Underwater Signal Detection Using Non-Parametric Classifiers
OCEANS 2023 - MTS/IEEE U.S. Gulf Coast(2023)
摘要
Detecting signals of interest amidst additive noise and reverberation is an important problem in sonar. Model-based parametric classifiers have been widely used to discriminate between alternative and null hypotheses. However, underwater signals exhibit dynamic and non-stationary characteristics, necessitating the use of complex and often infeasible models to accurately replicate them. While model-free data-driven algorithms offer an alternative, existing methods do not fully exploit the statistical properties of the data and may converge only to local solutions. To address these limitations, we introduce a novel model-free and non-parametric classifier, the Cumulant Generating Function Classifier (CGFC), designed to harness the underlying statistical properties of available data sets and employ convex optimization to converge to global solutions. We apply the CGFC to underwater detection problems and conduct a comparative analysis against convolutional neural networks (CNNs). The results demonstrate the CGFC's superiority over CNNs, presenting it as a promising alternative for effective detection and classification tasks in sonar applications.
更多查看译文
关键词
Cumulative generating function classifier,detection,neural networks,nonparametric classifier,sonar
AI 理解论文
溯源树
样例
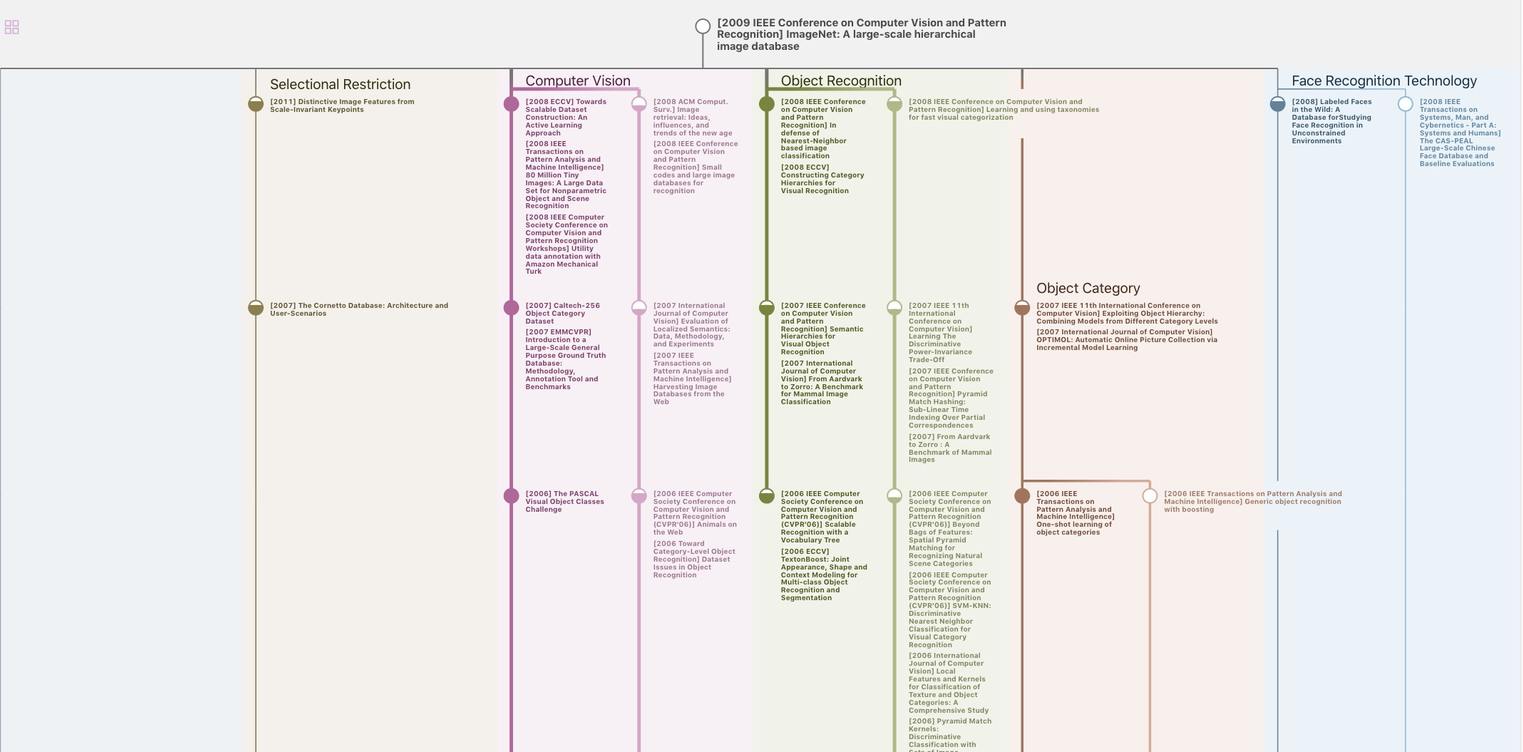
生成溯源树,研究论文发展脉络
Chat Paper
正在生成论文摘要