Runtime Monitoring for Markov Decision Processes.
arXiv (Cornell University)(2021)
摘要
We investigate the problem of monitoring partially observable systems with nondeterministic and probabilistic dynamics. In such systems, every state may be associated with a risk, e.g., the probability of an imminent crash. During runtime, we obtain partial information about the system state in form of observations. The monitor uses this information to estimate the risk of the (unobservable) current system state. Our results are threefold. First, we show that extensions of state estimation approaches do not scale due the combination of nondeterminism and probabilities. While convex hull algorithms improve the practical runtime, they do not prevent an exponential memory blowup. Second, we present a tractable algorithm based on model checking conditional reachability probabilities. Third, we provide prototypical implementations and manifest the applicability of our algorithms to a range of benchmarks. The results highlight the possibilities and boundaries of our novel algorithms.
更多查看译文
关键词
markov decision processes,monitoring
AI 理解论文
溯源树
样例
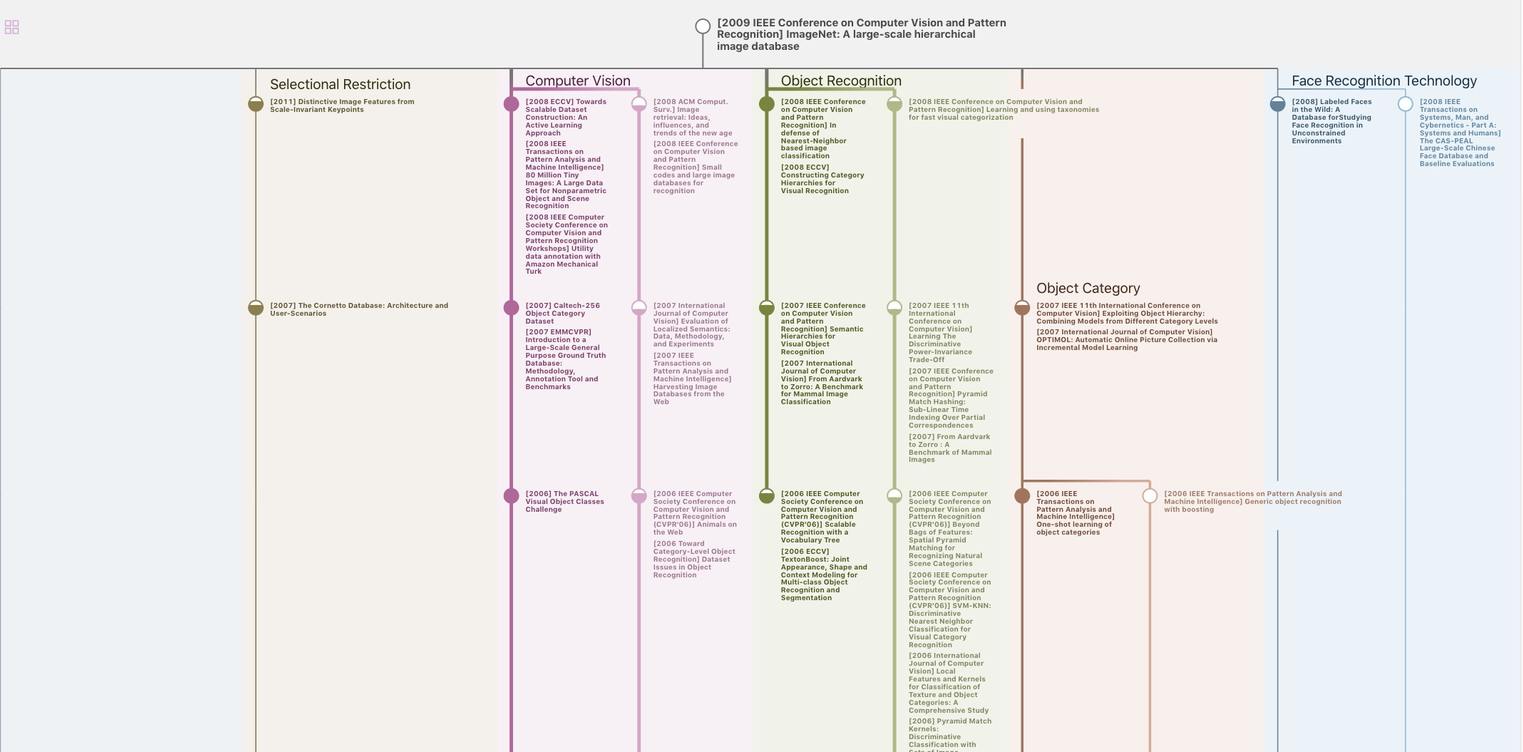
生成溯源树,研究论文发展脉络
Chat Paper
正在生成论文摘要