On the Mitigation of Read Disturbances in Neuromorphic Inference Hardware
arXiv (Cornell University)(2022)
摘要
Non-Volatile Memory (NVM) cells are used in neuromorphic hardware to store model parameters, which are programmed as resistance states. NVMs suffer from the read disturb issue, where the programmed resistance state drifts upon repeated access of a cell during inference. Resistance drifts can lower the inference accuracy. To address this, it is necessary to periodically reprogram model parameters (a high overhead operation). We study read disturb failures of an NVM cell. Our analysis show both a strong dependency on model characteristics such as synaptic activation and criticality, and on the voltage used to read resistance states during inference. We propose a system software framework to incorporate such dependencies in programming model parameters on NVM cells of a neuromorphic hardware. Our framework consists of a convex optimization formulation which aims to implement synaptic weights that have more activations and are critical, i.e., those that have high impact on accuracy on NVM cells that are exposed to lower voltages during inference. In this way, we increase the time interval between two consecutive reprogramming of model parameters. We evaluate our system software with many emerging inference models on a neuromorphic hardware simulator and show a significant reduction in the system overhead.
更多查看译文
关键词
neuromorphic inference
AI 理解论文
溯源树
样例
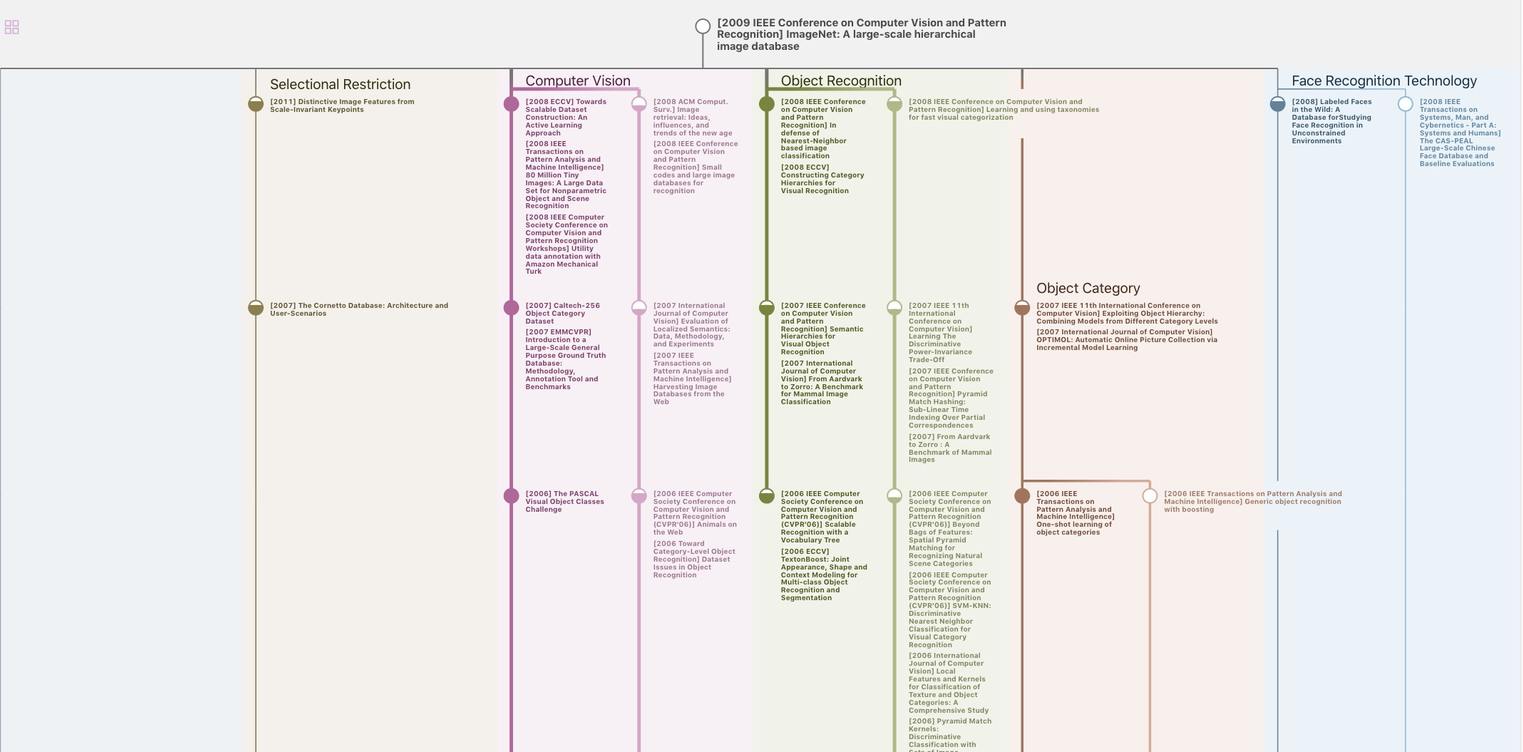
生成溯源树,研究论文发展脉络
Chat Paper
正在生成论文摘要