Deep-Learning Markerless Tracking of Infant General Movements using Standard Video Recordings
2023 45TH ANNUAL INTERNATIONAL CONFERENCE OF THE IEEE ENGINEERING IN MEDICINE & BIOLOGY SOCIETY, EMBC(2023)
摘要
Monitoring spontaneous General Movements (GM) of infants 6-20 weeks post-term age is a reliable tool to assess the quality of neurodevelopment in early infancy. Abnormal or absent GMs are reliable prognostic indicators of whether an infant is at risk of developing neurological impairments and disorders such as cerebral palsy (CP). Therapeutic interventions are most effective at improving neuromuscular outcomes if administered in early infancy. Current clinical protocols require trained assessors to rate videos of infant movements, a time-intensive task. This work proposes a simple, inexpensive, and broadly applicable markerless pose-estimation approach for automatic infant movement tracking using conventional video recordings from handheld devices (e.g., tablets and mobile phones). We leverage the enhanced capabilities of deep-learning technology in image processing to identify 12 anatomical locations (3 per limb) in each video frame, tracking a baby's natural movement throughout the recordings. We validate the capability of resnet152 and a mobile-net-v2-1 to identify body-parts in unseen frames from a full-term male infant, using a novel automatic unsupervised approach that fuses likelihood outputs of a Kalman filter and the deep-nets. Both deep-net models were found to perform very well in the identification of anatomical locations in the unseen data with high average Percentage of Correct Keypoints (aPCK) performances of >99.65% across all locations.
更多查看译文
AI 理解论文
溯源树
样例
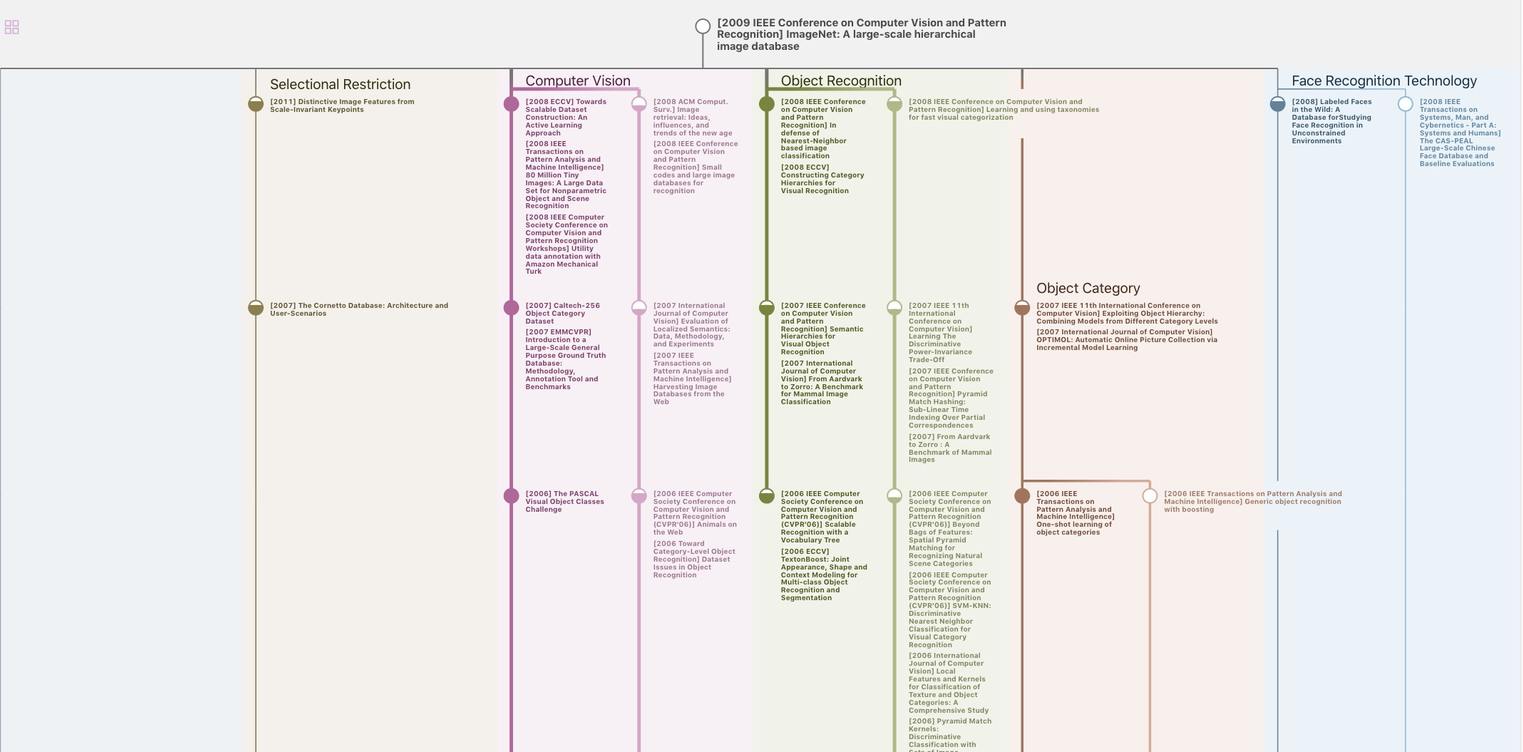
生成溯源树,研究论文发展脉络
Chat Paper
正在生成论文摘要