Federated Learning Empowered by Generative Content
CoRR(2023)
摘要
Federated learning (FL) enables leveraging distributed private data for model
training in a privacy-preserving way. However, data heterogeneity significantly
limits the performance of current FL methods. In this paper, we propose a novel
FL framework termed FedGC, designed to mitigate data heterogeneity issues by
diversifying private data with generative content. FedGC is a
simple-to-implement framework as it only introduces a one-shot step of data
generation. In data generation, we summarize three crucial and worth-exploring
aspects (budget allocation, prompt design, and generation guidance) and propose
three solution candidates for each aspect. Specifically, to achieve a better
trade-off between data diversity and fidelity for generation guidance, we
propose to generate data based on the guidance of prompts and real data
simultaneously. The generated data is then merged with private data to
facilitate local model training. Such generative data increases the diversity
of private data to prevent each client from fitting the potentially biased
private data, alleviating the issue of data heterogeneity. We conduct a
systematic empirical study on FedGC, covering diverse baselines, datasets,
scenarios, and modalities. Interesting findings include (1) FedGC consistently
and significantly enhances the performance of FL methods, even when notable
disparities exist between generative and private data; (2) FedGC achieves both
better performance and privacy-preservation. We wish this work can inspire
future works to further explore the potential of enhancing FL with generative
content.
更多查看译文
AI 理解论文
溯源树
样例
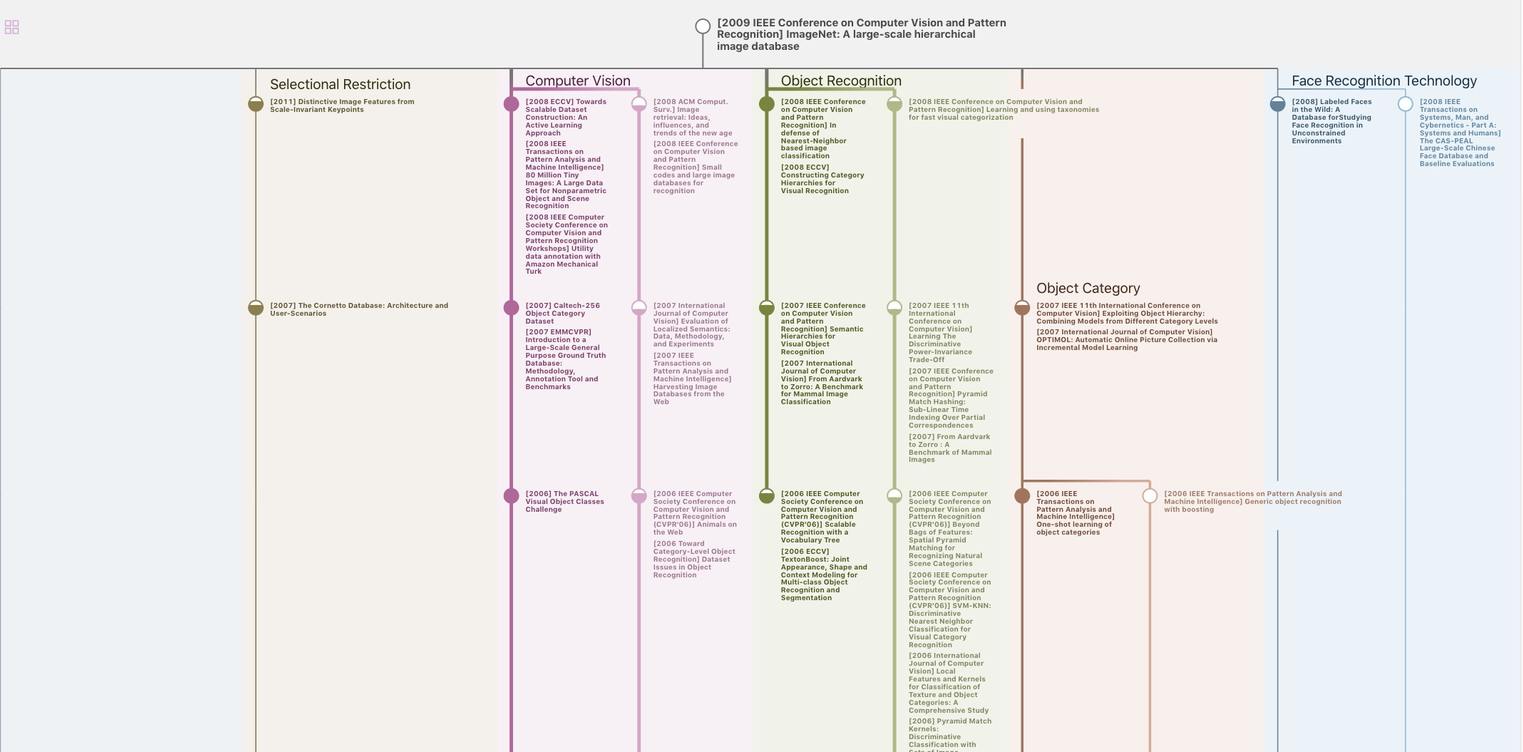
生成溯源树,研究论文发展脉络
Chat Paper
正在生成论文摘要