Machine learning assisted quantum super-resolution microscopy
arXiv (Cornell University)(2021)
摘要
One of the main characteristics of optical imaging systems is the spatial resolution, which is restricted by the diffraction limit to approximately half the wavelength of the incident light. Along with the recently developed classical super-resolution techniques, which aim at breaking the diffraction limit in classical systems, there is a class of quantum super-resolution techniques which leverage the non-classical nature of the optical signals radiated by quantum emitters, the so-called antibunching super-resolution microscopy. This approach can ensure a factor of $\sqrt{n}$ improvement in the spatial resolution by measuring the n-th order autocorrelation function. The main bottleneck of the antibunching super-resolution microscopy is the time-consuming acquisition of multi-photon event histograms. We present a machine learning-assisted approach for the realization of rapid antibunching super-resolution imaging and demonstrate 12 times speed-up compared to conventional, fitting-based autocorrelation measurements. The developed framework paves the way to the practical realization of scalable quantum super-resolution imaging devices that can be compatible with various types of quantum emitters.
更多查看译文
关键词
microscopy,quantum,learning,super-resolution
AI 理解论文
溯源树
样例
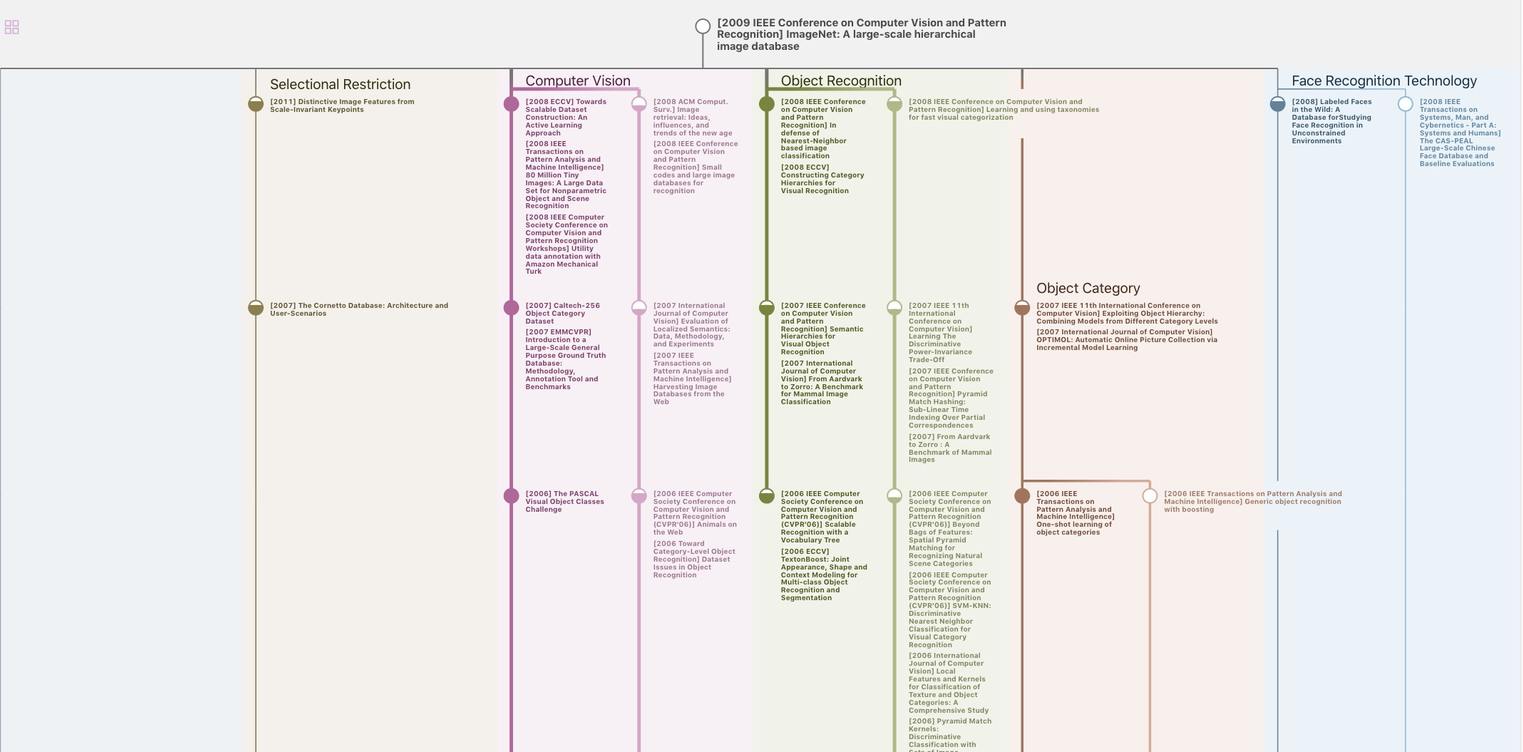
生成溯源树,研究论文发展脉络
Chat Paper
正在生成论文摘要