Deep Learning Based Electrical Load Forecasting Using Temporal Fusion Transformer and Trend-Seasonal Decomposition
2023 31st International Conference on Electrical Engineering (ICEE)(2023)
摘要
Secure and consistent electrical power supply is promised by smart grids. Moreover, high-tech monitoring and metering instruments provoked smart grids into more self-controlled and automated systems. Therefore, load forecasting with high accuracy is fundamentally required at the individual and aggregated level of the power system for planning and operation studies. Deep neural networks have proved their capability of time-series forecasting in different fields. As well, Transformer architecture was a revolution in deep neural networks with outstanding performance in different fields. So far, Temporal Fusion Transformer (TFT) is one of the leading neural networks based on the Transformer concept. The TFT model is designed particularly for stochastic time-series forecasting, which reveals high-accuracy forecasting results. In this paper, TFT is employed as the backbone neural network architecture for electrical load forecasting. Furthermore, a trend-seasonal decomposition method is utilized based on the moving-average concept to break down the original time series into the trend and seasonal components. Trend-seasonal decomposition can provide a bright view of time series over time. The proposed model is tested on Iran's historical load data to validate the performance of mid-term load forecasting. However, the proposed method is robust for any time horizon. Results demonstrate notable improvements in the forecasting accuracy of the proposed model compared to the original TFT.
更多查看译文
关键词
Deep Learning,Deep Neural Networks,Load Forecasting,Temporal Fusion Transformer,Time-series Decomposition
AI 理解论文
溯源树
样例
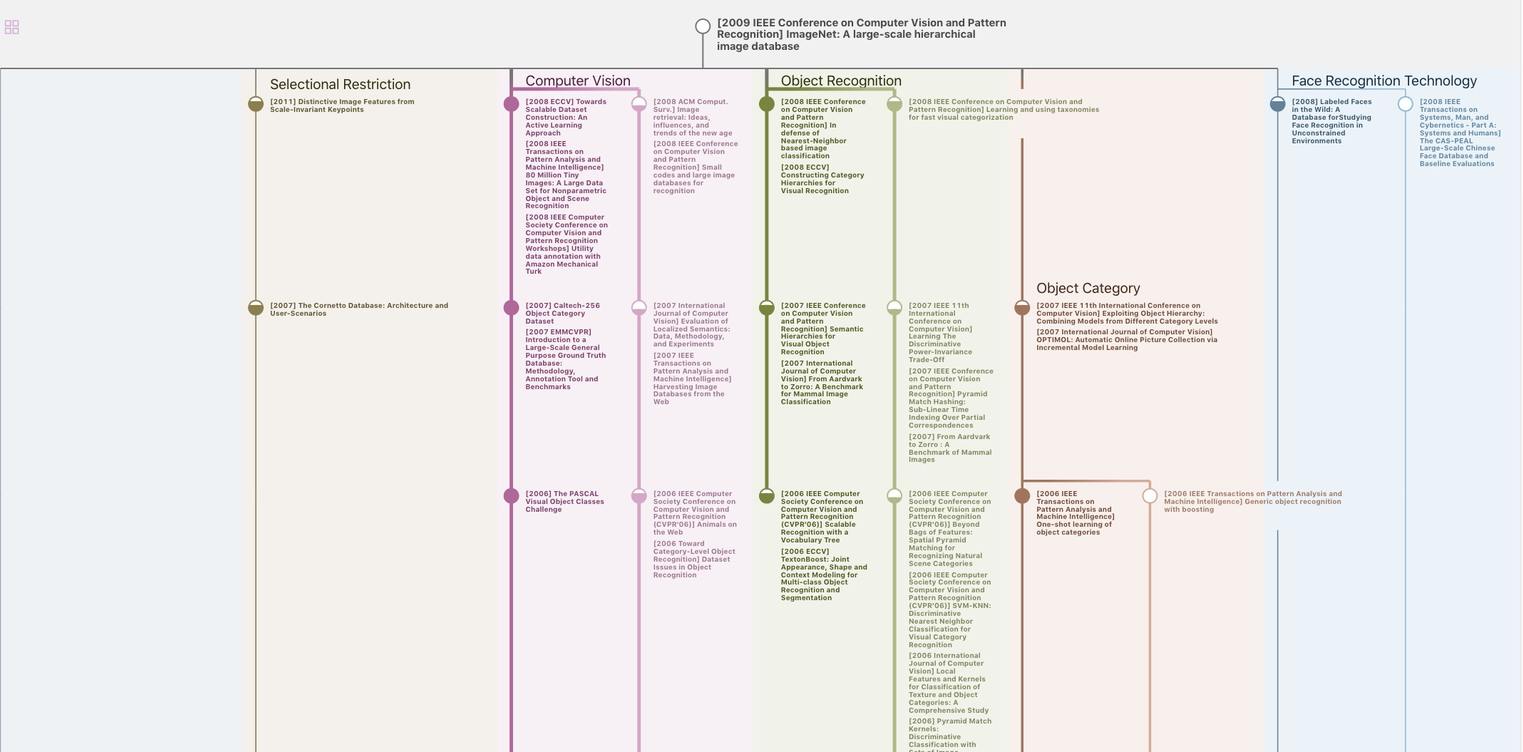
生成溯源树,研究论文发展脉络
Chat Paper
正在生成论文摘要