Evaluating Self and Semi-Supervised Methods for Remote Sensing Segmentation Tasks
arXiv (Cornell University)(2021)
摘要
Self- and semi-supervised machine learning techniques leverage unlabeled data for improving downstream task performance. These methods are especially valuable for remote sensing tasks where producing labeled ground truth datasets can be prohibitively expensive but there is easy access to a wealth of unlabeled imagery. We perform a rigorous evaluation of SimCLR, a self-supervised method, and FixMatch, a semi-supervised method, on three remote sensing tasks: riverbed segmentation, land cover mapping, and flood mapping. We quantify performance improvements on these remote sensing segmentation tasks when additional imagery outside of the original supervised dataset is made available for training. We also design experiments to test the effectiveness of these techniques when the test set is domain shifted to sample different geographic areas compared to the training and validation sets. We find that such techniques significantly improve generalization performance when labeled data is limited and there are geographic domain shifts between the training data and the validation/test data.
更多查看译文
关键词
remote sensing
AI 理解论文
溯源树
样例
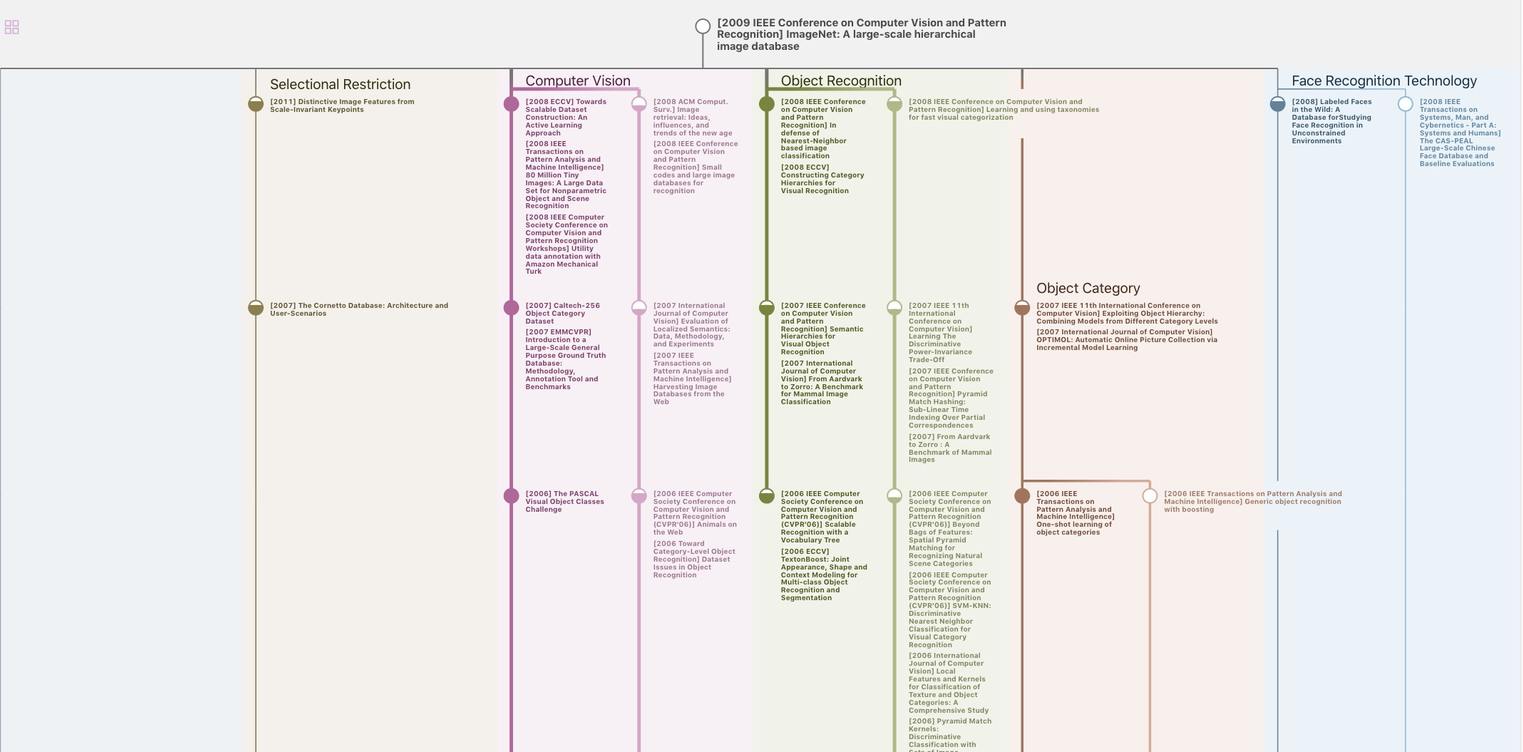
生成溯源树,研究论文发展脉络
Chat Paper
正在生成论文摘要