Towards Controlled Table-to-Text Generation with Scientific Reasoning
CoRR(2023)
摘要
The sheer volume of scientific experimental results and complex technical
statements, often presented in tabular formats, presents a formidable barrier
to individuals acquiring preferred information. The realms of scientific
reasoning and content generation that adhere to user preferences encounter
distinct challenges. In this work, we present a new task for generating fluent
and logical descriptions that match user preferences over scientific tabular
data, aiming to automate scientific document analysis. To facilitate research
in this direction, we construct a new challenging dataset CTRLSciTab consisting
of table-description pairs extracted from the scientific literature, with
highlighted cells and corresponding domain-specific knowledge base. We
evaluated popular pre-trained language models to establish a baseline and
proposed a novel architecture outperforming competing approaches. The results
showed that large models struggle to produce accurate content that aligns with
user preferences. As the first of its kind, our work should motivate further
research in scientific domains.
更多查看译文
关键词
Table-to-text Generation,Scientific Reasoning,Controlled Generation
AI 理解论文
溯源树
样例
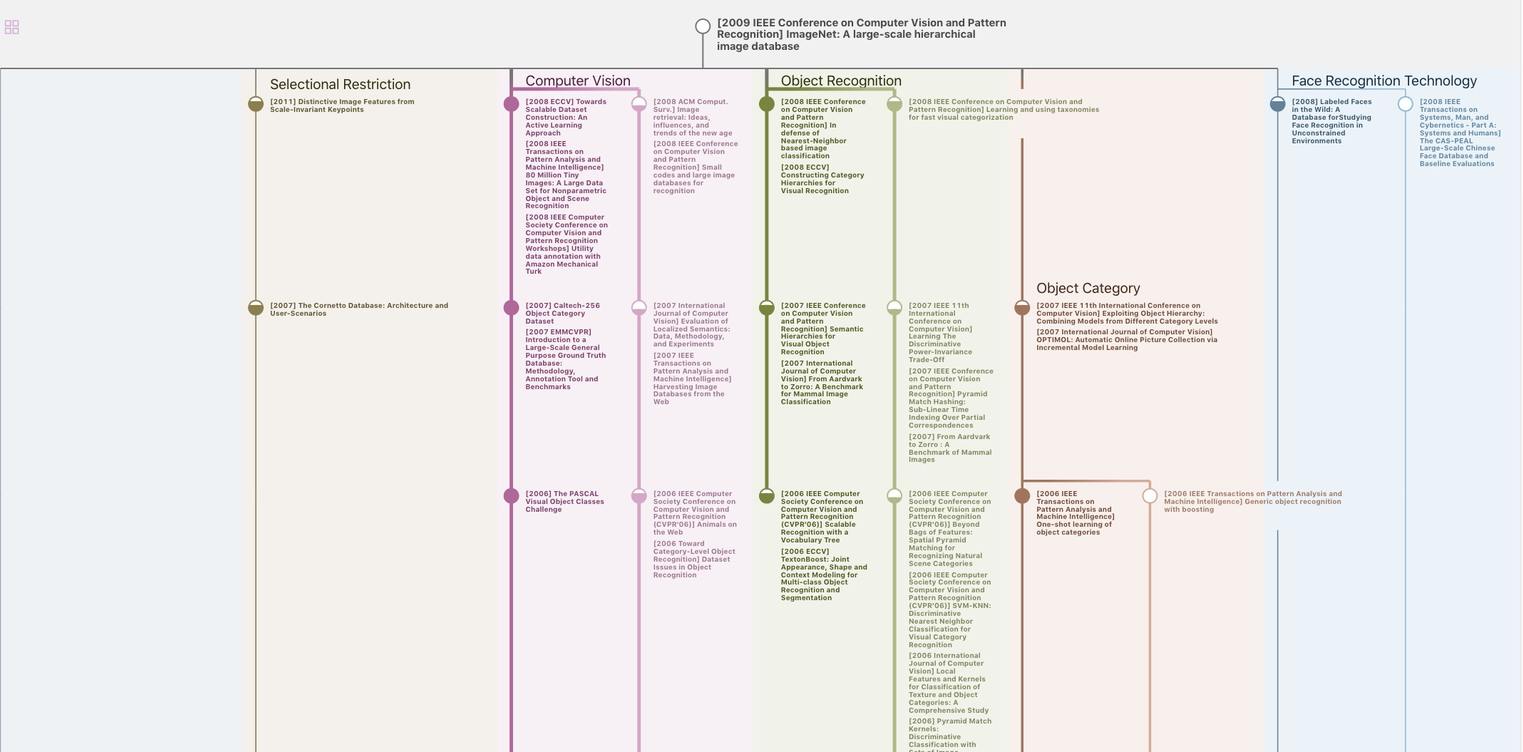
生成溯源树,研究论文发展脉络
Chat Paper
正在生成论文摘要