Stellar Spectra Fitting with Amortized Neural Posterior Estimation and nbi
CoRR(2023)
摘要
Modern surveys often deliver hundreds of thousands of stellar spectra at
once, which are fit to spectral models to derive stellar parameters/labels.
Therefore, the technique of Amortized Neural Posterior Estimation (ANPE) stands
out as a suitable approach, which enables the inference of large number of
targets as sub-linear/constant computational costs. Leveraging our new nbi
software package, we train an ANPE model for the APOGEE survey and demonstrate
its efficacy on both mock and real APOGEE stellar spectra. Unique to the nbi
package is its out-of-the-box functionality on astronomical inverse problems
with sequential data. As such, we have been able to acquire the trained model
with minimal effort. We introduce an effective approach to handling the
measurement noise properties inherent in spectral data, which utilizes the
actual uncertainties in the observed data. This allows training data to
resemble observed data, an aspect that is crucial for ANPE applications. Given
the association of spectral data properties with the observing instrument, we
discuss the utility of an ANPE "model zoo," where models are trained for
specific instruments and distributed under the nbi framework to facilitate
real-time stellar parameter inference.
更多查看译文
AI 理解论文
溯源树
样例
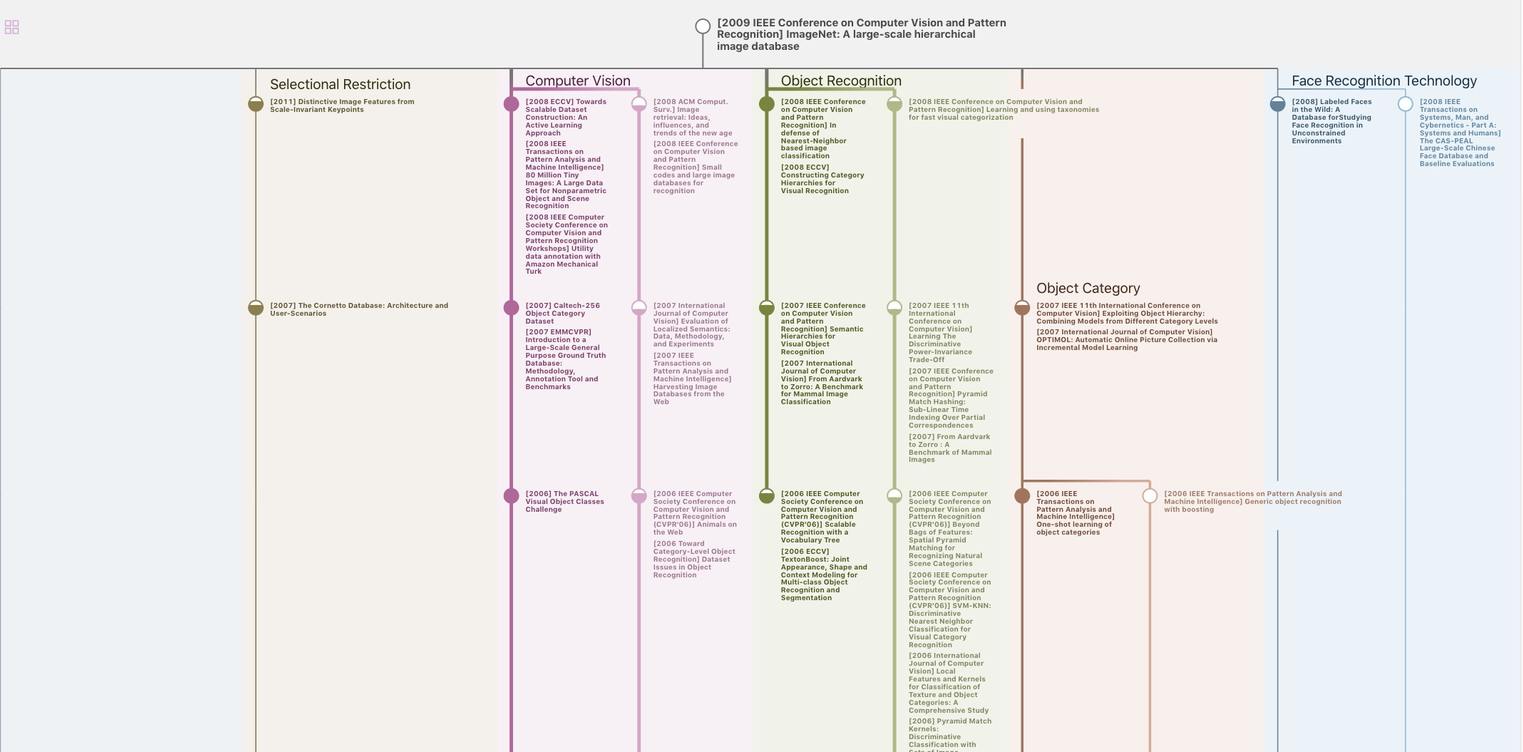
生成溯源树,研究论文发展脉络
Chat Paper
正在生成论文摘要