Performance Analysis and Evaluation of Supervised Machine Learning Algorithms for the Detection of Parkinson’s Disease
crossref(2023)
摘要
Disease detection and prediction have been one of the most frequent uses of machine learning models in the last decade, and Parkinson's disease is no exception. It is common for people in their old age to have one form of disease or another depending on the circumstances in which they find themselves. This study analyzed and evaluated various supervised machine learning models to detect the presence of Parkinson's disease in elderly individuals. An extreme gradient boosting model, along with other models, such as naïve Bayes, random forest, K-nearest neighbor, and support vector machine (SVM), was implemented to determine the best outcome. The extreme gradient boosting (XGBoost) model is a powerful boosting machine learning technique, particularly in terms of speed and accuracy. The results showed that the XGBoost model, which is an optimized version of the other boosting models, is capable of projecting the presence of Parkinson's disease with very high accuracy compared to other algorithms. The implemented classification algorithm extreme gradient boosting method achieved approximately 98 % accuracy, 97.5 % precision, 100.0 % recall, and 98.7% f1-score which are high compared with the results found in the existing literature.
更多查看译文
AI 理解论文
溯源树
样例
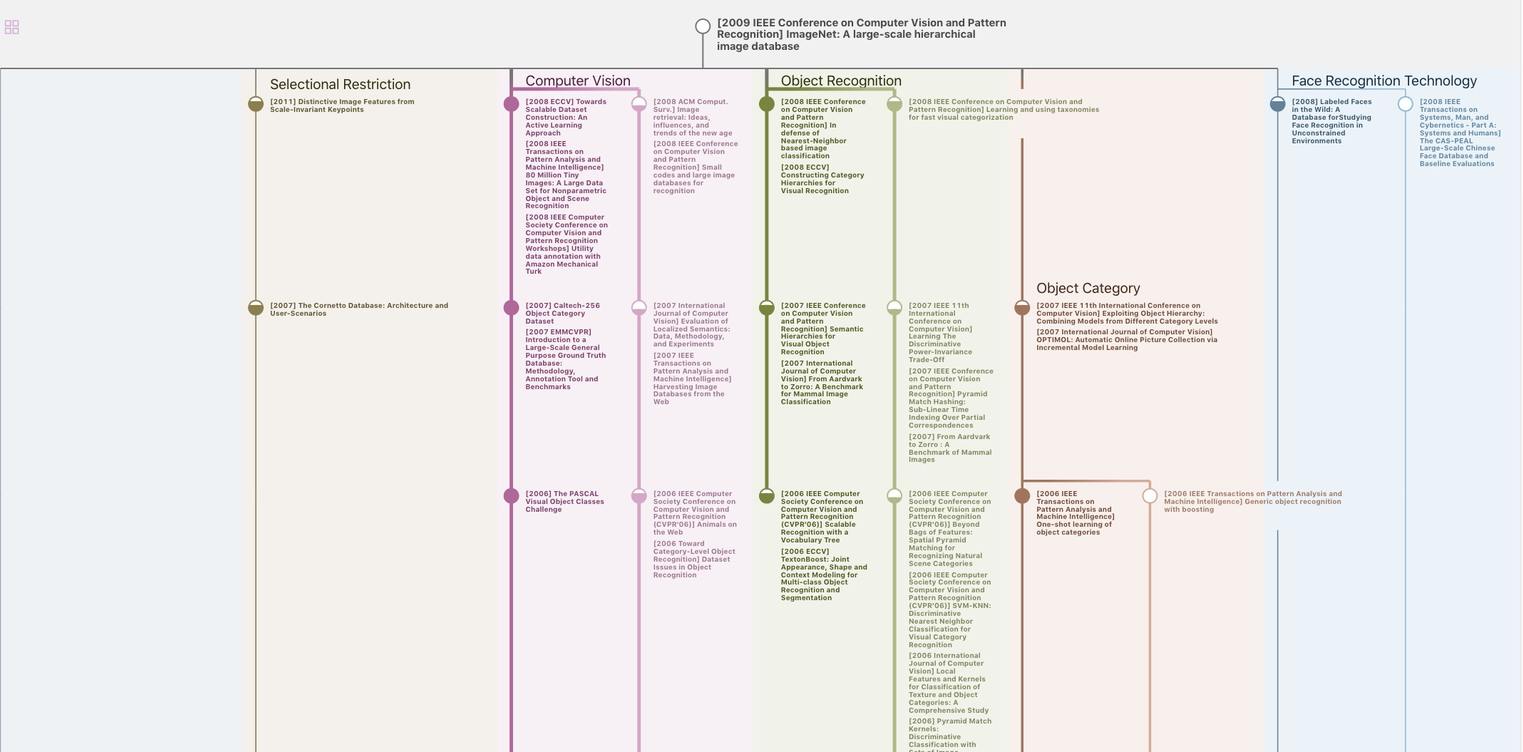
生成溯源树,研究论文发展脉络
Chat Paper
正在生成论文摘要