3D single-cell shape analysis using geometric deep learning
bioRxiv (Cold Spring Harbor Laboratory)(2022)
摘要
A bstract Aberrations in 3D cell morphogenesis are linked to diseases such as cancer. Yet there is little systems-level understanding of cell shape determination in 3D, largely because there is a paucity of data-driven methods to quantify and describe 3D cell shapes. We have addressed this need using unsupervised geometric deep learning to learn shape representations of over 95,000 melanoma cells imaged by 3D high-throughput light-sheet microscopy. We used a dynamic graph convolutional foldingnet autoencoder with improved deep embedded clustering to simultaneously learn lower-dimensional representations and classes of 3D cell shapes. We describe a landscape of 3D cell morphology using deep learning-derived 3D quantitative morphological signatures (3DQMS) across different substrate geometries, following treatment by different clinically relevant small molecules and systematic gene depletion in high-throughput. By data integration, we predict modes of action for different small molecules providing mechanistic insights and blueprints for biological re-engineering. Finally, we provide explainability and interpretability for deep learning models.
更多查看译文
关键词
geometric deep learning,shape,3d,single-cell
AI 理解论文
溯源树
样例
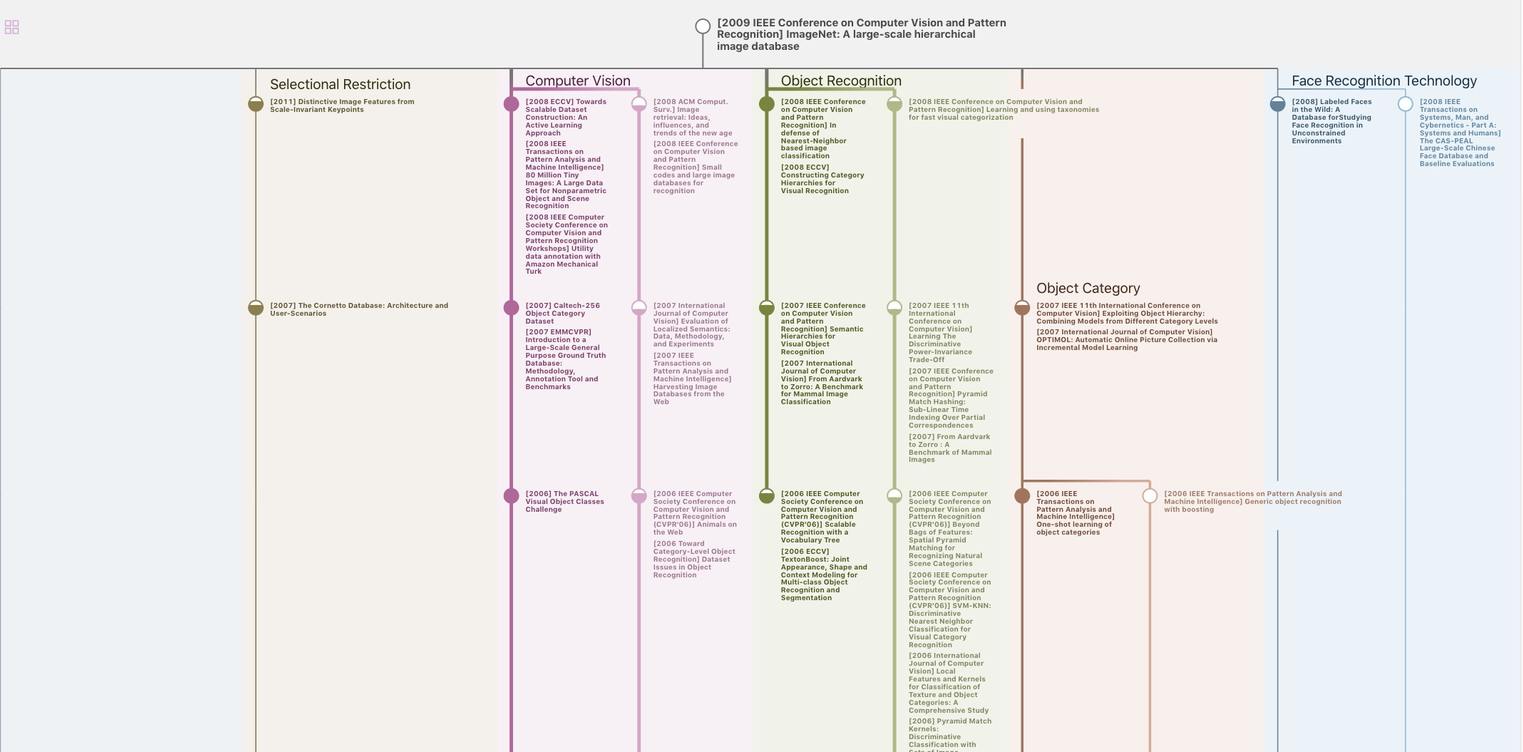
生成溯源树,研究论文发展脉络
Chat Paper
正在生成论文摘要