Contrastive Multi-view Subspace Clustering of Hyperspectral Images based on Graph Convolutional Networks
CoRR(2023)
摘要
High-dimensional and complex spectral structures make the clustering of
hyperspectral images (HSI) a challenging task. Subspace clustering is an
effective approach for addressing this problem. However, current subspace
clustering algorithms are primarily designed for a single view and do not fully
exploit the spatial or textural feature information in HSI. In this study,
contrastive multi-view subspace clustering of HSI was proposed based on graph
convolutional networks. Pixel neighbor textural and spatial-spectral
information were sent to construct two graph convolutional subspaces to learn
their affinity matrices. To maximize the interaction between different views, a
contrastive learning algorithm was introduced to promote the consistency of
positive samples and assist the model in extracting robust features. An
attention-based fusion module was used to adaptively integrate these affinity
matrices, constructing a more discriminative affinity matrix. The model was
evaluated using four popular HSI datasets: Indian Pines, Pavia University,
Houston, and Xu Zhou. It achieved overall accuracies of 97.61%, 96.69%, 87.21%,
and 97.65%, respectively, and significantly outperformed state-of-the-art
clustering methods. In conclusion, the proposed model effectively improves the
clustering accuracy of HSI.
更多查看译文
关键词
Contrastive learning,hyperspectral images (HSI),multi-view clustering,remote sensing,subspace clustering
AI 理解论文
溯源树
样例
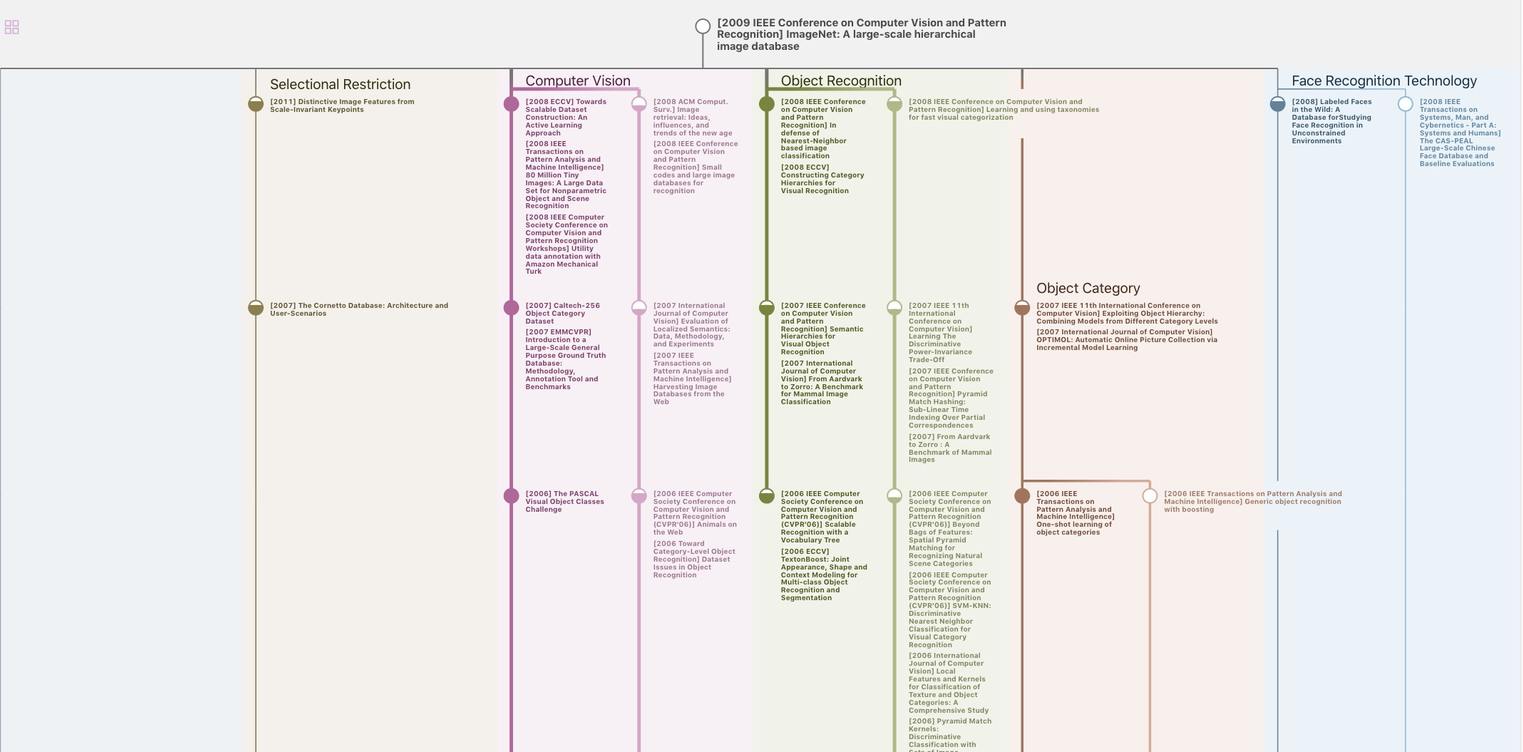
生成溯源树,研究论文发展脉络
Chat Paper
正在生成论文摘要