OpTopNET: A Learning Optimal Topology Synthesizer for Ad-hoc Robot Networks
arXiv (Cornell University)(2022)
摘要
In this paper, we synthesize a machine-learning stacked ensemble model a vector of which predicts the optimal topology of a robot network. This problem is technically a multi-task classification problem. However, we divide it into a class of multi-class classification problems that can be more efficiently solved. For this purpose, we first compose an algorithm to create ground-truth topologies associated with various configurations of a robot network. This algorithm incorporates a complex collection of nonlinear optimality criteria that our learning model successfully manages to learn. Then, we propose a stacked ensemble model whose output is the topology prediction for the particular robot associated with it. Each stacked ensemble instance constitutes three low-level estimators whose outputs will be aggregated by a high-level boosting blender. The results of the simulations, applying our model to a network of 10 robots, represents over %80 accuracy in the prediction of optimal topologies corresponding to various configurations of this complex optimal topology learning problem.
更多查看译文
关键词
learning optimal topology synthesizer,optopnet,networks,robot
AI 理解论文
溯源树
样例
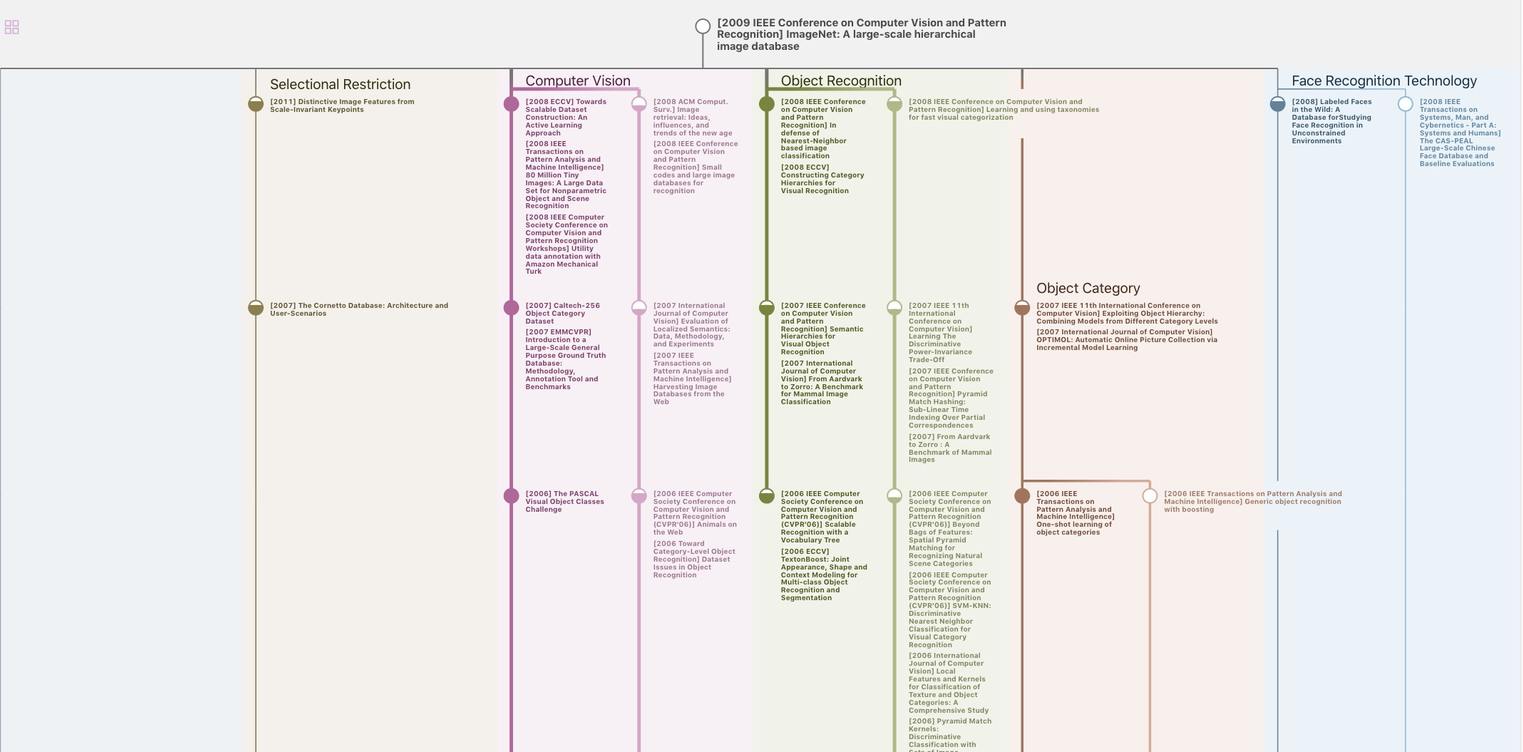
生成溯源树,研究论文发展脉络
Chat Paper
正在生成论文摘要