PDBL: Improving Histopathological Tissue Classification with Plug-and-Play Pyramidal Deep-Broad Learning
arXiv (Cornell University)(2021)
摘要
Histopathological tissue classification is a fundamental task in pathomics cancer research. Precisely differentiating different tissue types is a benefit for the downstream researches, like cancer diagnosis, prognosis and etc. Existing works mostly leverage the popular classification backbones in computer vision to achieve histopathological tissue classification. In this paper, we proposed a super lightweight plug-and-play module, named Pyramidal Deep-Broad Learning (PDBL), for any well-trained classification backbone to further improve the classification performance without a re-training burden. We mimic how pathologists observe pathology slides in different magnifications and construct an image pyramid for the input image in order to obtain the pyramidal contextual information. For each level in the pyramid, we extract the multi-scale deep-broad features by our proposed Deep-Broad block (DB-block). We equipped PDBL in three popular classification backbones, ShuffLeNetV2, EfficientNetb0, and ResNet50 to evaluate the effectiveness and efficiency of our proposed module on two datasets (Kather Multiclass Dataset and the LC25000 Dataset). Experimental results demonstrate the proposed PDBL can steadily improve the tissue-level classification performance for any CNN backbones, especially for the lightweight models when given a small among of training samples (less than 10%), which greatly saves the computational time and annotation efforts.
更多查看译文
关键词
histopathological tissue classification,plug-and-play,deep-broad
AI 理解论文
溯源树
样例
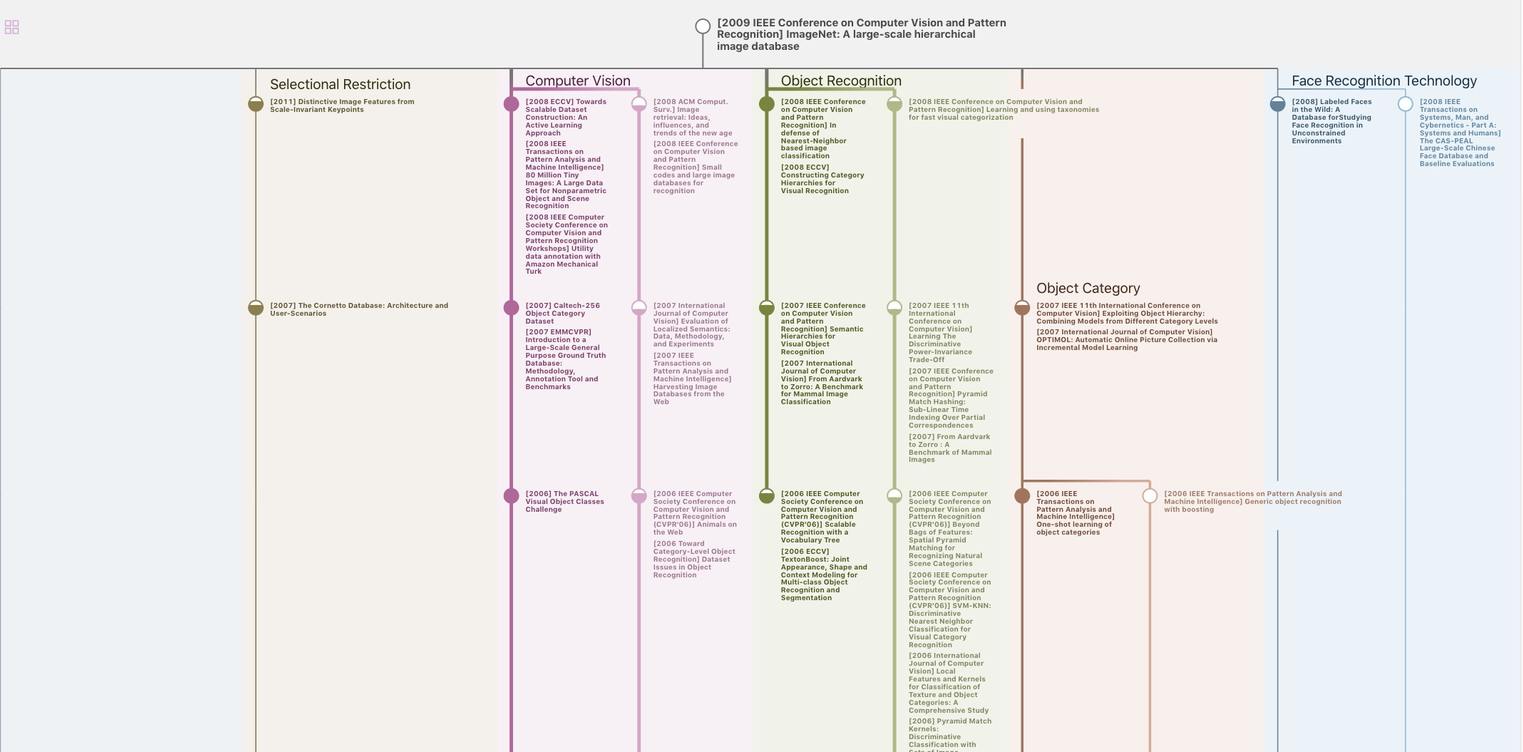
生成溯源树,研究论文发展脉络
Chat Paper
正在生成论文摘要