A Comparative Study on Neural Architectures and Training Methods for Japanese Speech Recognition
arXiv (Cornell University)(2021)
摘要
End-to-end (E2E) modeling is advantageous for automatic speech recognition (ASR) especially for Japanese since word-based tokenization of Japanese is not trivial, and E2E modeling is able to model character sequences directly. This paper focuses on the latest E2E modeling techniques, and investigates their performances on character-based Japanese ASR by conducting comparative experiments. The results are analyzed and discussed in order to understand the relative advantages of long short-term memory (LSTM), and Conformer models in combination with connectionist temporal classification, transducer, and attention-based loss functions. Furthermore, the paper investigates on effectivity of the recent training techniques such as data augmentation (SpecAugment), variational noise injection, and exponential moving average. The best configuration found in the paper achieved the state-of-the-art character error rates of 4.1%, 3.2%, and 3.5% for Corpus of Spontaneous Japanese (CSJ) eval1, eval2, and eval3 tasks, respectively. The system is also shown to be computationally efficient thanks to the efficiency of Conformer transducers.
更多查看译文
关键词
neural architectures,training methods,recognition,speech
AI 理解论文
溯源树
样例
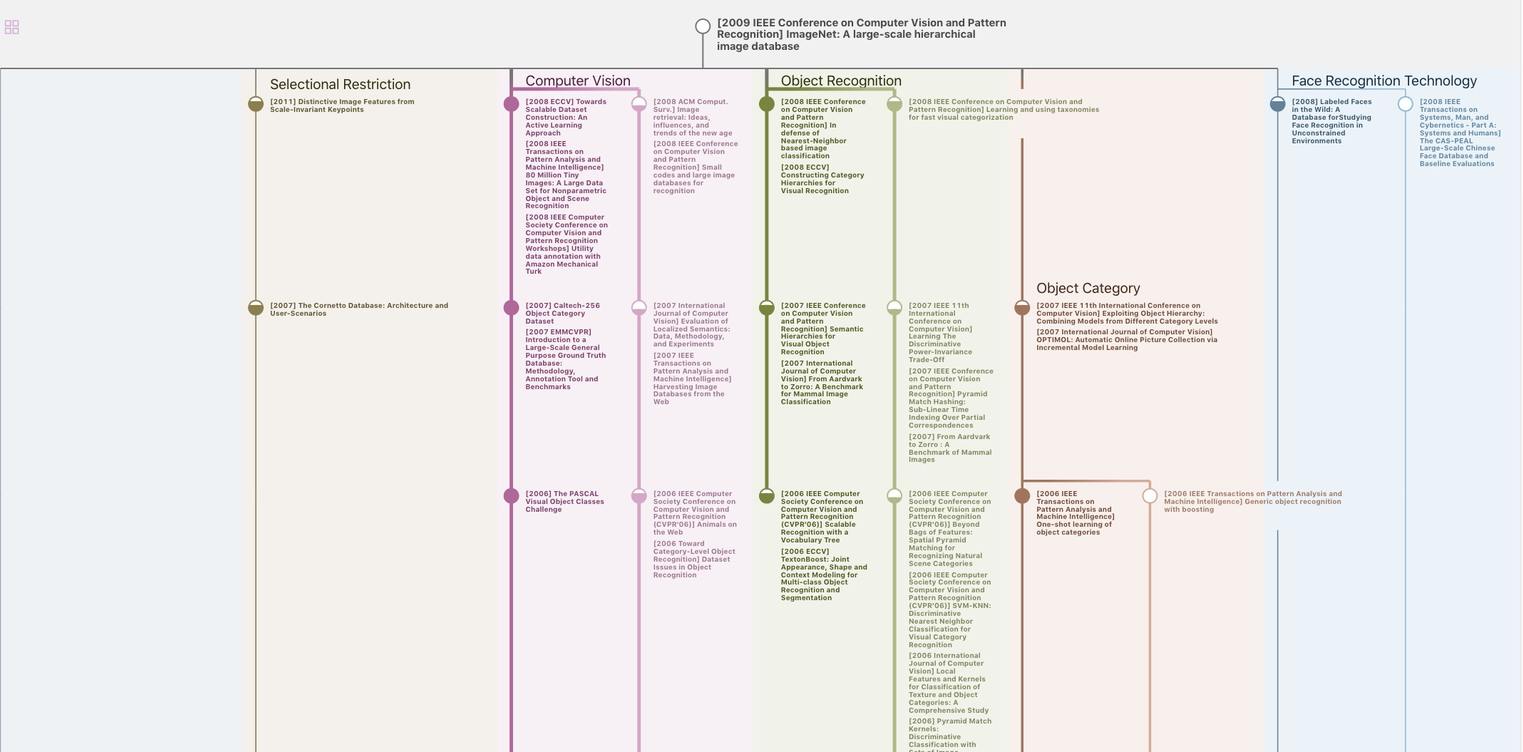
生成溯源树,研究论文发展脉络
Chat Paper
正在生成论文摘要