ImmuNet: A Segmentation-Free Machine Learning Pipeline for Immune Landscape Phenotyping in Tumors by Muliplex Imaging
bioRxiv (Cold Spring Harbor Laboratory)(2021)
摘要
Abstract Tissue specimens taken from primary tumors or metastases contain important information for diagnosis and treat-ment of cancer patients. Multiplex imaging allows in situ visualization of heterogeneous cell populations, such as immune cells, in tissue samples. Most image processing pipelines first segment cell boundaries and then measure marker expression to assign cell phenotypes. In dense tissue environments, this segmentation-first approach can be inaccurate due to segmentation errors or overlapping cells. Here we introduce the machine learning pipeline “ImmuNet” that identifies positions and phenotypes of cells without segmenting them. ImmuNet is easy to train: human annotators only need to click on an immune cell and score its expression of each marker. This approach al-lowed us to annotate 34,458 cells. We show that ImmuNet consistently outperforms a state-of-the-art segmentation-based pipeline for multiplex immunohistochemistry analysis across tissue types, cell types and tissue densities, achieving error rates below 5-10% on challenging detection and phenotyping tasks. We externally validate Im-muNet results by comparing them to flow cytometric measurements from the same tissue. In summary, ImmuNet is an effective, simpler alternative to segmentation-based approaches when only cell positions and phenotypes, but not their shapes, are required for downstream analyses. Thus, ImmuNet helps researchers to analyze multiplex tissue images more easily and accurately.
更多查看译文
关键词
multichannel imaging,solid tumors,segmentation-free,land-scape
AI 理解论文
溯源树
样例
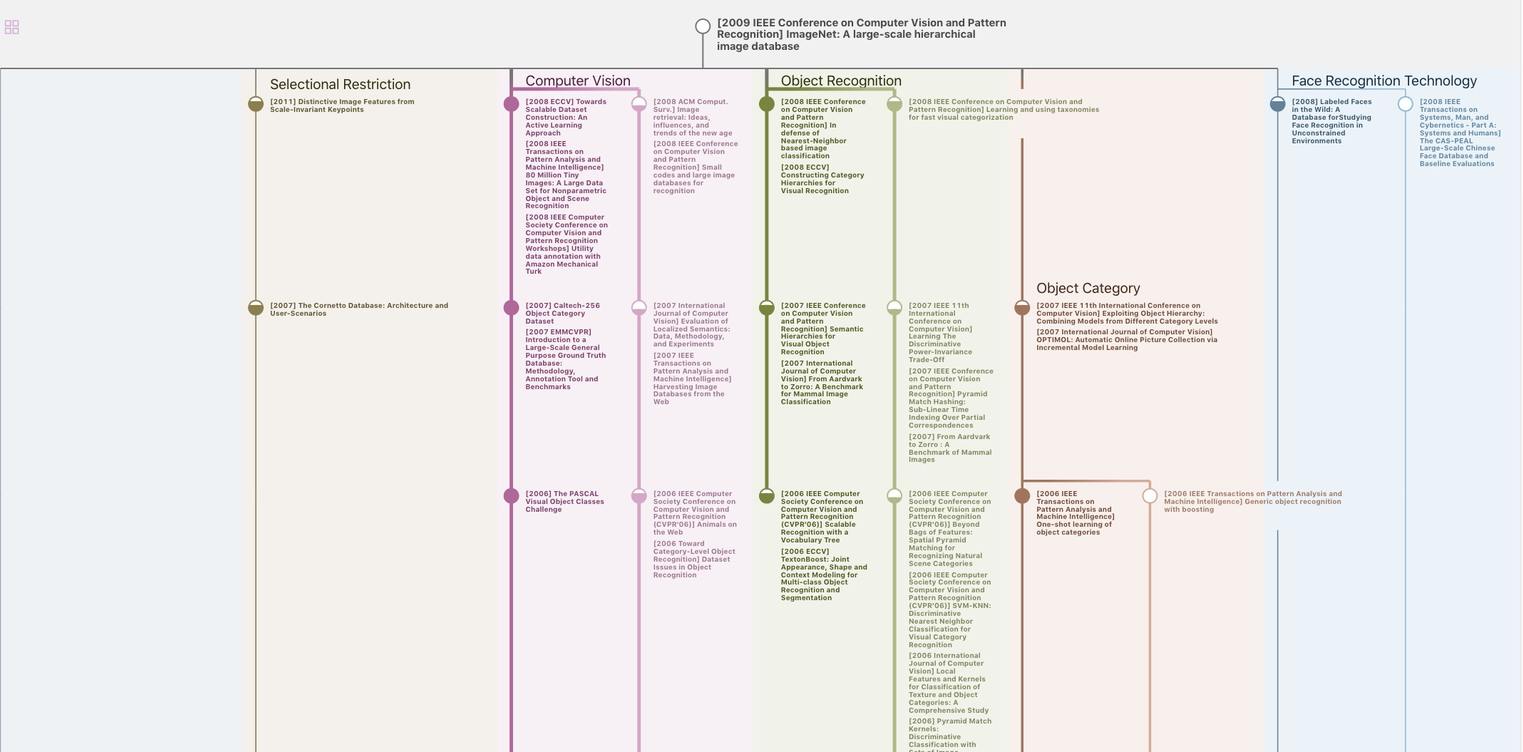
生成溯源树,研究论文发展脉络
Chat Paper
正在生成论文摘要