DedustGAN: Unpaired learning for image dedusting based on Retinex with GANs
EXPERT SYSTEMS WITH APPLICATIONS(2024)
摘要
Image dedusting has gained increasing attention as a preprocessing step for computer vision tasks. Current traditional image dedusting methods rely on a variety of constraints or priors, which are easy to be limited in real complex dusty scenes. Recently, some paired learning CNN methods have been proposed. However, they usually imitate the idea of image dehazing and fail to consider the inherent natural characteristics of dusty images. To bridge this gap, we propose an unpaired learning algorithm for image dedusting based on Retinex with GANs (DedustGAN). Specifically, we first design the DedustGAN with two branches, one branch uses Retinex to obtain one preliminary dedusted image in a physical way, and the other branch uses GANs to obtain another preliminary dedusted image in a learning way. Then, we design a no-reference image fusion strategy to fuse the two preliminary dedusted images for outputting the final dedusted image. The proposed DedustGAN breaks the restriction of existing paired learning methods that require paired images for network training and provide an effective physical dedusting model. Qualitative and quantitative experimental comparisons demonstrate the powerful dedusting ability of the Retinex model and the superiority of DedustGAN over the existing methods. In addition, to validate the strong generalization of the proposed DedustGAN, we further performed experiments on underwater image enhancement and low-light image enhancement tasks. We found that the effectiveness of DedustGAN even outperforms the existing methods in their domains. The code of the proposed DedustGAN is available at https://github.com/MXL696/DedustGAN.
更多查看译文
关键词
Image dedusting,Unpaired learning,Retinex,GANs
AI 理解论文
溯源树
样例
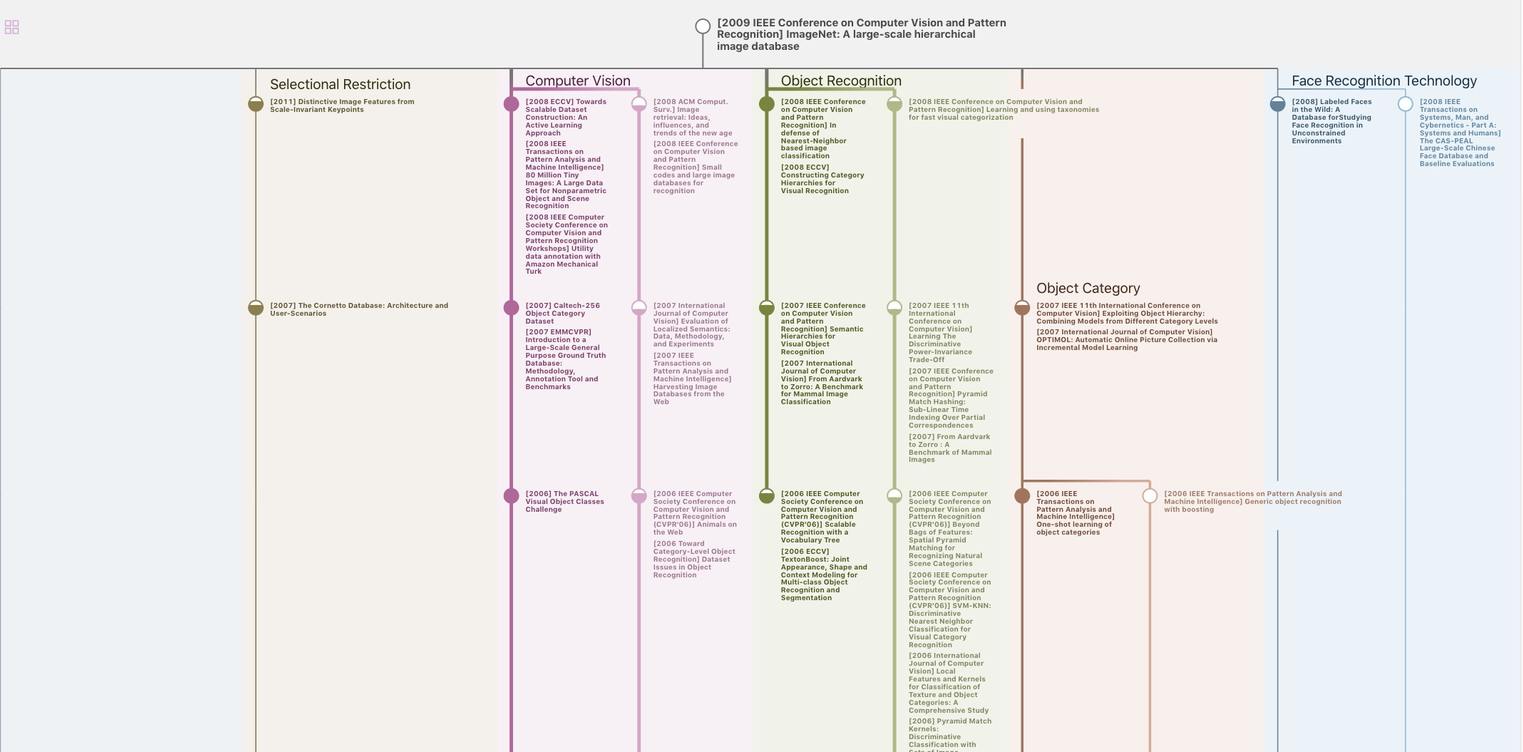
生成溯源树,研究论文发展脉络
Chat Paper
正在生成论文摘要