Structure-Aware Dialogue Modeling Methods for Conversational Semantic Role Labeling
IEEE-ACM TRANSACTIONS ON AUDIO SPEECH AND LANGUAGE PROCESSING(2024)
摘要
Conversational semantic role labeling (CSRL) is believed to be a crucial step toward dialogue understanding. By incorporating the CSRL information into the conversational models, previous work (Xu et al., 2021) has confirmed the usefulness of CSRL to downstream conversation-based tasks, including multi-turn dialogue rewriting and multi-turn dialogue response generation. However, (Xu et al., 2021) found that the quality of the extracted CSRL structures would consequently affect the performance of downstream dialogue tasks while the performance of existing CSRL models is still unsatisfactory. There are two major problems in existing CSRL models to handle predicate-aware and conversational structural information. First, they ignore the fact that explicitly correlating the predicate and the context utterances could help the model better identify the arguments. Secondly, these models do not encode some vital conversational structural information, such as the speaker information which is necessary for modeling inter-speaker dependency. In this paper, we model the conversational structure-aware features based on three components: 1) the predicate-aware module which aims to capture rich correlations between the predicate and utterances; 2) a speaker-aware graph network which explicitly encodes the speaker-dependent information; 3) a novel structure-aware dialogue modeling method for the model warm-up. Experimental results on benchmark datasets show that our model significantly outperforms the baselines. We also examine the efficiency of our model and its effectiveness in low-resource scenarios. We find that our model can achieve better performance with less training time and training data than the existing models. In addition, further improvements are observed when applying the CSRL information extracted by our model into downstream dialogue tasks, which consistently indicates the superiority of our model.
更多查看译文
关键词
Context modeling,Task analysis,Semantics,Labeling,Speech processing,Biological system modeling,Training,Conversational semantic role labeling,dialogue modeling,graph neural networks
AI 理解论文
溯源树
样例
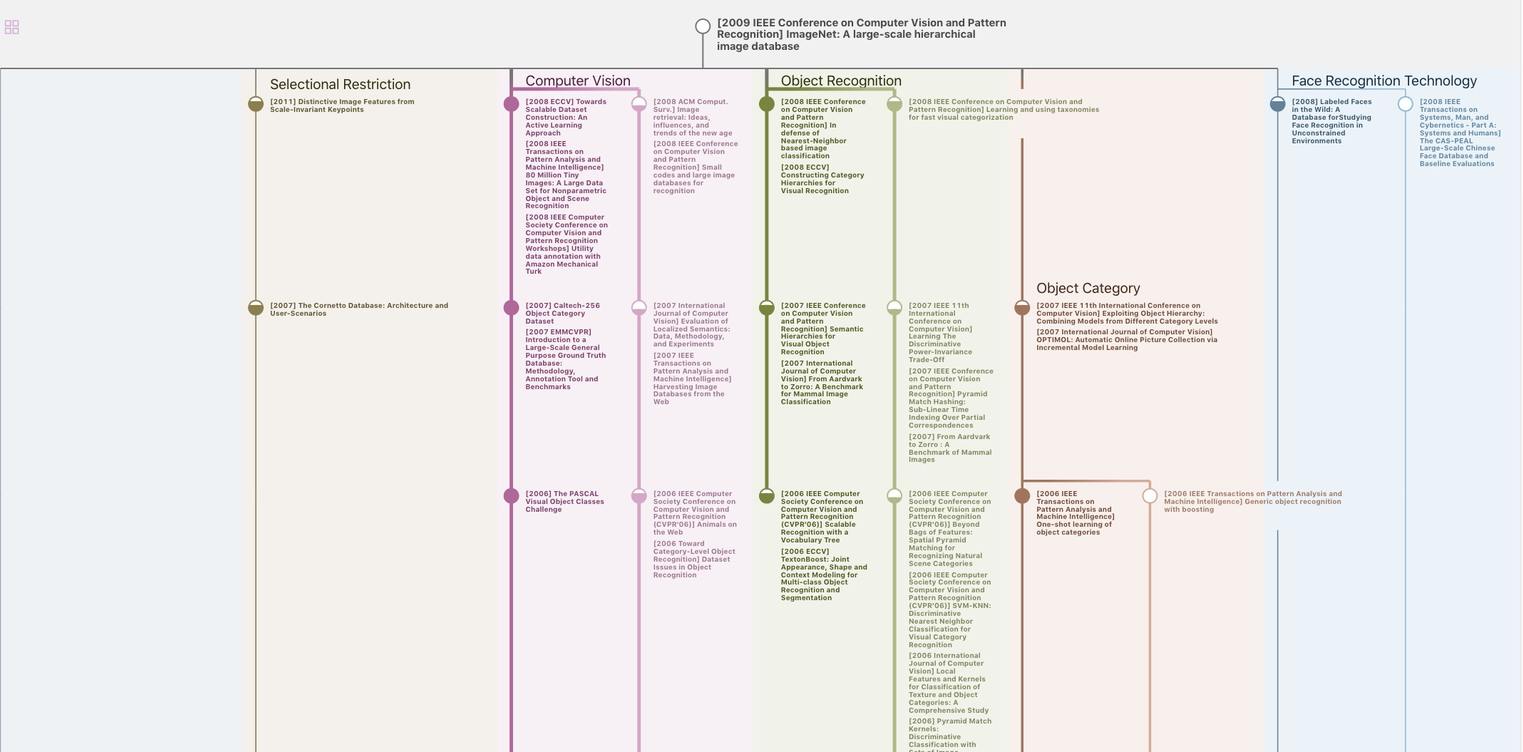
生成溯源树,研究论文发展脉络
Chat Paper
正在生成论文摘要