Enhancing the Sensitivity of Atom-Based Microwave-Field Electrometry Using a Mach-Zehnder Interferometer
PHYSICAL REVIEW APPLIED(2023)
Abstract
We demonstrate the sensitivity enhancement of measurement of microwave electric fields by use of the full information of the complex atomic susceptibility. The transfer characteristics of both the microwave electric fields and the noise relevant to atoms, which are mapped to two quadrature components of the probe light, are systematically measured with use of a Mach-Zehnder interferometer. On the basis of the readout of the full complex atomic susceptibility, a sensitivity enhancement of 12 dB is achieved when the probe light has red detuning of 6 MHz. The results shed light on increasing the sensitivity of atomic sensors of microwave electric fields that is beyond the capability of the traditional antenna.
MoreTranslated text
求助PDF
上传PDF
View via Publisher
AI Read Science
AI Summary
AI Summary is the key point extracted automatically understanding the full text of the paper, including the background, methods, results, conclusions, icons and other key content, so that you can get the outline of the paper at a glance.
Example
Background
Key content
Introduction
Methods
Results
Related work
Fund
Key content
- Pretraining has recently greatly promoted the development of natural language processing (NLP)
- We show that M6 outperforms the baselines in multimodal downstream tasks, and the large M6 with 10 parameters can reach a better performance
- We propose a method called M6 that is able to process information of multiple modalities and perform both single-modal and cross-modal understanding and generation
- The model is scaled to large model with 10 billion parameters with sophisticated deployment, and the 10 -parameter M6-large is the largest pretrained model in Chinese
- Experimental results show that our proposed M6 outperforms the baseline in a number of downstream tasks concerning both single modality and multiple modalities We will continue the pretraining of extremely large models by increasing data to explore the limit of its performance
Upload PDF to Generate Summary
Must-Reading Tree
Example
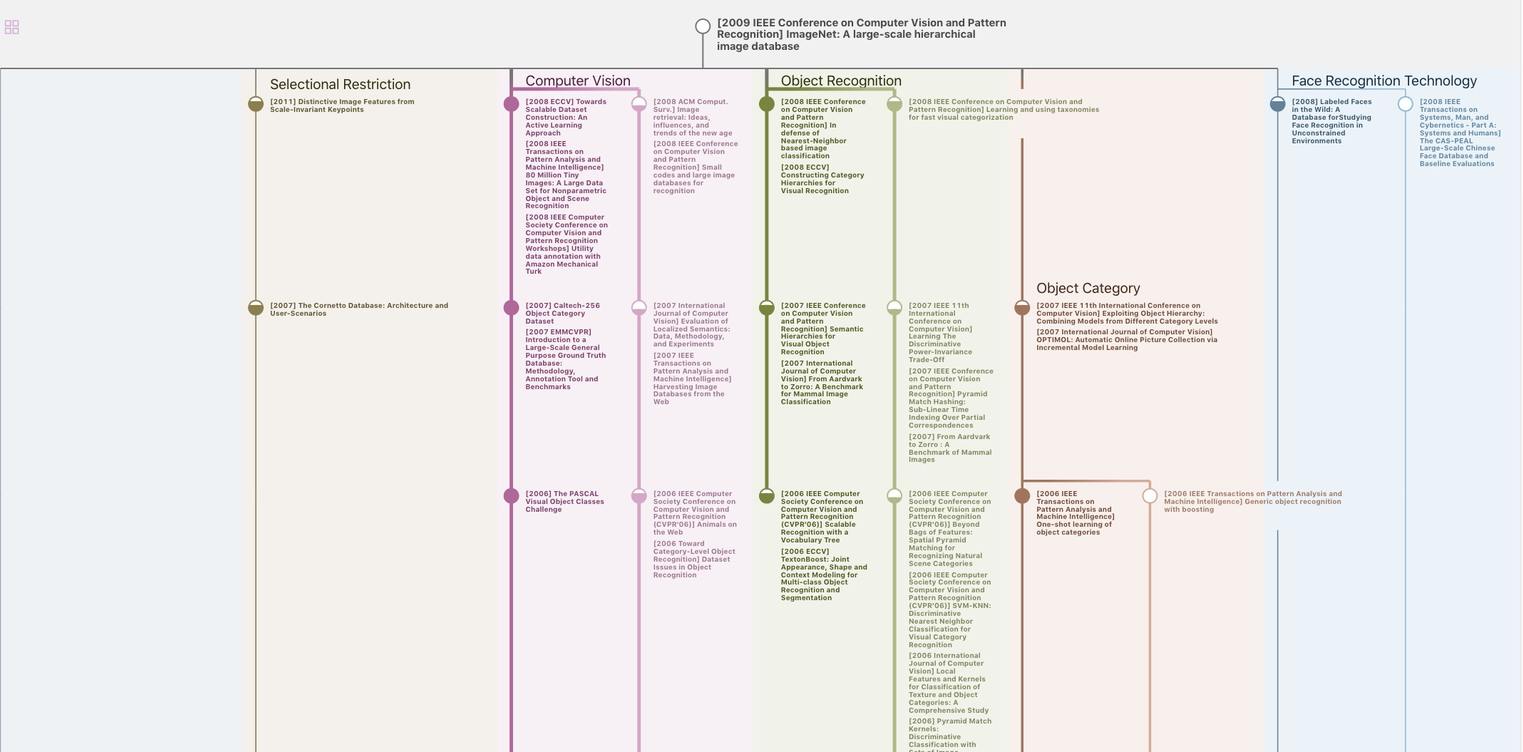
Generate MRT to find the research sequence of this paper
Related Papers
1996
被引用88 | 浏览
2007
被引用23 | 浏览
2002
被引用43 | 浏览
2007
被引用28 | 浏览
2007
被引用130 | 浏览
1994
被引用162 | 浏览
1995
被引用867 | 浏览
2013
被引用251 | 浏览
2012
被引用530 | 浏览
2017
被引用106 | 浏览
2015
被引用108 | 浏览
2017
被引用12940 | 浏览
2019
被引用107 | 浏览
2022
被引用15 | 浏览
Data Disclaimer
The page data are from open Internet sources, cooperative publishers and automatic analysis results through AI technology. We do not make any commitments and guarantees for the validity, accuracy, correctness, reliability, completeness and timeliness of the page data. If you have any questions, please contact us by email: report@aminer.cn
Chat Paper