SimPSI: A Simple Strategy to Preserve Spectral Information in Time Series Data Augmentation
THIRTY-EIGHTH AAAI CONFERENCE ON ARTIFICIAL INTELLIGENCE, VOL 38 NO 13(2024)
关键词
Dynamic Time Warping
AI 理解论文
溯源树
样例
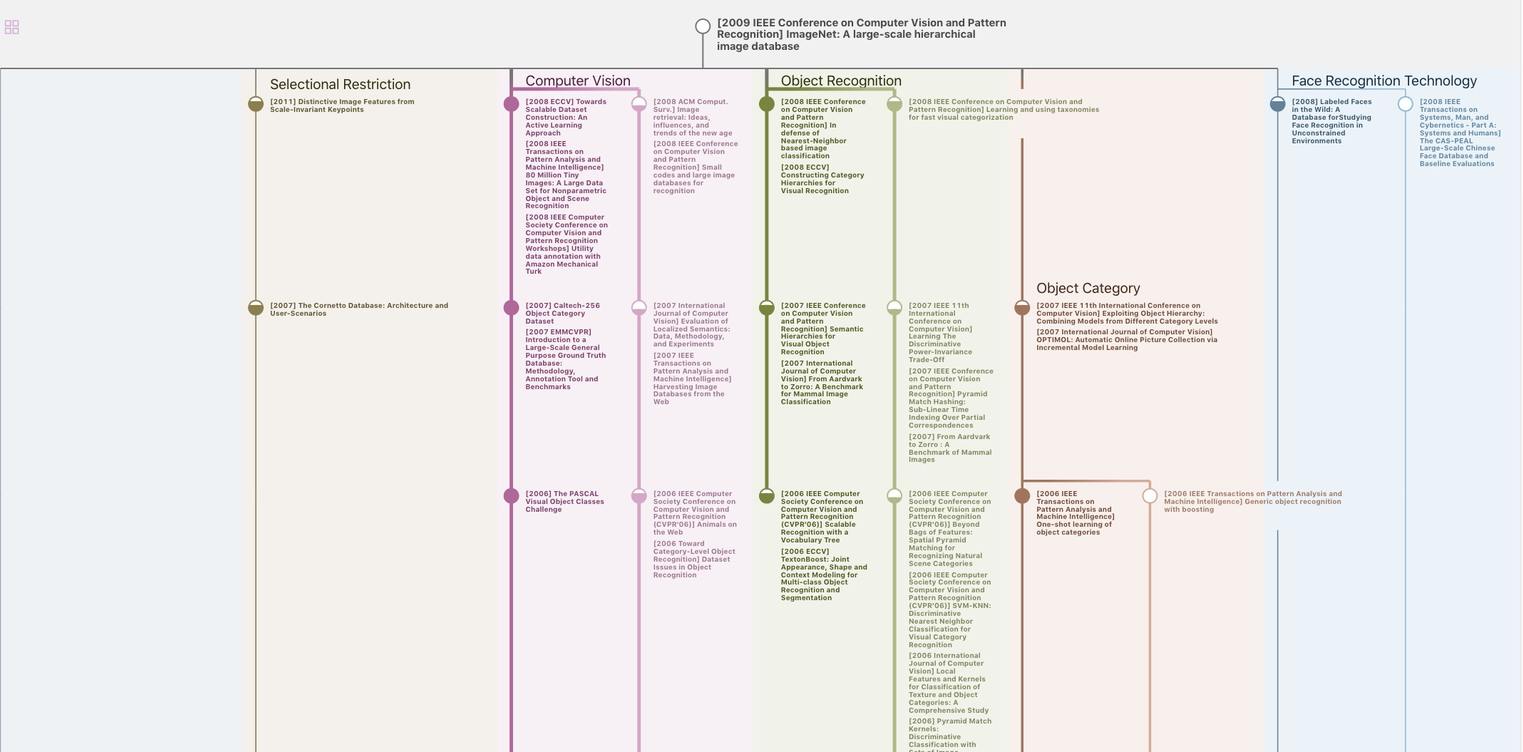
生成溯源树,研究论文发展脉络
Chat Paper
正在生成论文摘要
THIRTY-EIGHTH AAAI CONFERENCE ON ARTIFICIAL INTELLIGENCE, VOL 38 NO 13(2024)