Physics-Guided Discovery of Highly Nonlinear Parametric Partial Differential Equations
PROCEEDINGS OF THE 29TH ACM SIGKDD CONFERENCE ON KNOWLEDGE DISCOVERY AND DATA MINING, KDD 2023(2023)
摘要
Partial differential equations (PDEs) that fit scientific data can represent physical laws with explainable mechanisms for various mathematically-oriented subjects, such as physics and finance. The data-driven discovery of PDEs from scientific data thrives as a new attempt to model complex phenomena in nature, but the effectiveness of current practice is typically limited by the scarcity of data and the complexity of phenomena. Especially, the discovery of PDEs with highly nonlinear coefficients from low-quality data remains largely under-addressed. To deal with this challenge, we propose a novel physics-guided learning method, which can not only encode observation knowledge such as initial and boundary conditions but also incorporate the basic physical principles and laws to guide the model optimization. We theoretically show that our proposed method strictly reduces the coefficient estimation error of existing baselines, and is also robust against noise. Extensive experiments show that the proposed method is more robust against data noise, and can reduce the estimation error. Moreover, all the PDEs in the experiments are correctly discovered, and for the first time we are able to discover PDEs with highly nonlinear coefficients.
更多查看译文
关键词
Partial differential equations,PDE discovery,highly nonlinear coefficients,physics-guided learning,spatial kernel estimation
AI 理解论文
溯源树
样例
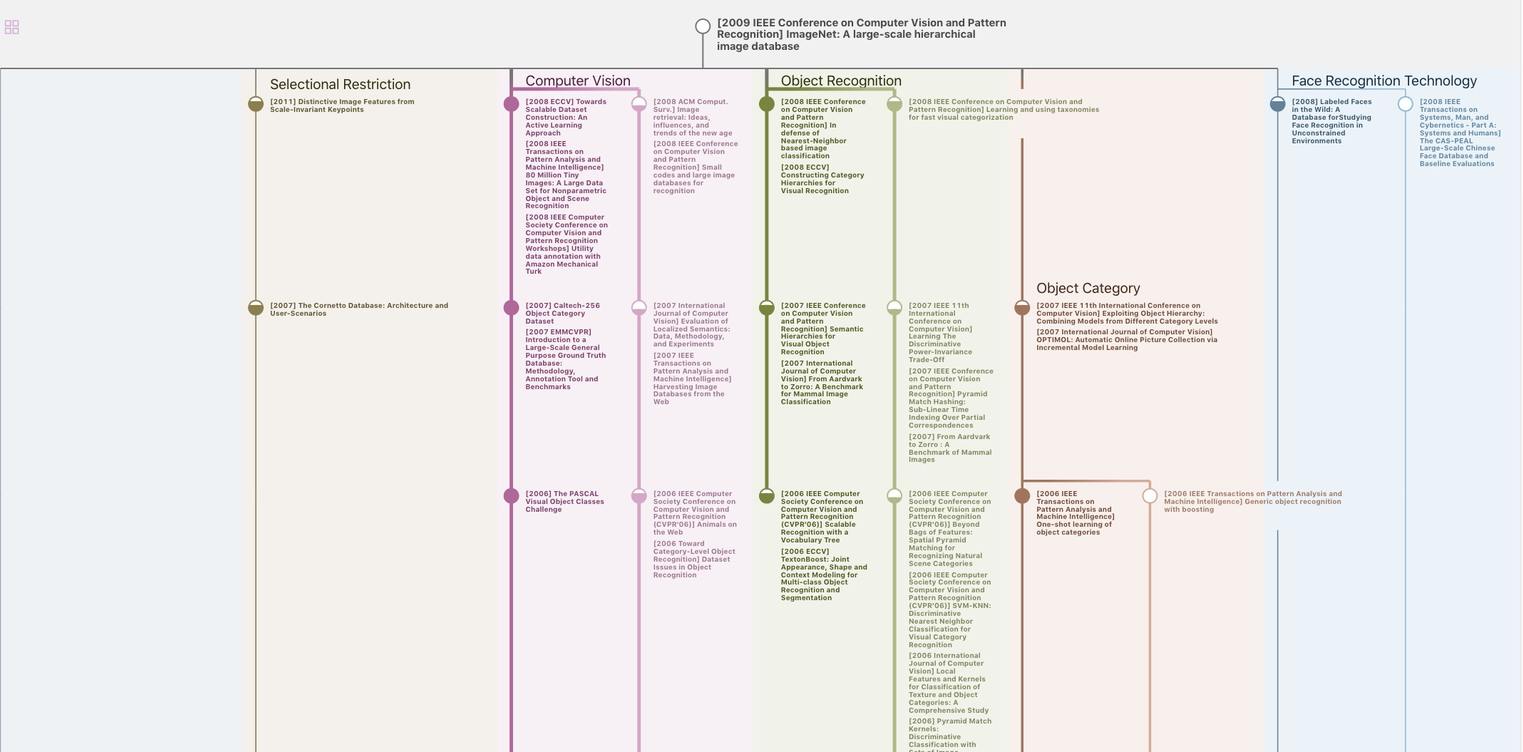
生成溯源树,研究论文发展脉络
Chat Paper
正在生成论文摘要