Randomizing multi-product formulas for improved Hamiltonian simulation.
arXiv (Cornell University)(2021)
摘要
Quantum simulation suggests a path forward for the efficient simulation of problems in condensed-matter physics, quantum chemistry and materials science. While the majority of quantum simulation algorithms is deterministic, a recent surge of ideas has shown that randomization can greatly benefit algorithmic performance. In this work, we introduce a scheme for quantum simulation that unites the advantages of randomized compiling and higher-order multi-product formulas as they are used in linear-combination-of-unitaries (LCU) algorithms. In doing so, we propose a framework of randomized sampling that could prove useful for programmable quantum simulators and present two new algorithms tailored to it. Our framework greatly reduces the circuit depth by circumventing the need for oblivious amplitude amplification required by standard LCU methods, rendering it especially useful for near-term quantum computing. Our algorithms achieve a simulation error that shrinks exponentially with the circuit depth. To corroborate their functioning, we prove rigorous performance bounds as well as the concentration of the randomized sampling procedure. We also discuss examples at hand of a Hamiltonian comprising pairwise anticommuting terms.
更多查看译文
关键词
hamiltonian simulation,multi-product
AI 理解论文
溯源树
样例
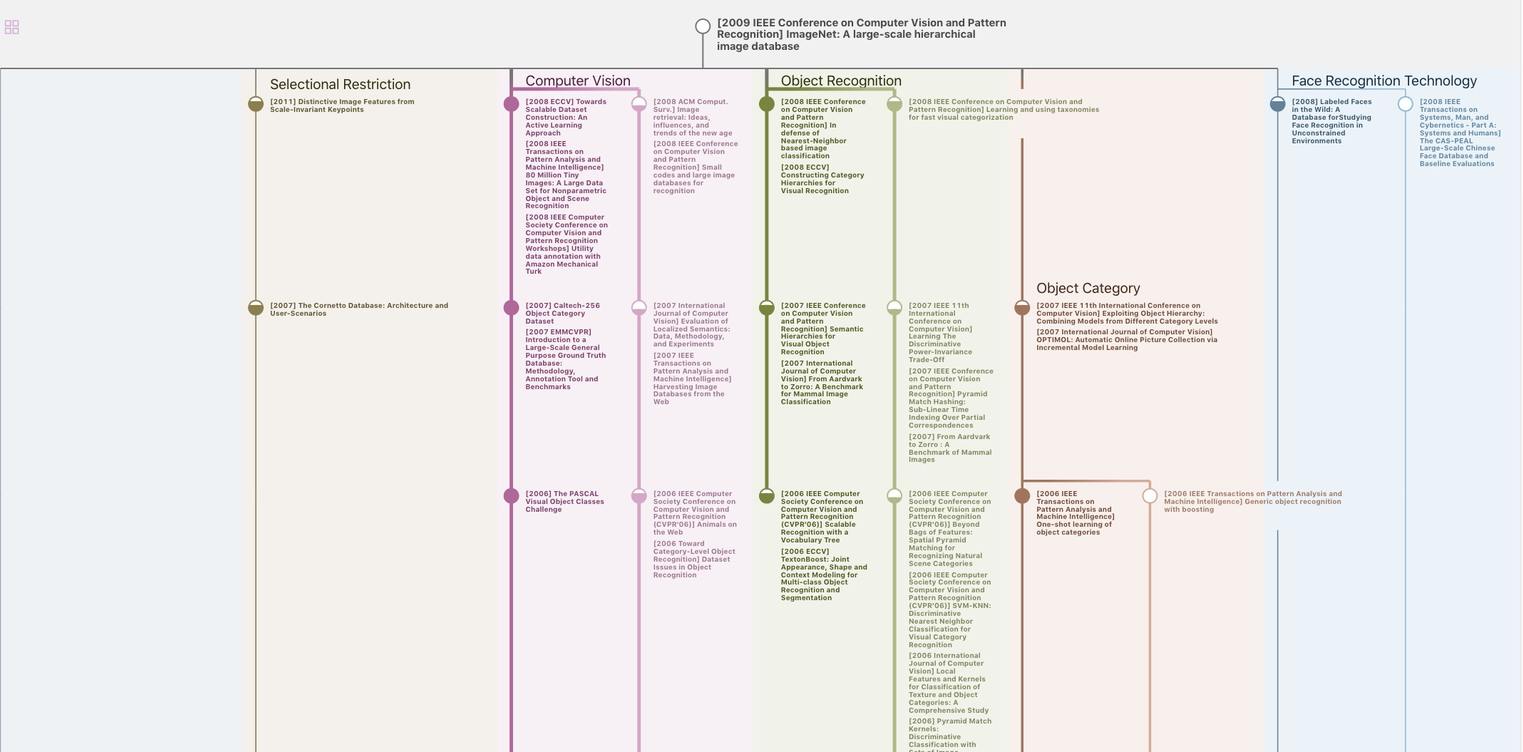
生成溯源树,研究论文发展脉络
Chat Paper
正在生成论文摘要