Data vs classifiers, who wins?
arXiv (Cornell University)(2021)
摘要
The experiments covered by Machine Learning (ML) must consider two important aspects to assess the performance of a model: datasets and algorithms. Robust benchmarks are needed to evaluate the best classifiers. For this, one can adopt gold standard benchmarks available in public repositories. However, it is common not to consider the complexity of the dataset when evaluating. This work proposes a new assessment methodology based on the combination of Item Response Theory (IRT) and Glicko-2, a rating system mechanism generally adopted to assess the strength of players (e.g., chess). For each dataset in a benchmark, the IRT is used to estimate the ability of classifiers, where good classifiers have good predictions for the most difficult test instances. Tournaments are then run for each pair of classifiers so that Glicko-2 updates performance information such as rating value, rating deviation and volatility for each classifier. A case study was conducted hereby which adopted the OpenML-CC18 benchmark as the collection of datasets and pool of various classification algorithms for evaluation. Not all datasets were observed to be really useful for evaluating algorithms, where only 10% were considered really difficult. Furthermore, the existence of a subset containing only 50% of the original amount of OpenML-CC18 was verified, which is equally useful for algorithm evaluation. Regarding the algorithms, the methodology proposed herein identified the Random Forest as the algorithm with the best innate ability.
更多查看译文
关键词
classifiers,data
AI 理解论文
溯源树
样例
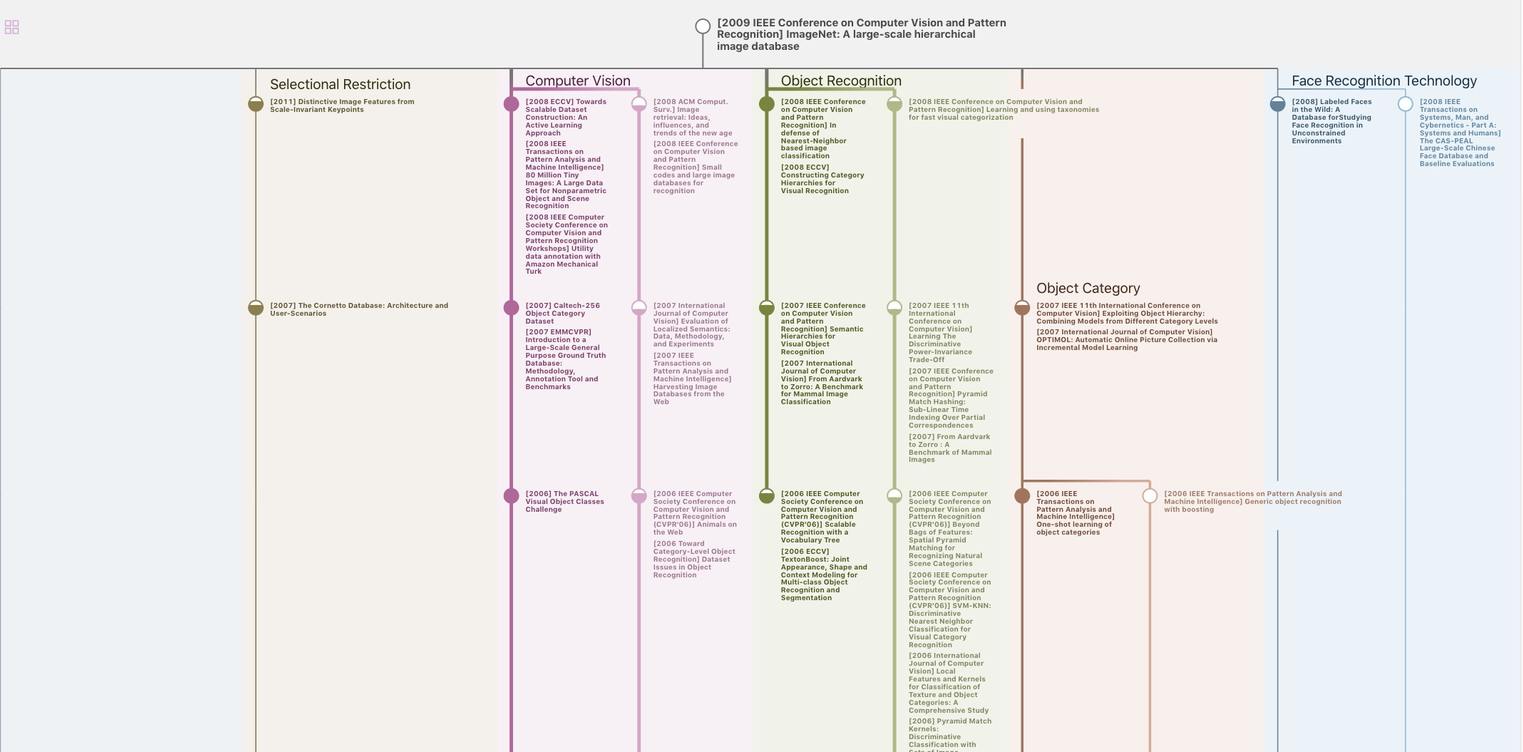
生成溯源树,研究论文发展脉络
Chat Paper
正在生成论文摘要