A Data-Driven Approach for Modeling Unknown Multi-Scale Systems.
2023 IEEE International Conference on Autonomic Computing and Self-Organizing Systems Companion (ACSOS-C)(2023)
摘要
Complex adaptive systems often organize via multiple abstraction levels, or ‘scales’, interconnected by feedback loops. This enables adaptation and survival in changing environments, while managing complexity with limited resources. For an external observer unaware of such multi-scale structure, modeling an unknown system may be a complicated endeavor. This position paper proposes a data-driven approach for addressing this issue. It generates multi-scale models from incomplete monitoring data, capitalizing on the behavioral regularities that stem from its feedback loops. It also defines the appropriate language elements for expressing these multi-scale models. We validate our approach on data obtained from a theoretical multi-scale system: a holonic cellular automata (HCA) simulator. Results show that the proposed approach can identify the HCA's three abstraction levels and main modeling concepts. This is an encouraging first step towards establishing automatic methods for multi-scale model discovery from partial observations.
更多查看译文
关键词
knowledge abstraction,multi-scale feedbacks,dynamic meta-models,data-driven modeling
AI 理解论文
溯源树
样例
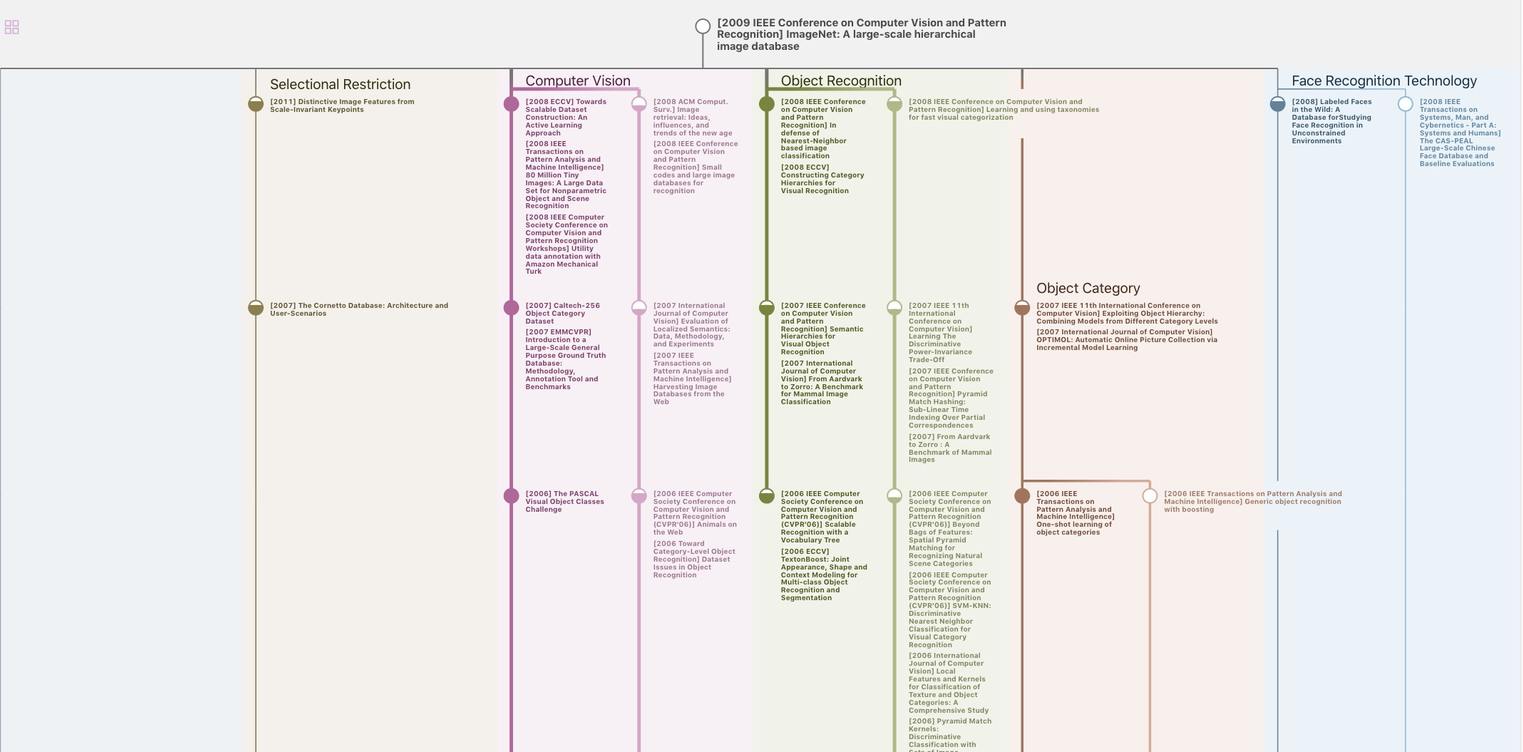
生成溯源树,研究论文发展脉络
Chat Paper
正在生成论文摘要