AI-assisted quantum many-body computation beyond Markov-chain Monte Carlo
arXiv (Cornell University)(2021)
摘要
We find artificial neural networks can constructively help the Monte Carlo computations to provide better sampling and complete absence of autocorrelation between configurations in the study of classical and quantum many-body systems. We design generic generative neural-network architecture for the Ising and Hubbard models on two-dimensional lattices and demonstrate it can overcome the traditional computational complexity as well as the difficulty in generating uncorrelated configurations, irrespective of the system locating at the classical critical point, antiferromagnetic Mott insulator, correlated Dirac semimetal or the Gross-Neveu quantum criticality. Our work therefore paves the avenue for highly efficient AI-assisted quantum many-body computation beyond the Markov-chain Monte Carlo.
更多查看译文
关键词
quantum,ai-assisted,many-body,markov-chain
AI 理解论文
溯源树
样例
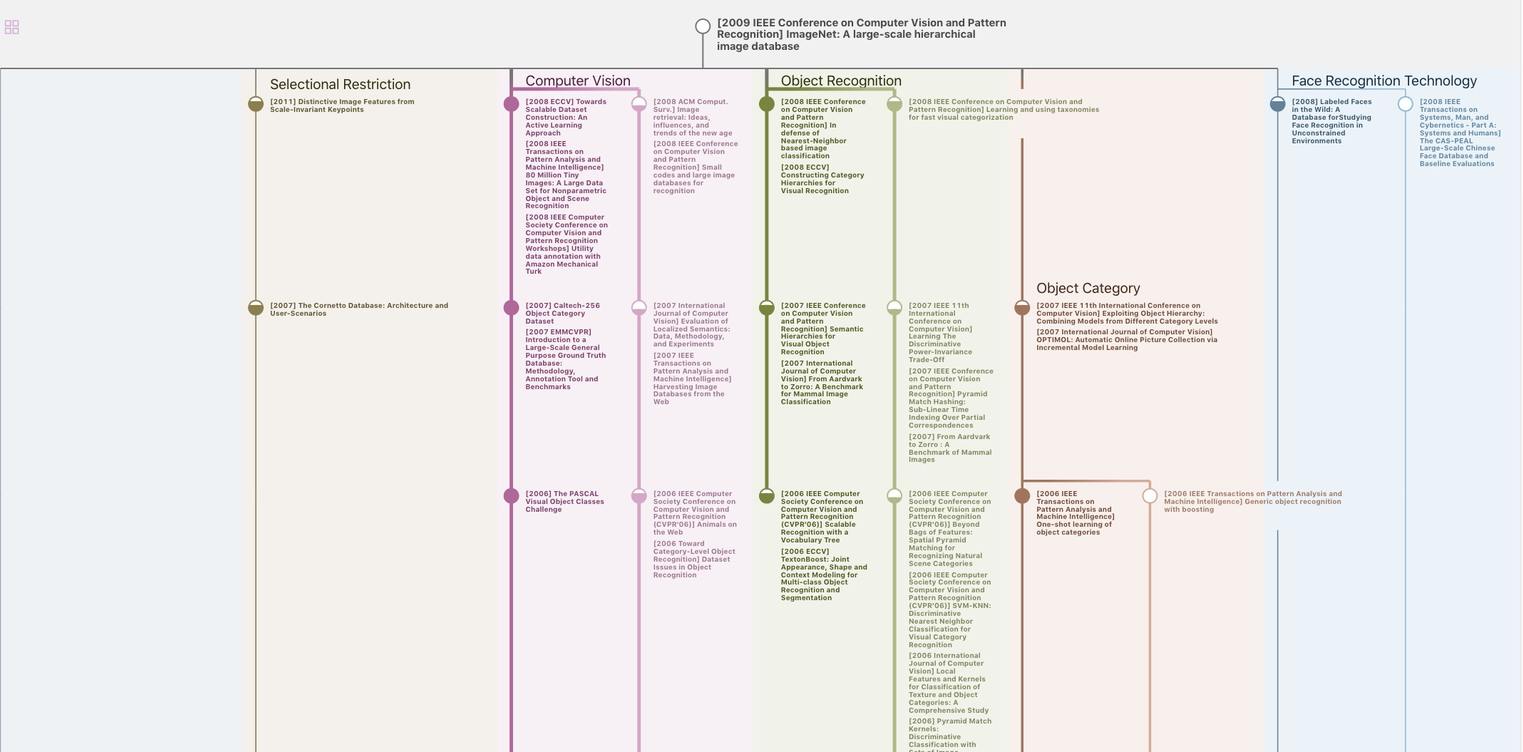
生成溯源树,研究论文发展脉络
Chat Paper
正在生成论文摘要