Prediction of Forest Fire Risk for Artillery Military Training using Weighted Support Vector Machine for Imbalanced Data
Journal of Classification(2024)
摘要
Since the 1953 truce, the Republic of Korea Army (ROKA) has regularly conducted artillery training, posing a risk of wildfires — a threat to both the environment and the public perception of national defense. To assess this risk and aid decision-making within the ROKA, we built a predictive model of wildfires triggered by artillery training. To this end, we combined the ROKA dataset with meteorological database. Given the infrequent occurrence of wildfires (imbalance ratio ≈ 1:24 in our dataset), achieving balanced detection of wildfire occurrences and non-occurrences is challenging. Our approach combines a weighted support vector machine with a Gaussian mixture-based oversampling, effectively penalizing misclassification of the wildfires. Applied to our dataset, our method outperforms traditional algorithms (G-mean=0.864, sensitivity=0.956, specificity= 0.781), indicating balanced detection. This study not only helps reduce wildfires during artillery trainings but also provides a practical wildfire prediction method for similar climates worldwide.
更多查看译文
关键词
Forest fire,Imbalanced data,Risk prediction,Weighted support vector machine
AI 理解论文
溯源树
样例
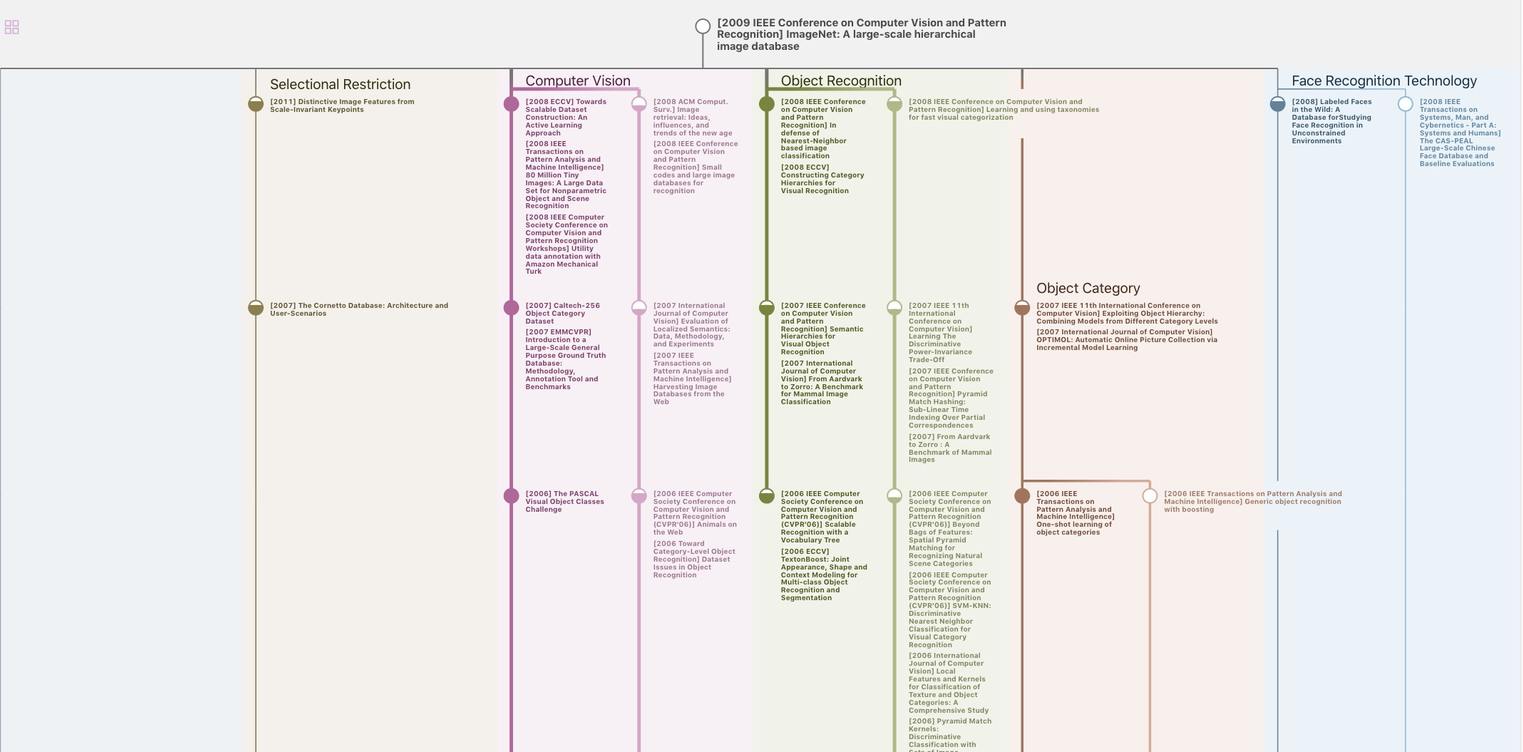
生成溯源树,研究论文发展脉络
Chat Paper
正在生成论文摘要