The Hyperspherical Geometry of Community Detection: Modularity as a Distance.
arXiv (Cornell University)(2021)
摘要
The Louvain algorithm is currently one of the most popular community detection methods. This algorithm finds communities by maximizing a quantity called modularity. In this work, we describe a metric space of clusterings, where clusterings are described by a binary vector indexed by the vertex-pairs. We extend this geometry to a hypersphere and prove that maximizing modularity is equivalent to minimizing the angular distance to some modularity vector over the set of clustering vectors. This equivalence allows us to view the Louvain algorithm as a nearest-neighbor search that approximately minimizes the distance to this modularity vector. By replacing this modularity vector by a different vector, many alternative community detection methods can be obtained. We explore this wider class and compare it to existing modularity-based methods. Our experiments show that these alternatives may outperform modularity-based methods. For example, when communities are large compared to vertex neighborhoods, a vector based on numbers of common neighbors outperforms existing community detection methods. While the focus of the present work is community detection in networks, the proposed methodology can be applied to any clustering problem where pair-wise similarity data is available.
更多查看译文
关键词
community detection,modularity,hyperspherical geometry
AI 理解论文
溯源树
样例
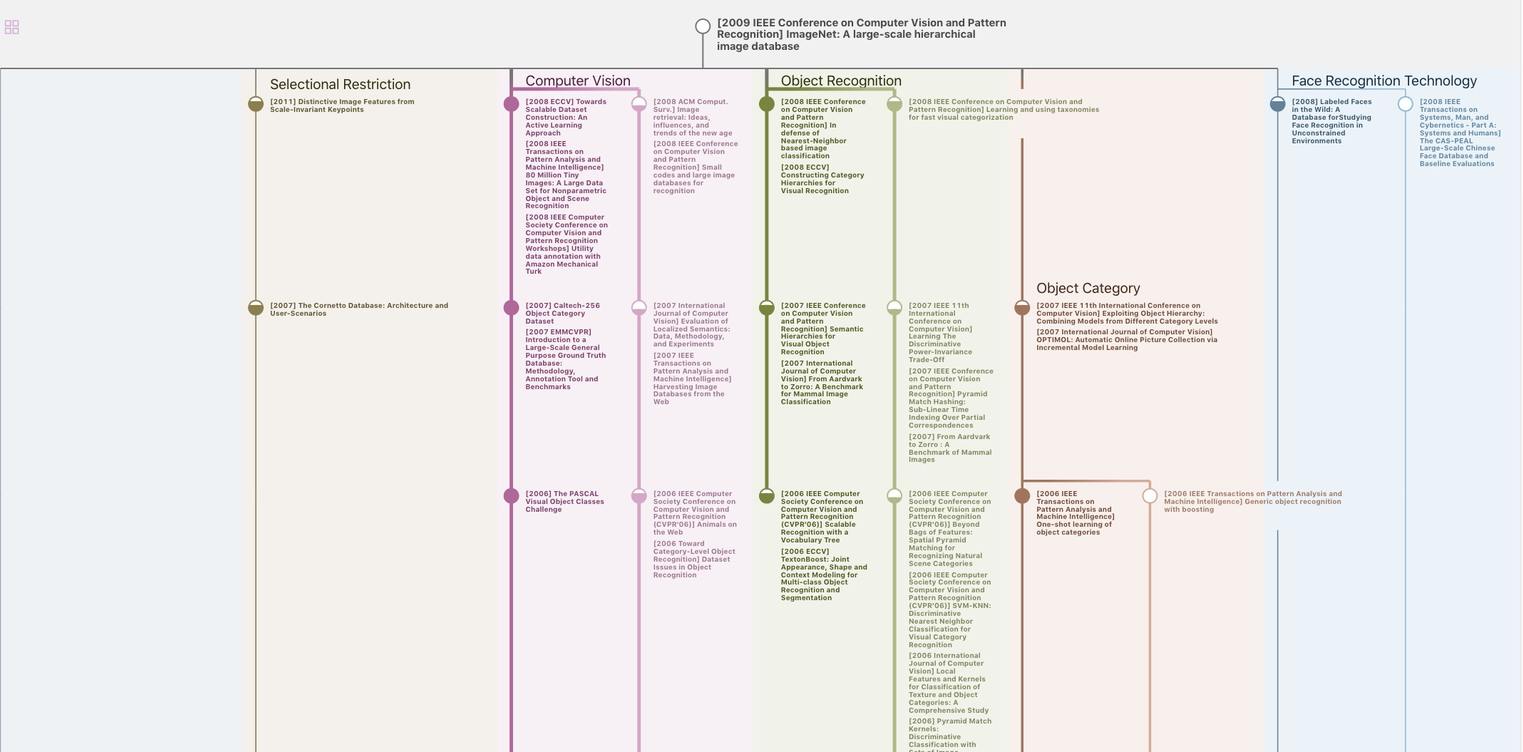
生成溯源树,研究论文发展脉络
Chat Paper
正在生成论文摘要