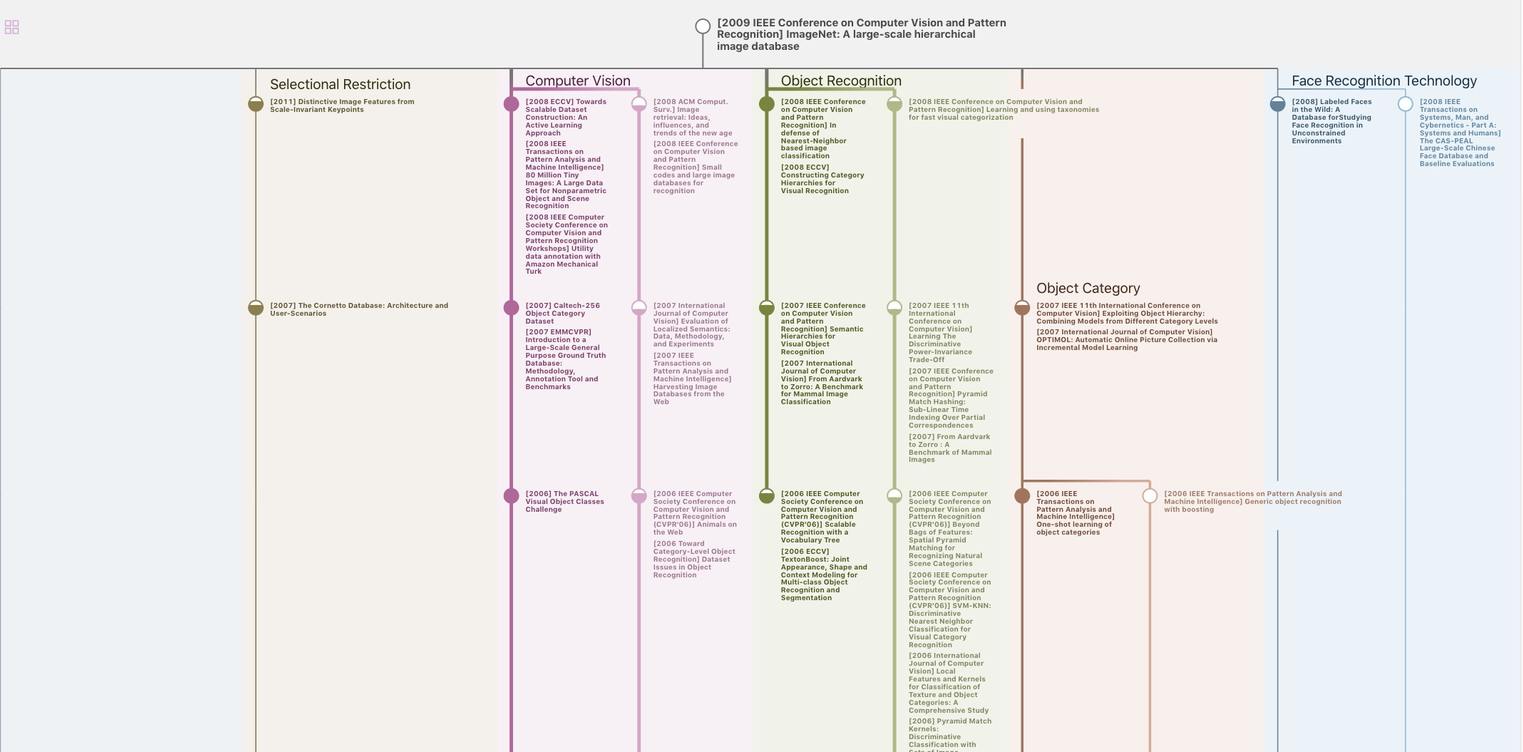
生成溯源树,研究论文发展脉络
Chat Paper
正在生成论文摘要