Shapley values for LightGBM model applied to regime detection
HAL (Le Centre pour la Communication Scientifique Directe)(2021)
摘要
We consider a gradient boosting decision trees (GBDT) approach to predict large S&P 500 price drops from a set of 150 technical, fundamental and macroeconomic features. We report an improved accuracy of GBDT over other machine learning (ML) methods on the S&P 500 futures prices. We show that retaining fewer and carefully selected features provides improvements across all ML approaches. Shapley values have recently been introduced from game theory to the field of ML. They allow for a robust identification of the most important variables predicting stock market crises, and of a local explanation of the crisis probability at each date, through a consistent features attribution. We apply this methodology to analyse in detail the March 2020 financial meltdown, for which the model offered a timely out of sample prediction. This analysis unveils in particular the contrarian predictive role of the tech equity sector before and after the crash.
更多查看译文
关键词
lightgbm model,regime
AI 理解论文
溯源树
样例
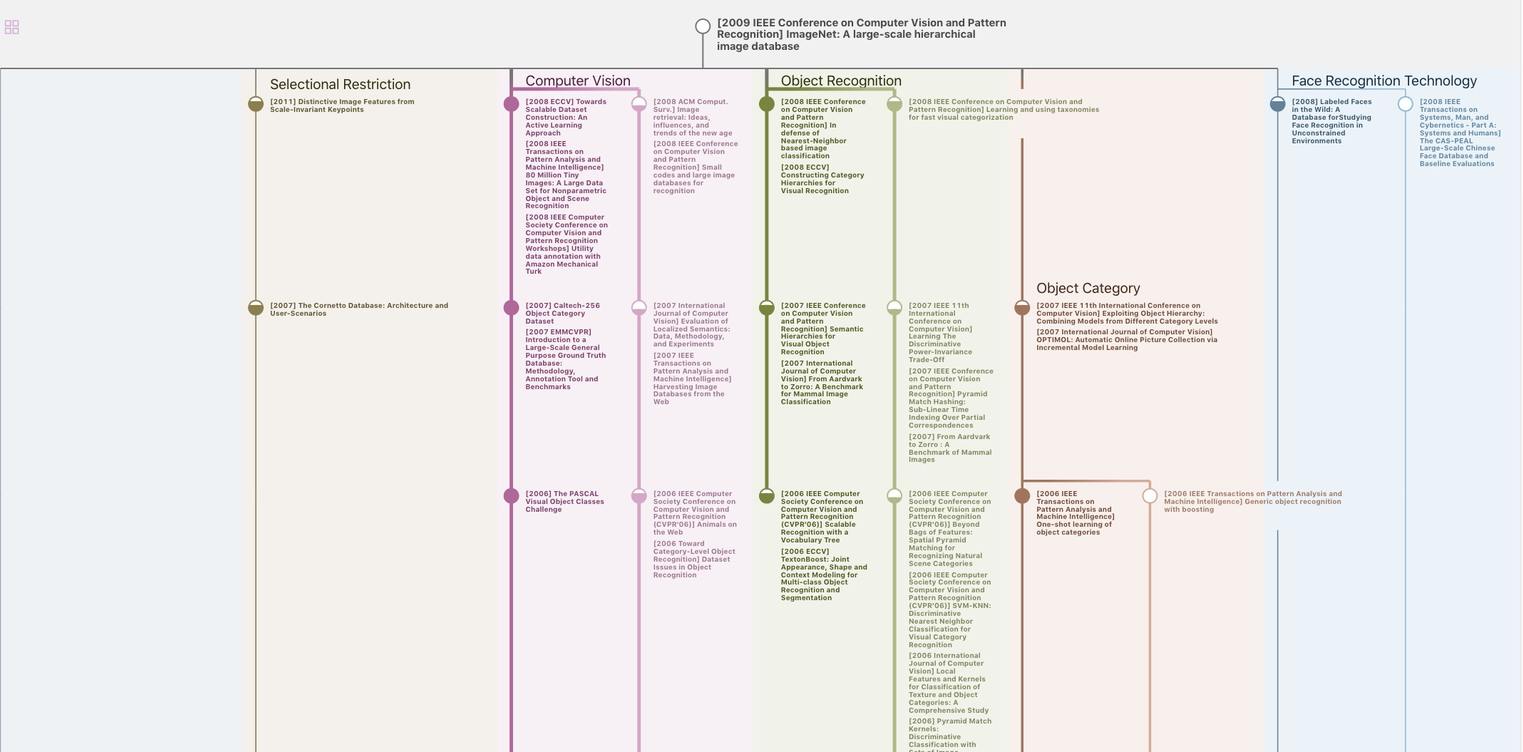
生成溯源树,研究论文发展脉络
Chat Paper
正在生成论文摘要