Using Deep Neural Networks to Improve the Precision of Fast-Sampled Particle Timing Detectors
Computer Science(2024)
摘要
Measurements from particle timing detectors are often affected by the time walk effect caused by statistical fluctuations in the charge deposited by passing particles. The constant fraction discriminator (CFD) algorithm is frequently used to mitigate this effect both in test setups and in running experiments, such as the CMS-PPS system at the CERN's LHC. The CFD is simple and effective but does not leverage all voltage samples in a time series. Its per-formance could be enhanced with deep neural networks, which are commonly used for time series analysis, including computing the particle arrival time. We evaluated various neural network architectures using data acquired at the test beam facility in the DESY-II synchrotron, where a precise MCP (MicroChan-nel Plate) detector was installed in addition to PPS diamond timing detectors. MCP measurements were used as a reference to train the networks and com-pare the results with the standard CFD method. Ultimately, we improved the timing precision by 8% to 23%, depending on the detector's readout channel. The best results were obtained using a UNet-based model, which outperformed classical convolutional networks and the multilayer perceptron.
更多查看译文
关键词
deep neural networks,timing detectors,diamond sensors,time series analysis,time walk correction,CERN,Precision Proton Spectrometer
AI 理解论文
溯源树
样例
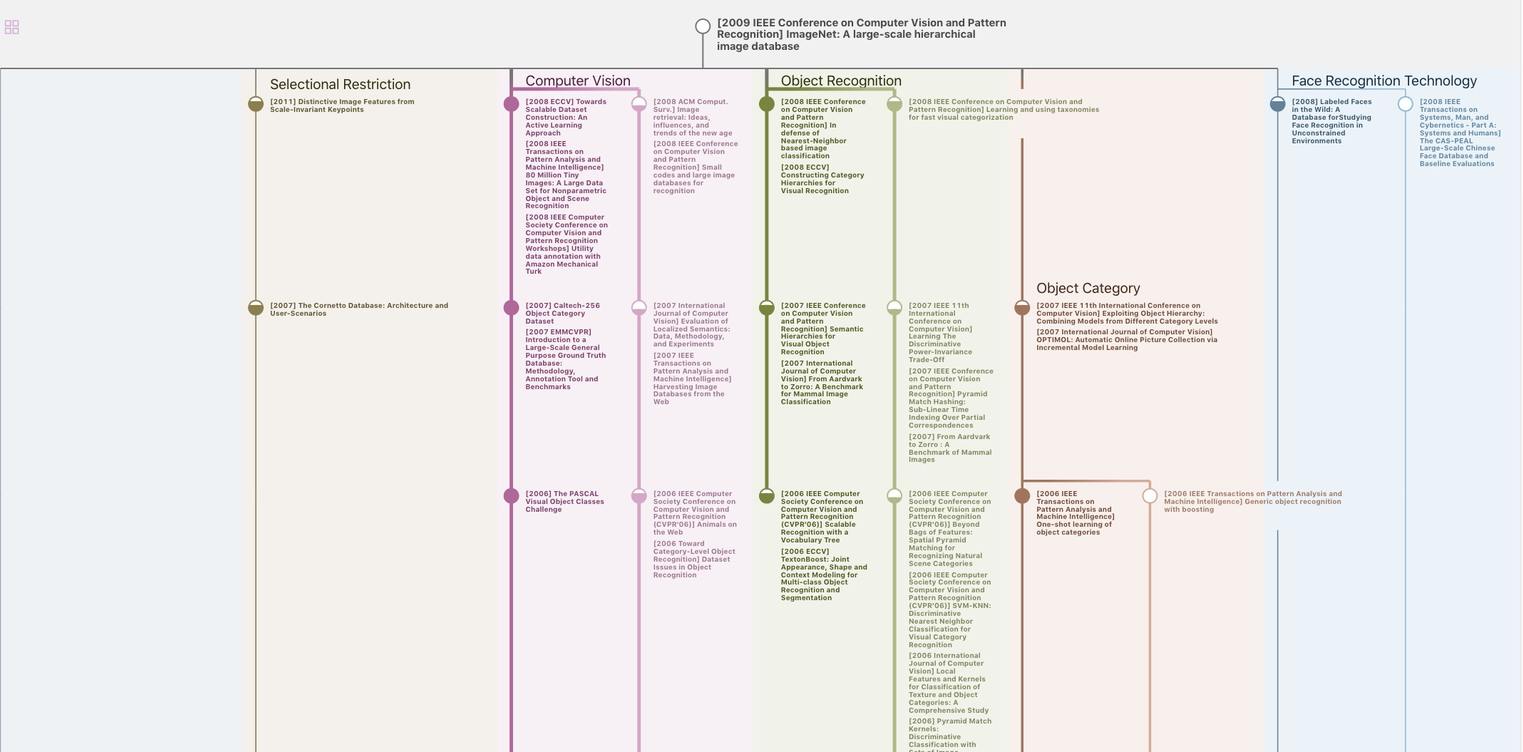
生成溯源树,研究论文发展脉络
Chat Paper
正在生成论文摘要