A graph-embedded topic model enables characterization of diverse pain phenotypes among UK Biobank individuals
bioRxiv (Cold Spring Harbor Laboratory)(2022)
摘要
SUMMARY Large biobank repositories of clinical conditions and medications data open opportunities to investigate the phenotypic disease network. To enable systematic investigation of entire structured phenomes, we present graph embedded topic model (GETM). Our contributions are two folds in terms of method and applications. On the methodology side, we offer two main contributions in GETM. First, to aid topic inference, we integrate existing biomedical knowledge graph information in the form of pre-trained graph embedding into the embedded topic model. Second, leveraging deep learning techniques, we developed a variational autoencoder framework to infer patient phenotypic mixture by modeling multi-modal discrete patient medical records. In particular, for interpretability, we use a linear decoder to simultaneously infer the bi-modal distributions of the disease conditions and medications. On the application side, we applied GETM to UK Biobank (UKB) self-reported clinical phenotype data, which contains 443 self-reported medical conditions and 802 self-reported medications for 457,461 individuals. Compared to existing methods, GETM demonstrates overall superior performance in imputing missing conditions and medications. Here, we focused on characterizing pain phenotypes recorded in the questionnaire of the UKB individuals. GETM accurately predicts the status of chronic musculoskeletal (CMK) pain, chronic pain by body-site, and non-specific chronic pain using past conditions and medications. Our analyses revealed not only the known pain-related topics but also the surprising predominance of medications and conditions in the cardiovascular category among the most predictive topics across chronic pain phenotypes.
更多查看译文
关键词
diverse pain phenotypes,topic model,uk biobank individuals,graph-embedded
AI 理解论文
溯源树
样例
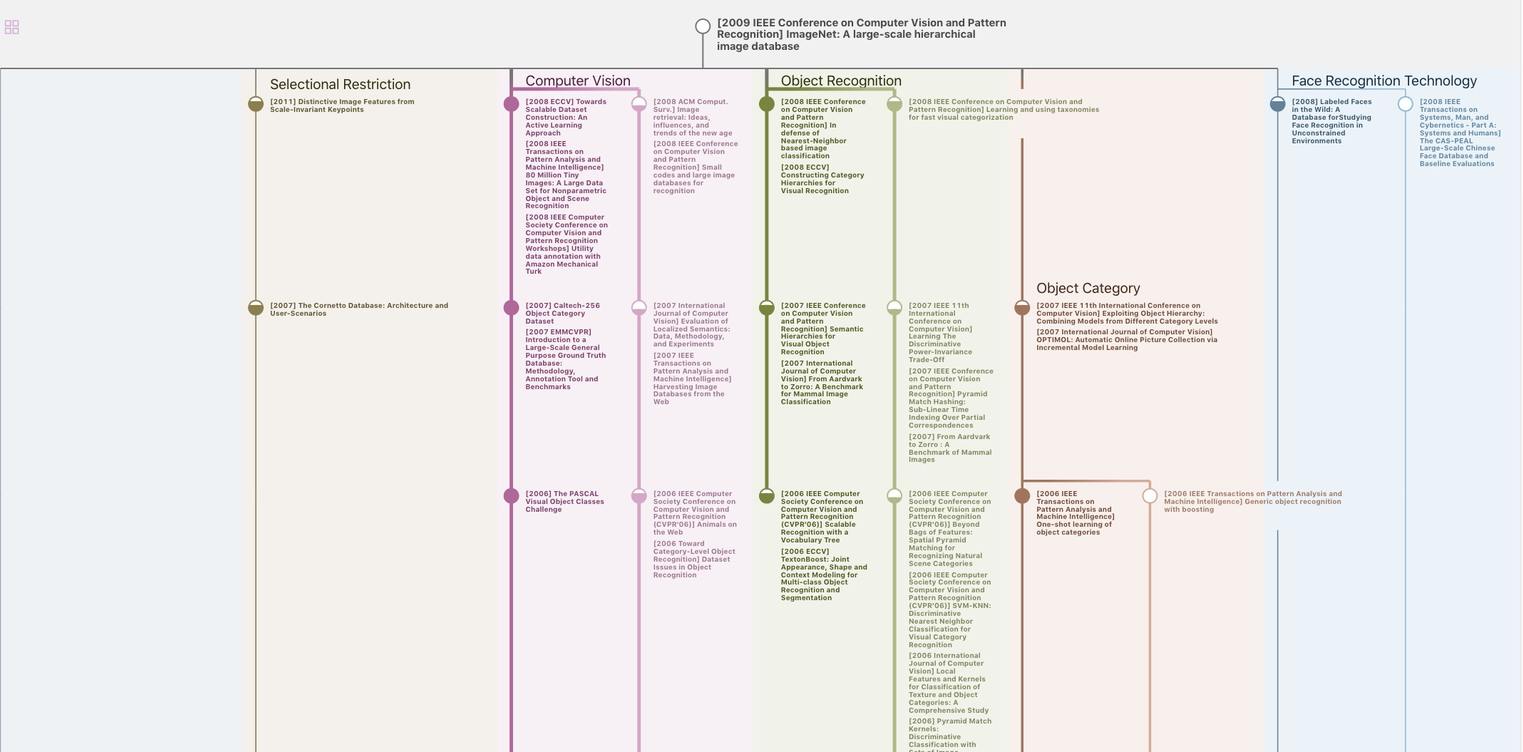
生成溯源树,研究论文发展脉络
Chat Paper
正在生成论文摘要