Abstract 10054: Super Resolution Deep Learning Reconstruction for Detection of In-Stent Restenosis
Circulation(2022)
摘要
Introduction: Evaluation of in-stent-restenosis (ISR), especially of small stents, remains a challenge for computed tomography (CT) angiography. Hypothesis: To quantitatively define stent strut thickness and lumen vessel diameter at the stent site by deep learning reconstruction and compare this with conventional reconstruction strategies. Methods: We employed ultra-high-resolution CT (URCT) data to train a super resolution neural network (Precise IQ engine, or PIQE) and apply the network to current standard resolution CT (Forward projected model-based Iterative Reconstruction SoluTion, FIRST). We examined 166 stents in 85 consecutive patients who underwent CT and invasive coronary angiography (ICA) within three months of each other from 2019-2021 after percutaneous coronary intervention with coronary stent placement. The diagnostic accuracy for the presence of ISR was defined as percent diameter stenosis > 50% on ICA. All images were reconstructed with PIQE and FIRST and assessed by two blinded cardiovascular imagers. Results: Compared to FIRST, the full width at half maximum of the lumen was larger, and that of the strut was smaller in PIQE. Image quality score was significantly better in PIQE than in FIRST (4.2±1.1 vs. 2.7±1.2, P <0.01). The sensitivity, specificity, PPV, NPV, and accuracy for detection of ISR in PIQE were better than the FIRST (86.1, 89.5, 50.0, 98.1, and 89.2 vs. 80.6, 77.4, 30.2, 97.0, and 77.7, respectively); the differences were particularly pronounced in a group with < 3.0 mm stent diameter. Conclusions: PIQE provides superior image quality and diagnostic accuracy for ISR, even in stents with < 3.0 mm diameter.
更多查看译文
关键词
restenosis,deep learning,in-stent
AI 理解论文
溯源树
样例
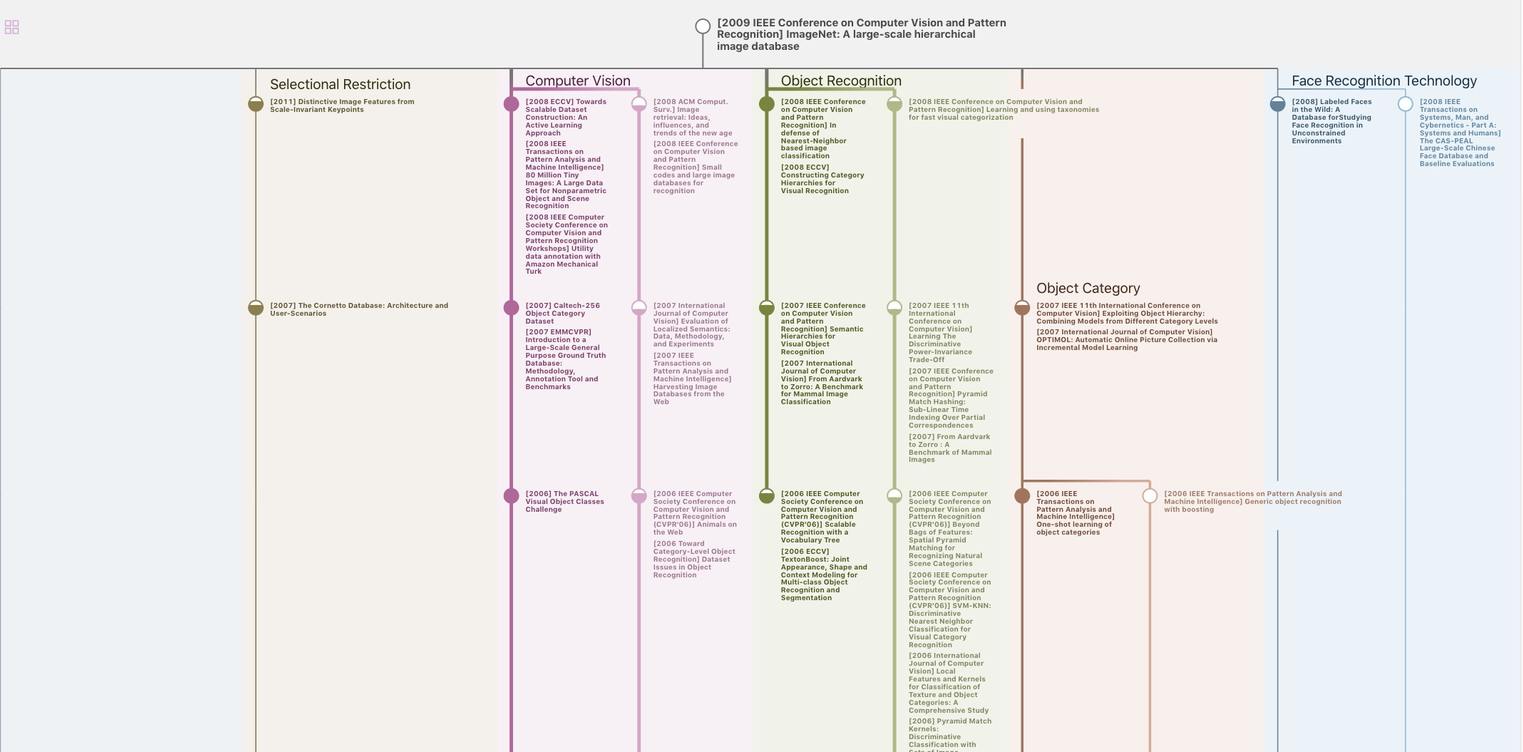
生成溯源树,研究论文发展脉络
Chat Paper
正在生成论文摘要